Assessment of Native Radar Reflectivity and Radar Rainfall Estimates for Discharge Forecasting in Mountain Catchments with a Random Forest Model.
REMOTE SENSING(2020)
摘要
Discharge forecasting is a key component for early warning systems and extremely useful for decision makers. Forecasting models require accurate rainfall estimations of high spatial resolution and other geomorphological characteristics of the catchment, which are rarely available in remote mountain regions such as the Andean highlands. While radar data is available in some mountain areas, the absence of a well distributed rain gauge network makes it hard to obtain accurate rainfall maps. Thus, this study explored a Random Forest model and its ability to leverage native radar data (i.e., reflectivity) by providing a simplified but efficient discharge forecasting model for a representative mountain catchment in the southern Andes of Ecuador. This model was compared with another that used as input derived radar rainfall (i.e., rainfall depth), obtained after the transformation from reflectivity to rainfall rate by using a local Z-R relation and a rain gauge-based bias adjustment. In addition, the influence of a soil moisture proxy was evaluated. Radar and runoff data from April 2015 to June 2017 were used. Results showed that (i) model performance was similar by using either native or derived radar data as inputs (0.66 < NSE < 0.75; 0.72 < KGE < 0.78). Thus, exhaustive pre-processing for obtaining radar rainfall estimates can be avoided for discharge forecasting. (ii) Soil moisture representation as input of the model did not significantly improve model performance (i.e., NSE increased from 0.66 to 0.68). Finally, this native radar data-based model constitutes a promising alternative for discharge forecasting in remote mountain regions where ground monitoring is scarce and hardly available.
更多查看译文
关键词
native radar data,discharge forecasting,machine learning,mountain region,Andes,X-band,radar reflectivity,radar rainfall
AI 理解论文
溯源树
样例
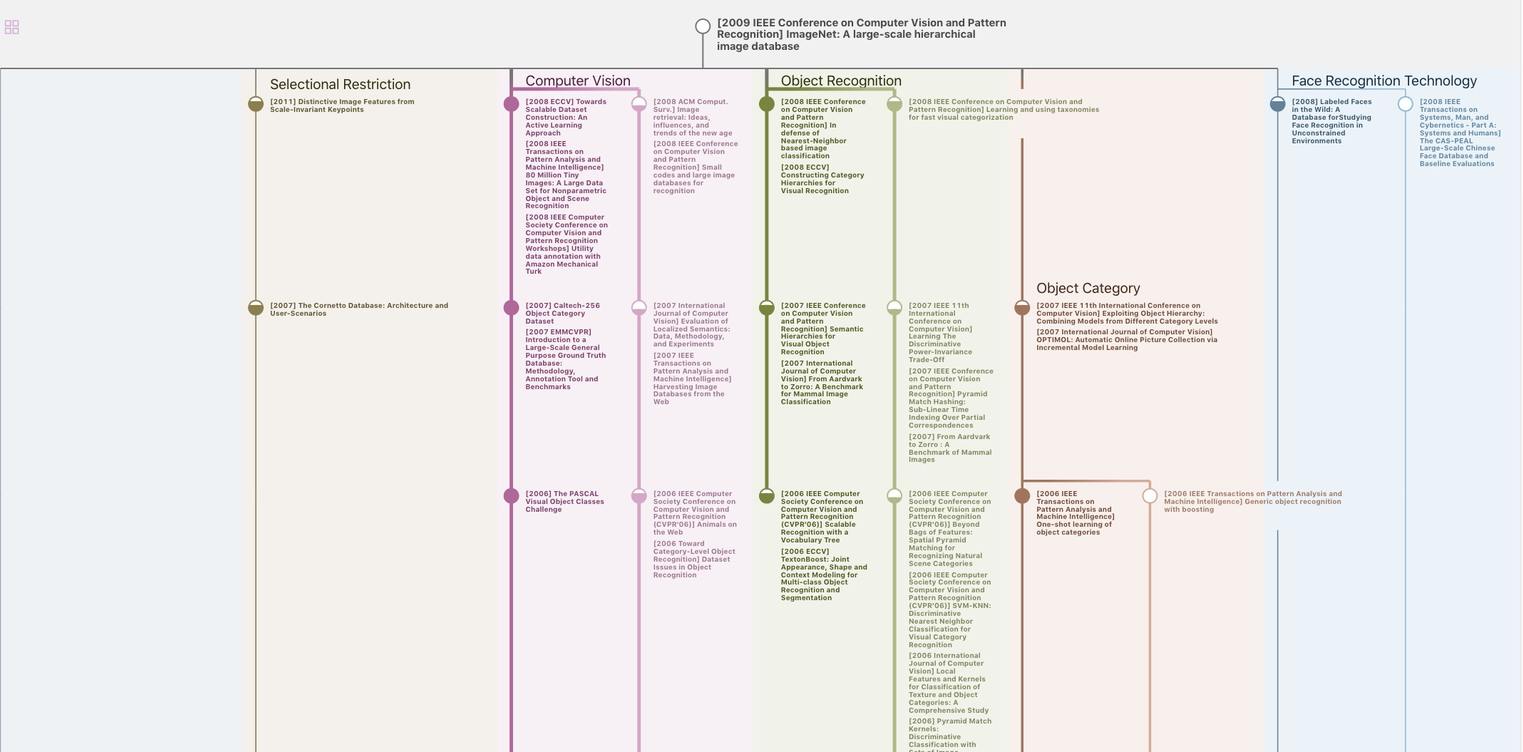
生成溯源树,研究论文发展脉络
Chat Paper
正在生成论文摘要