Robust Retinal Vessel Segmentation from a Data Augmentation Perspective.
OMIA@MICCAI(2021)
摘要
Retinal vessel segmentation is a fundamental step in screening, diagnosis, and treatment of various cardiovascular and ophthalmic diseases. Robustness is one of the most critical requirements for practical utilization, since the test images may be captured using different fundus cameras, or be affected by various pathological changes. We investigate this problem from a data augmentation perspective, with the merits of no additional training data or inference time. In this paper, we propose two new data augmentation modules, namely, channel-wise random Gamma correction and channel-wise random vessel augmentation. Given a training color fundus image, the former applies random gamma correction on each color channel of the entire image, while the latter intentionally enhances or decreases only the fine-grained blood vessel regions using morphological transformations. With the additional training samples generated by applying these two modules sequentially, a model could learn more invariant and discriminating features against both global and local disturbances. Experimental results on both real-world and synthetic datasets demonstrate that our method can improve the performance and robustness of a classic convolutional neural network architecture. Source codes are available https://github.com/PaddlePaddle/Research/tree/master/CV/robust_vessel_segmentation
更多查看译文
关键词
robust retinal vessel segmentation,data augmentation perspective
AI 理解论文
溯源树
样例
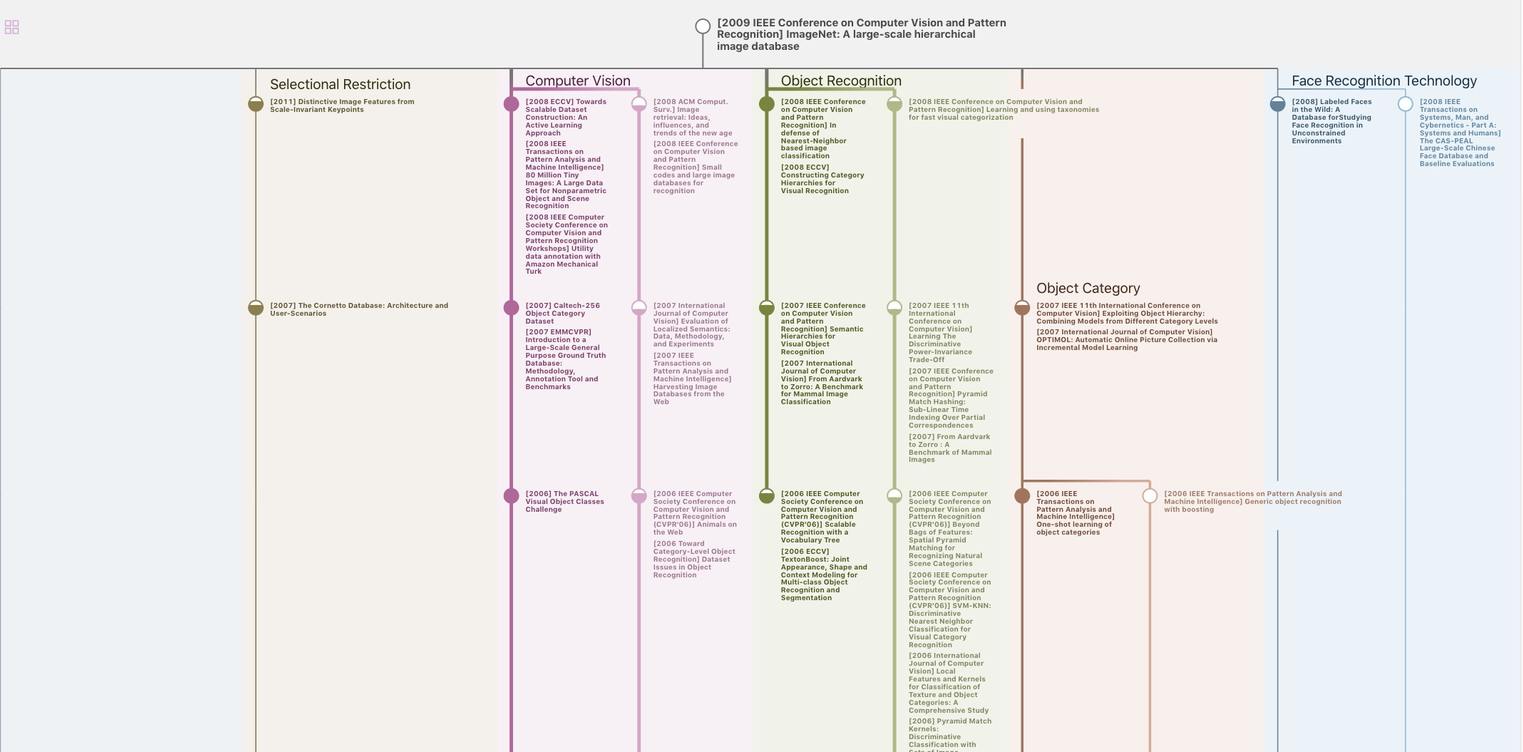
生成溯源树,研究论文发展脉络
Chat Paper
正在生成论文摘要