STATISTICAL GUARANTEES FOR BAYESIAN UNCERTAINTY QUANTIFICATION IN NONLINEAR INVERSE PROBLEMS WITH GAUSSIAN PROCESS PRIORS
ANNALS OF STATISTICS(2021)
摘要
Bayesian inference and uncertainty quantification in a general class of nonlinear inverse regression models is considered. Analytic conditions on the regression model {g(theta) : theta is an element of Theta} and on Gaussian process priors for theta are provided such that semiparametrically efficient inference is possible for a large class of linear functionals of theta. A general Bernstein-von Mises theorem is proved that shows that the (non-Gaussian) posterior distributions are approximated by certain Gaussian measures centred at the posterior mean. As a consequence, posterior-based credible sets are valid and optimal from a frequentist point of view. The theory is illustrated with two applications with PDEs that arise in nonlinear tomography problems: an elliptic inverse problem for a Schrodinger equation, and inversion of non-Abelian X-ray transforms. New analytical techniques are deployed to show that the relevant Fisher information operators are invertible between suitable function spaces.
更多查看译文
关键词
X-ray transforms, Schrodinger equation, credible sets, Bernstein-von Mises theorems
AI 理解论文
溯源树
样例
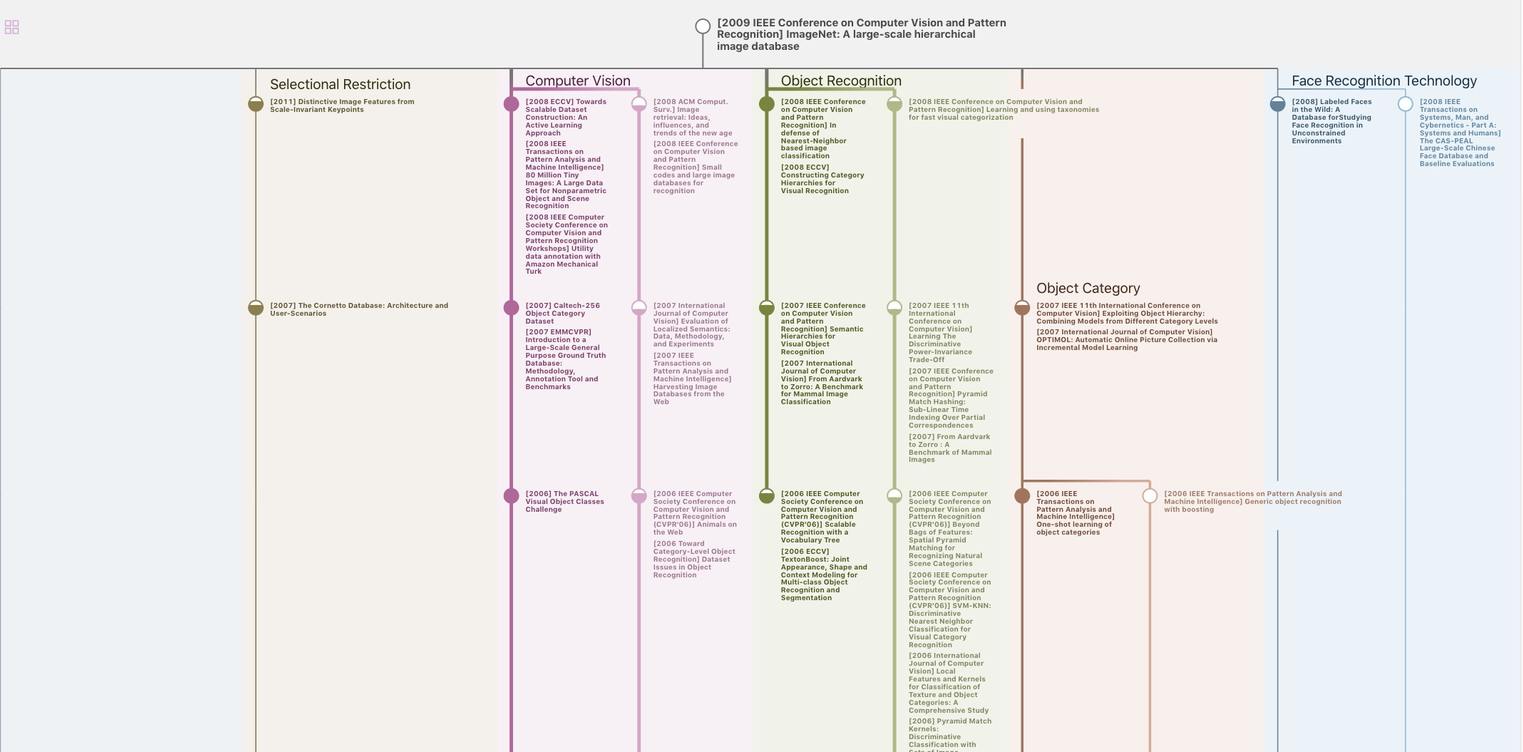
生成溯源树,研究论文发展脉络
Chat Paper
正在生成论文摘要