A Functional Model for Structure Learning and Parameter Estimation in Continuous Time Bayesian Network: An Application in Identifying Patterns of Multiple Chronic Conditions
IEEE ACCESS(2021)
摘要
Bayesian networks are powerful statistical models to study the probabilistic relationships among sets of random variables with significant applications in disease modeling and prediction. Here, we propose a continuous time Bayesian network with conditional dependencies represented as regularized Poisson regressions to model the impact of exogenous variables on the conditional intensities of the network. We also propose an adaptive group regularization method with an intuitive early stopping feature based on Gaussian mixture model clustering for efficient learning of the structure and parameters of the proposed network. Using a dataset of patients with multiple chronic conditions extracted from electronic health records of the Department of Veterans Affairs, we compare the performance of the proposed network with some of the existing methods in the literature for both short-term (one-year ahead) and long-term (multi-year ahead) predictions. The proposed model provides a sparse intuitive representation of the complex functional relationships between multiple chronic conditions. It also provides the capability of analyzing multiple disease trajectories over time, given any combination of preexisting conditions.
更多查看译文
关键词
Random variables, Bayes methods, Predictive models, Inference algorithms, Trajectory, Heuristic algorithms, Approximation algorithms, Continuous time Bayesian network, Poisson regression, adaptive group lasso, Gaussian mixture model, multiple chronic conditions
AI 理解论文
溯源树
样例
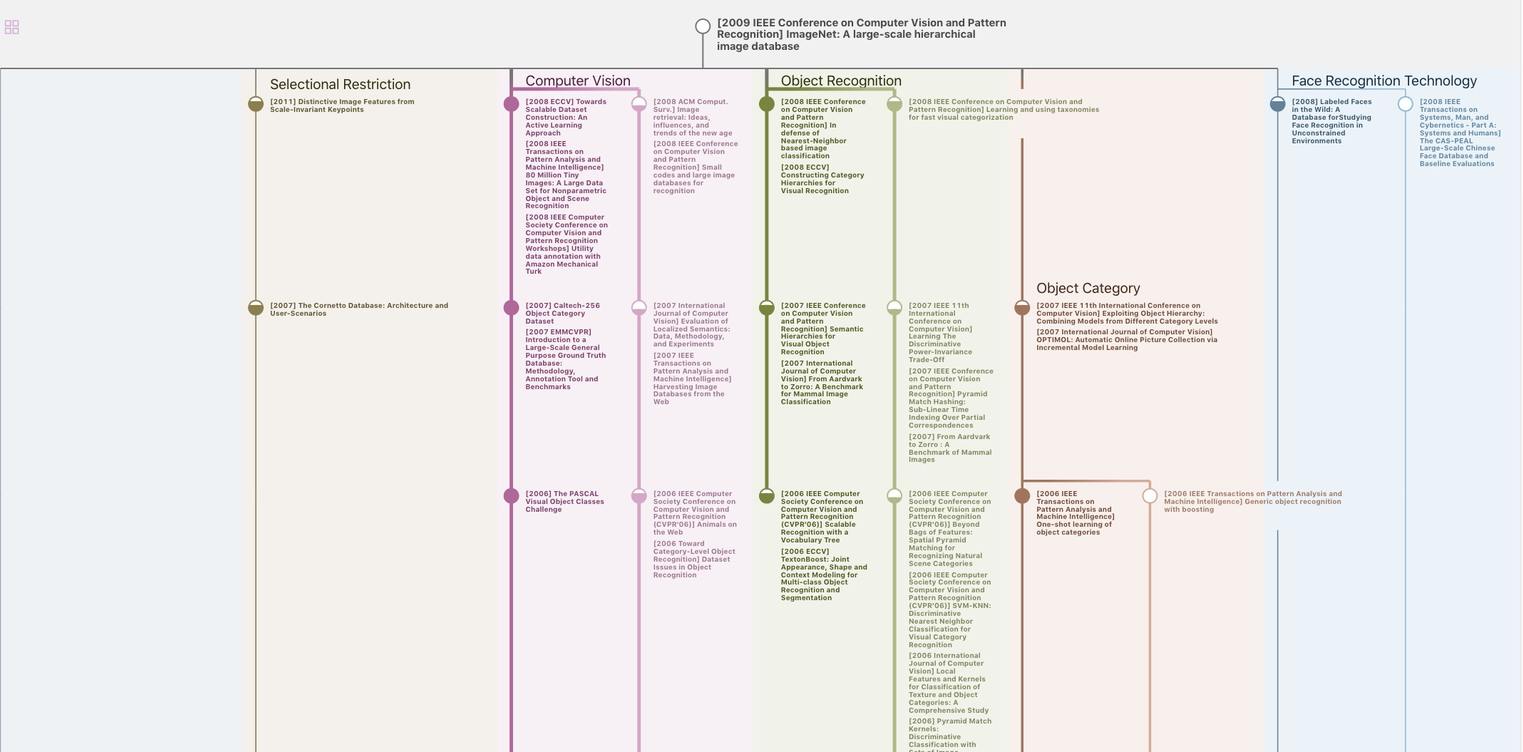
生成溯源树,研究论文发展脉络
Chat Paper
正在生成论文摘要