Accuracy vs. Cost Trade-off for Machine Learning Based QoE Estimation in 5G Networks
ICC 2020 - 2020 IEEE INTERNATIONAL CONFERENCE ON COMMUNICATIONS (ICC)(2020)
摘要
Since their first release, 5G systems have been enhanced with Network Data Analytics Functionalities (NWDAF) as well as with the ability to interact with 3rd parties' Application Functions (AFs). Such capabilities enable a variety of potentials, unimaginable for earlier generation networks, notable examples being 5G built-in Machine Learning (ML) mechanisms for QoE estimation, subject of this paper. In this work, an ML-based mechanism for video streaming QoE estimation in 5G networks is presented and evaluated. The mechanism relies on an ML algorithm embedded in NWDAF, the collection of 5G network KPIs, and the collection of QoE information from video streaming service provider, i.e., the 3rd party AF. The mechanism has been evaluated in terms of QoE estimation accuracy against the cost in terms of required input sources and data for the estimation, and its performance has been compared to alternative methodologies not making use of ML. The evaluation, via simulation activity, clearly highlights the benefits of the proposed mechanism. Based on the derived results, the required input sources are ranked with respect to their importance.
更多查看译文
关键词
HAS, QoE, Machine Learning, 5G
AI 理解论文
溯源树
样例
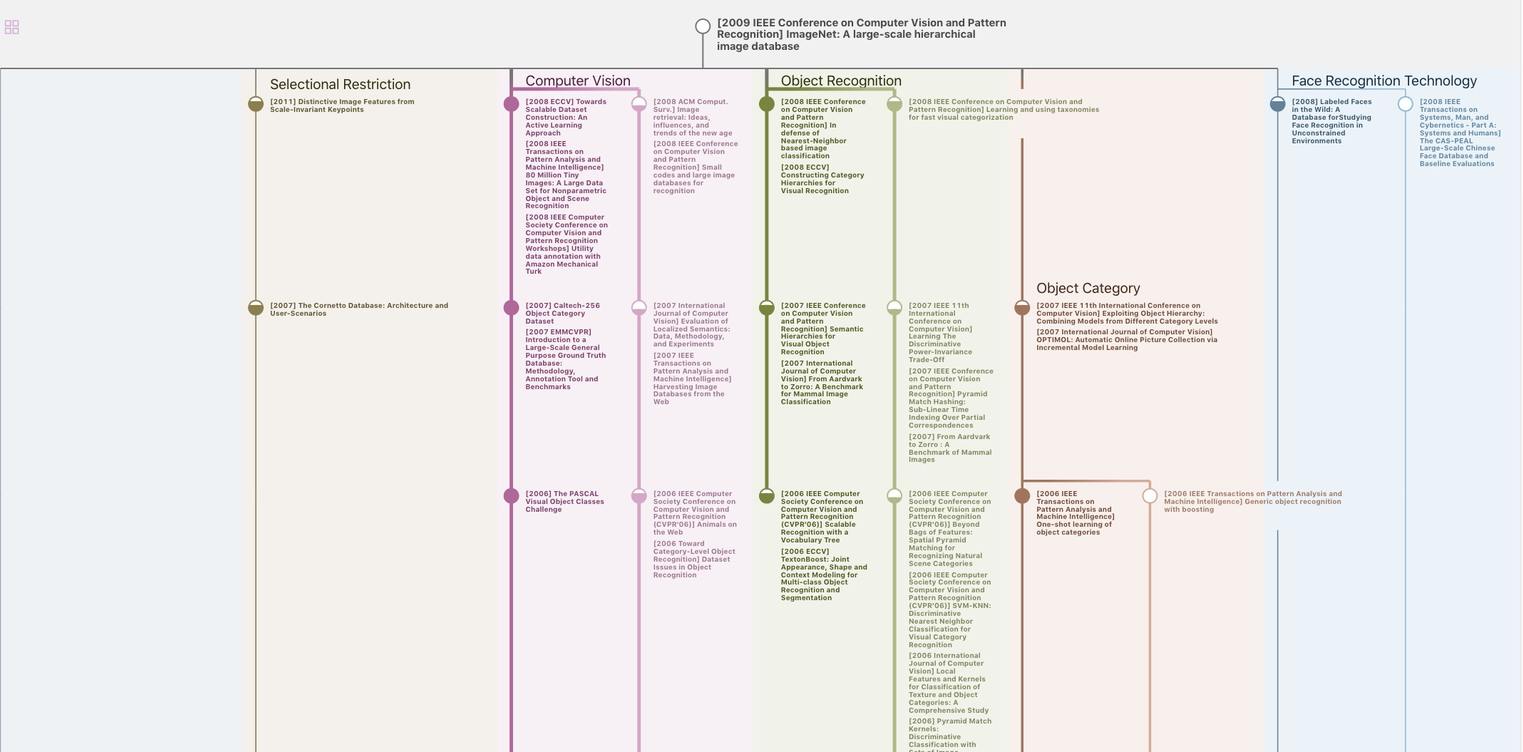
生成溯源树,研究论文发展脉络
Chat Paper
正在生成论文摘要