Bilinear Convolutional Auto-Encoder Based Pilot-Free End-To-End Communication Systems
ICC 2020 - 2020 IEEE INTERNATIONAL CONFERENCE ON COMMUNICATIONS (ICC)(2020)
摘要
Recently, deep learning based end-to-end communication systems have been developed, where both the transmitter and the receiver are represented as deep neural networks (DNN) and an end-to-end loss is optimized directly. In this paper, we address the effects of the more general wireless channels to the end-to-end framework. We formulate this problem as training a deep auto-encoder system with an adversarial convolutional layer and propose a training procedure with mini-batches of input samples and channels. Instead of using pilots to explicitly estimate the unknown channel, the auto-encoder learns to address the channel effects without any pilot information. In particular, the receiver contains two modules, designed for channel information extraction and data recovery, respectively. The features obtained from the channel information extraction module are combined with received signals by a bilinear production and then processed by the data recovery module to reconstruct the original input data. The experimental results show a performance improvement compared with the traditional methods in commonly seen wireless channels, including frequency-selective channels and multi-input multi-output (MIMO) channels.
更多查看译文
关键词
deep learning based end-to-end communication system,MIMO channel,multi-input multi-output channel,frequency-selective channel,adversarial convolutional layer,wireless channel,deep neural networks,data recovery,channel information extraction,deep auto-encoder system,bilinear convolutional auto-encoder based pilot-free end-to-end communication systems
AI 理解论文
溯源树
样例
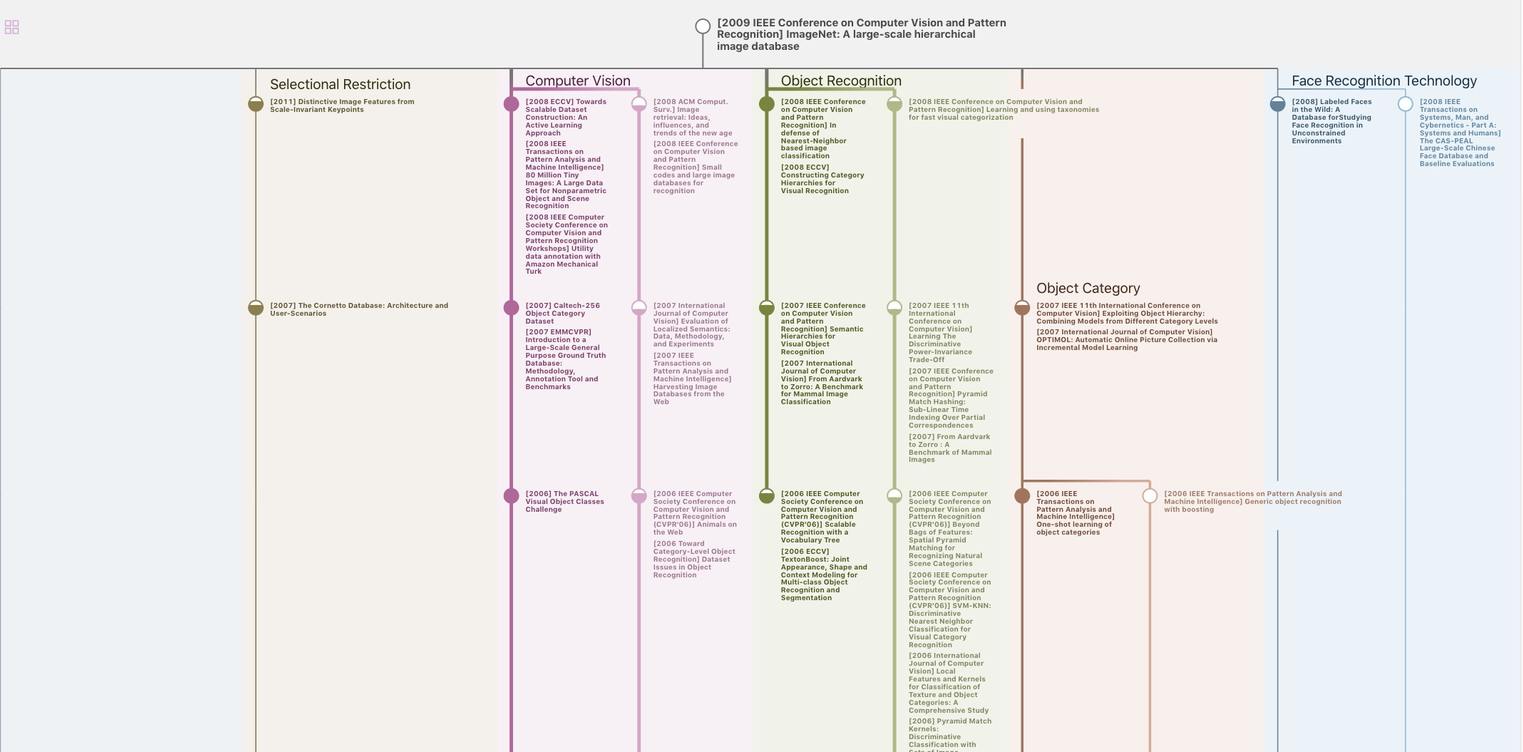
生成溯源树,研究论文发展脉络
Chat Paper
正在生成论文摘要