Reinforcement learning for an intelligent and autonomous production control of complex job-shops under time constraints
Production Engineering(2020)
摘要
Reinforcement learning (RL) offers promising opportunities to handle the ever-increasing complexity in managing modern production systems. We apply a Q -learning algorithm in combination with a process-based discrete-event simulation in order to train a self-learning, intelligent, and autonomous agent for the decision problem of order dispatching in a complex job shop with strict time constraints. For the first time, we combine RL in production control with strict time constraints. The simulation represents the characteristics of complex job shops typically found in semiconductor manufacturing. A real-world use case from a wafer fab is addressed with a developed and implemented framework. The performance of an RL approach and benchmark heuristics are compared. It is shown that RL can be successfully applied to manage order dispatching in a complex environment including time constraints. An RL-agent with a gain function rewarding the selection of the least critical order with respect to time-constraints beats heuristic rules strictly by picking the most critical lot first. Hence, this work demonstrates that a self-learning agent can successfully manage time constraints with the agent performing better than the traditional benchmark, a time-constraint heuristic combining due date deviations and a classical first-in-first-out approach.
更多查看译文
关键词
Complex job shop,Production planning and control,Reinforcement learning,Time constraints
AI 理解论文
溯源树
样例
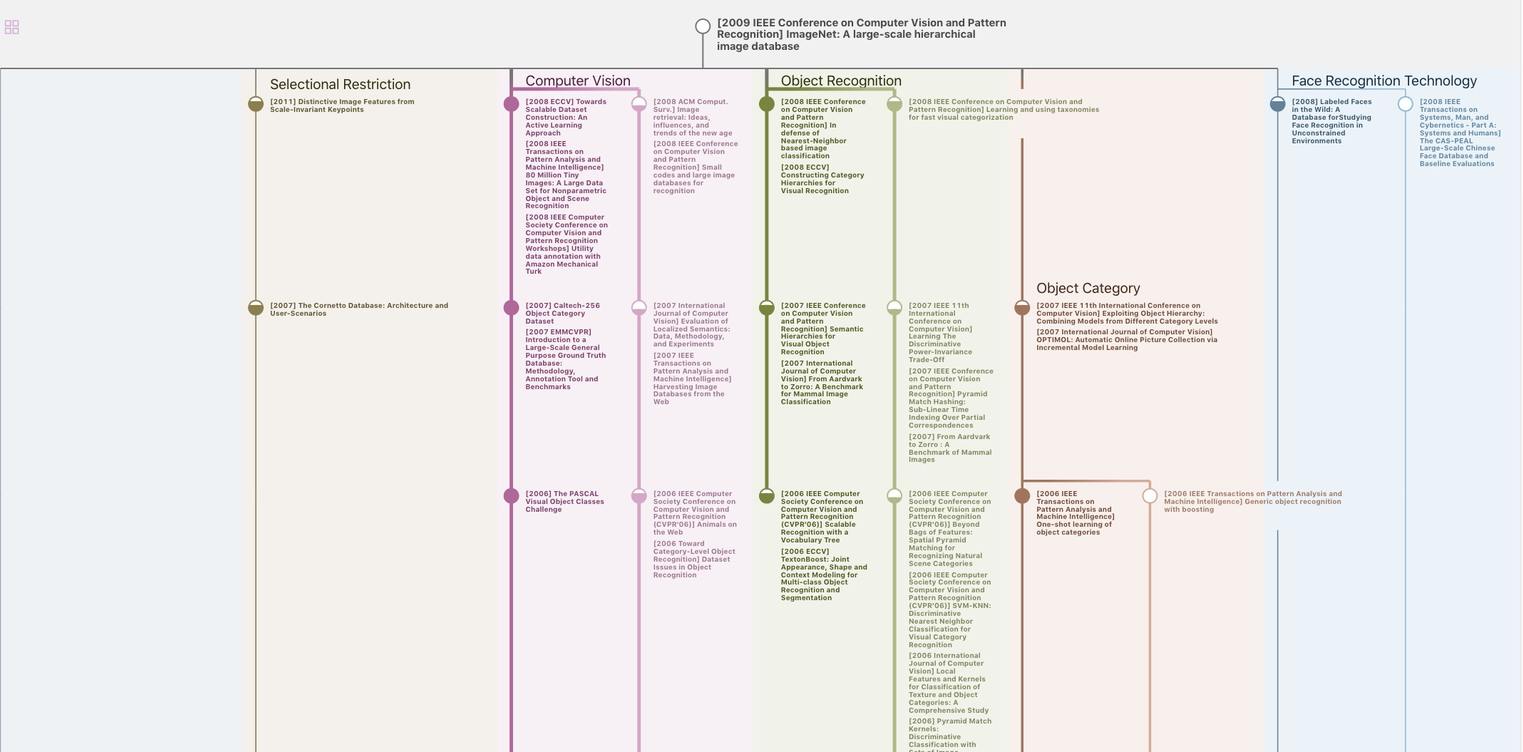
生成溯源树,研究论文发展脉络
Chat Paper
正在生成论文摘要