Unsupervised Mmwave Beamforming Via Autoencoders
ICC 2020 - 2020 IEEE INTERNATIONAL CONFERENCE ON COMMUNICATIONS (ICC)(2020)
摘要
We provide unsupervised machine learning (ML) schemes based on autoencoders for unconstrained beamforming (BF) and hybrid BF in millimeter-waves (mmWaves). An autoencoder is a powerful unsupervised ML model, and it is used to reconstruct the input with a minimal error by finding a low-dimensional representation of the input. In this paper, we present a linear autoencoder for finding the beamformers at the transmitter (Tx) and receiver (Rx), which maximize the achieved rates over the mmWave channel. Since the autoencoder has a close relationship with the singular value decomposition (SVD), we first study autoencoders for unconstrained BF based on SVD. In hybrid BF, beamformers are designed by using finite-precision phase shifters in the radio frequency (RF) domain along with power constraints. Therefore, we propose a hybrid BF algorithm based on autoencoders, which incorporates these constraints. We present our simulation results for both unconstrained BF as well as hybrid BF, and compare their performance with state-of-the-art. By using the stochastic and NYUSIM channel models, we achieve 30-40% and 60-70% gains in rates with the proposed autoencoder based approach compared to the supervised hybrid BF with the stochastic and NYUSIM channel models, respectively.
更多查看译文
关键词
stochastic channel models,RF domain,radio frequency domain,finite-precision phase shifters,SVD,singular value decomposition,unsupervised mmWave beamforming,unsupervised ML model,supervised hybrid BF,NYUSIM channel models,hybrid BF algorithm,unconstrained BF,mmWave channel,beamformers,linear autoencoder,millimeter-waves,unconstrained beamforming,unsupervised machine learning schemes
AI 理解论文
溯源树
样例
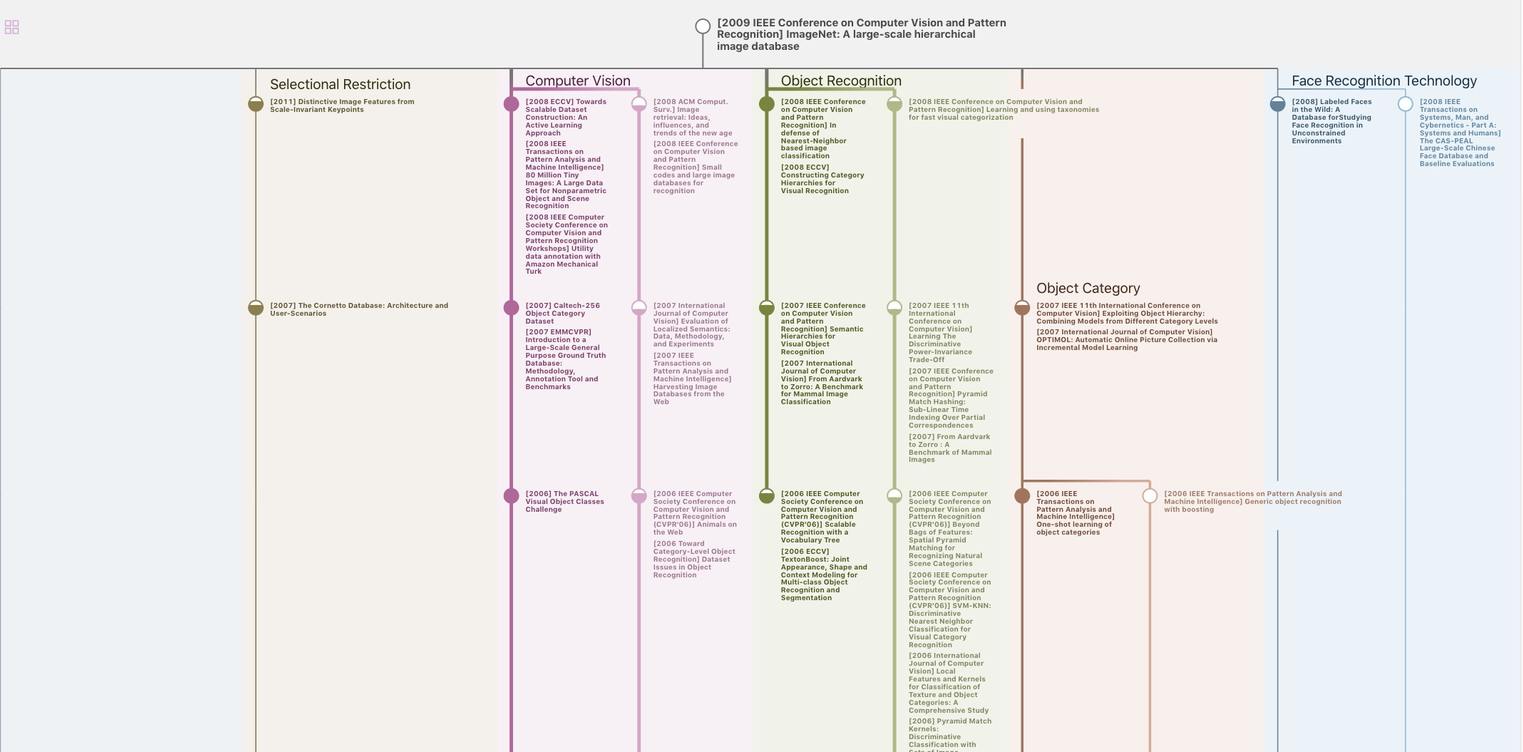
生成溯源树,研究论文发展脉络
Chat Paper
正在生成论文摘要