A Parallel Structured Divide-and-Conquer Algorithm for Symmetric Tridiagonal Eigenvalue Problems
IEEE Transactions on Parallel and Distributed Systems(2021)
摘要
In this article, a parallel structured divide-and-conquer (PSDC) eigensolver is proposed for symmetric tridiagonal matrices based on ScaLAPACK and a parallel structured matrix multiplication algorithm, called PSMMA. Computing the eigenvectors via matrix-matrix multiplications is the most computationally expensive part of the divide-and-conquer algorithm, and one of the matrices involved in such multiplications is a rank-structured Cauchy-like matrix. By exploiting this particular property, PSMMA constructs the local matrices by using generators of Cauchy-like matrices without any communication, and further reduces the computation costs by using a structured low-rank approximation algorithm. Thus, both the communication and computation costs are reduced. Experimental results show that both PSMMA and PSDC are highly scalable and scale to 4096 processes at least. PSDC has better scalability than PHDC that was proposed in [16] and only scaled to 300 processes for the same matrices. Comparing with PDSTEDC in ScaLAPACK, PSDC is always faster and achieves 1.4x-1.6x speedup for some matrices with few deflations. PSDC is also comparable with ELPA, with PSDC being faster than ELPA when using few processes and a little slower when using many processes.
更多查看译文
关键词
PSMMA,PUMMA algorithm,ScaLAPACK,divide-and-conquer,rank-structured matrix,cauchy-like matrix
AI 理解论文
溯源树
样例
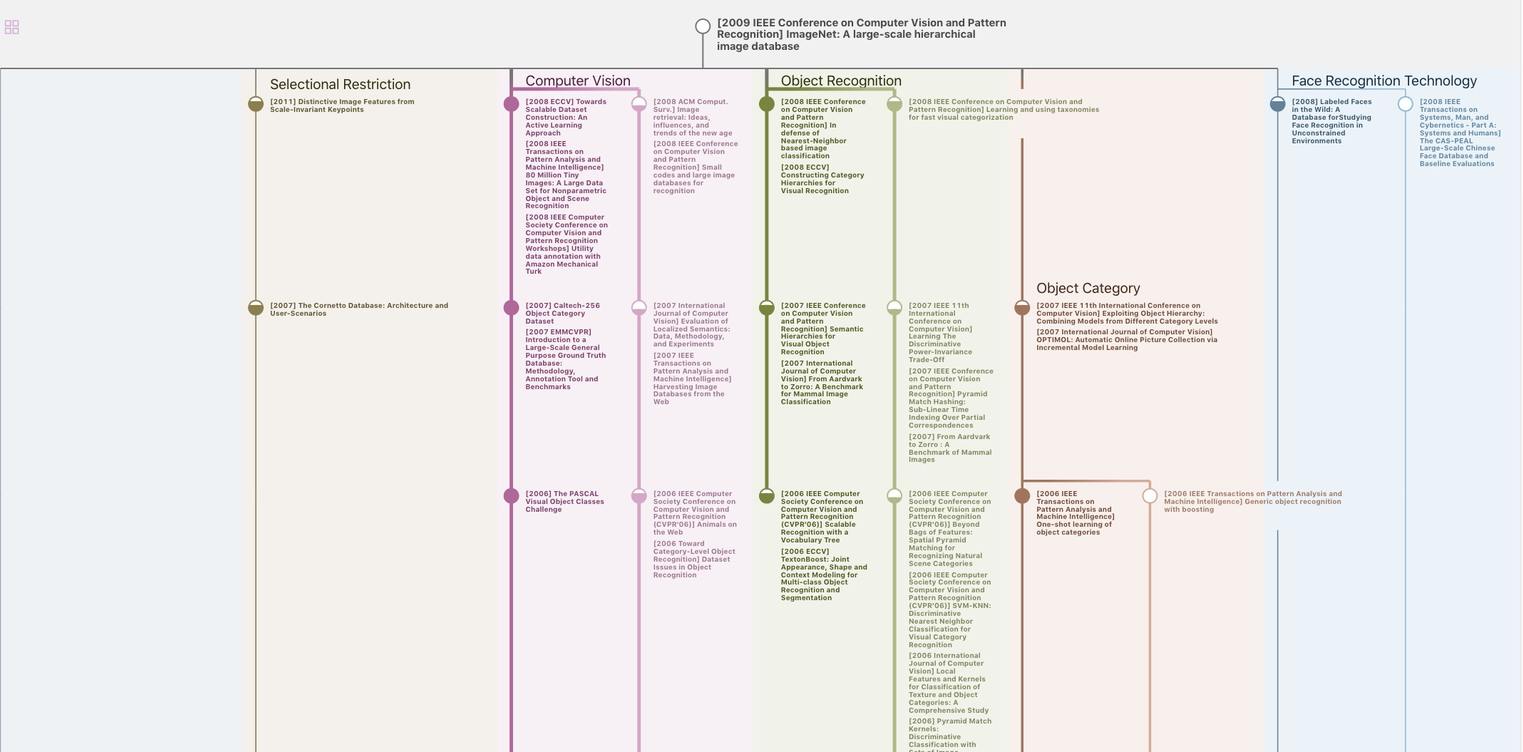
生成溯源树,研究论文发展脉络
Chat Paper
正在生成论文摘要