Prediction of snow water equivalent using artificial neural network and adaptive neuro-fuzzy inference system with two sampling schemes in semi-arid region of Iran
Journal of Mountain Science(2020)
摘要
Direct measurement of snow water equivalent (SWE) in snow-dominated mountainous areas is difficult, thus its prediction is essential for water resources management in such areas. In addition, because of nonlinear trend of snow spatial distribution and the multiple influencing factors concerning the SWE spatial distribution, statistical models are not usually able to present acceptable results. Therefore, applicable methods that are able to predict nonlinear trends are necessary. In this research, to predict SWE, the Sohrevard Watershed located in northwest of Iran was selected as the case study. Database was collected, and the required maps were derived. Snow depth (SD) at 150 points with two sampling patterns including systematic random sampling and Latin hypercube sampling (LHS), and snow density at 18 points were randomly measured, and then SWE was calculated. SWE was predicted using artificial neural network (ANN), adaptive neuro-fuzzy inference system (ANFIS) and regression methods. The results showed that the performance of ANN and ANFIS models with two sampling patterns were observed better than the regression method. Moreover, based on most of the efficiency criteria, the efficiency of ANN, ANFIS and regression methods under LHS pattern were observed higher than the systematic random sampling pattern. However, there were no significant differences between the two methods of ANN and ANFIS in SWE prediction. Data of both two sampling patterns had the highest sensitivity to the elevation. In addition, the LHS and the systematic random sampling patterns had the least sensitivity to the profile curvature and plan curvature, respectively.
更多查看译文
关键词
ANFIS, ANN, Latin hypercube sampling, Systematic random sampling, Snow water equivalent, Snow depth
AI 理解论文
溯源树
样例
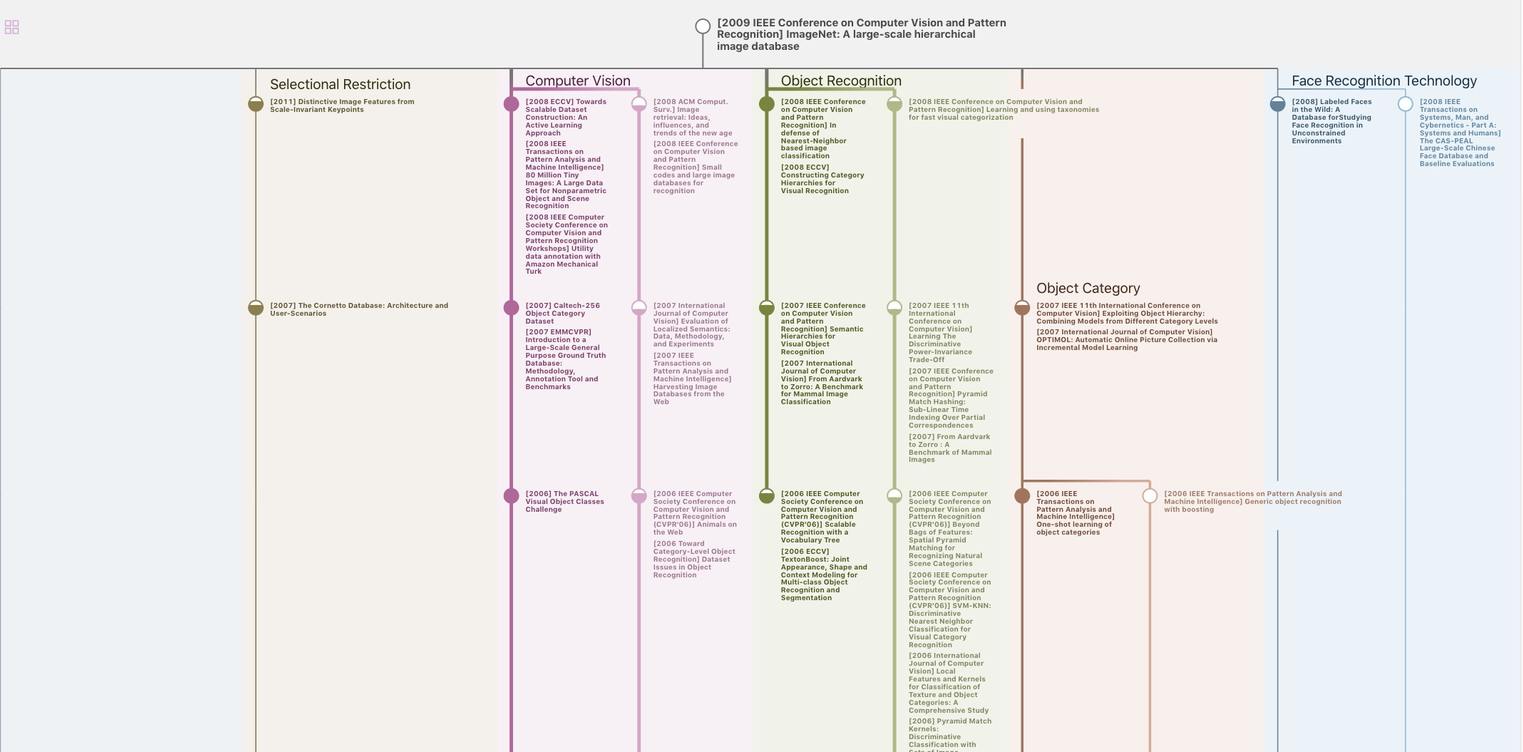
生成溯源树,研究论文发展脉络
Chat Paper
正在生成论文摘要