IntelligentPooling: practical Thompson sampling for mHealth
MACHINE LEARNING(2021)
摘要
In mobile health (mHealth) smart devices deliver behavioral treatments repeatedly over time to a user with the goal of helping the user adopt and maintain healthy behaviors. Reinforcement learning appears ideal for learning how to optimally make these sequential treatment decisions. However, significant challenges must be overcome before reinforcement learning can be effectively deployed in a mobile healthcare setting. In this work we are concerned with the following challenges: (1) individuals who are in the same context can exhibit differential response to treatments (2) only a limited amount of data is available for learning on any one individual, and (3) non-stationary responses to treatment. To address these challenges we generalize Thompson-Sampling bandit algorithms to develop IntelligentPooling . IntelligentPooling learns personalized treatment policies thus addressing challenge one. To address the second challenge, IntelligentPooling updates each user’s degree of personalization while making use of available data on other users to speed up learning. Lastly, IntelligentPooling allows responsivity to vary as a function of a user’s time since beginning treatment, thus addressing challenge three.
更多查看译文
关键词
Thompson sampling, Mobile health, Clinical trial, Physical activity, Non-stationary environment, Mixed effects, Bayesian reward model
AI 理解论文
溯源树
样例
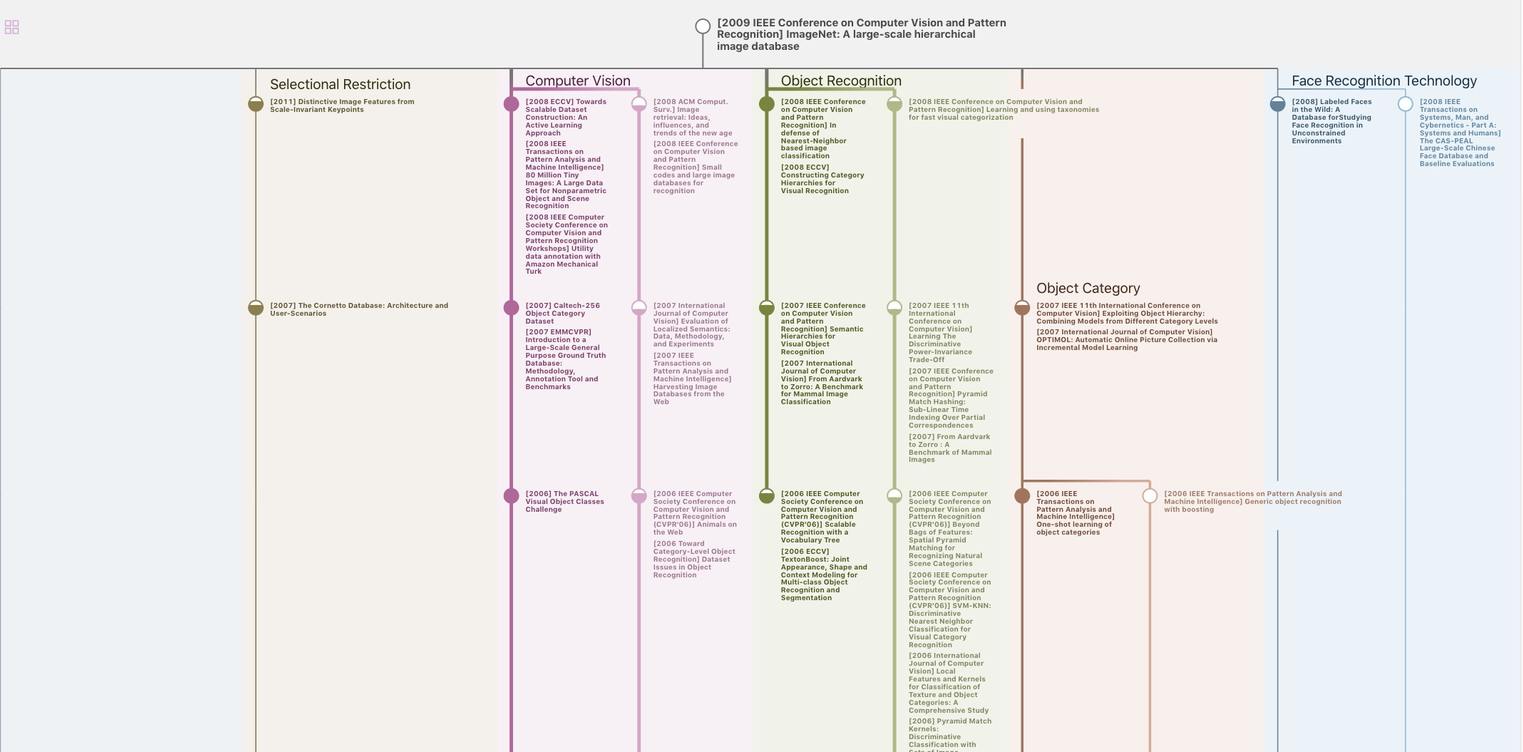
生成溯源树,研究论文发展脉络
Chat Paper
正在生成论文摘要