Understanding the stability of deep control policies for biped locomotion
The Visual Computer(2022)
摘要
Achieving stability and robustness is the primary goal of biped locomotion control. Recently, deep reinforcement learning (DRL) has attracted great attention as a general methodology for constructing biped control policies and demonstrated significant improvements over the previous state-of-the-art control methods. Although deep control policies are more advantageous compared with previous controller design approaches, many questions remain: Are deep control policies as robust as human walking? Does simulated walking involve strategies similar to human walking for maintaining balance? Does a particular gait pattern affect human and simulated walking similarly? What do deep policies learn to achieve improved gait stability? The goal of this study is to address these questions by evaluating the push-recovery stability of deep policies compared with those of human subjects and a previous feedback controller. Furthermore, we conducted experiments to evaluate the effectiveness of variants of DRL algorithms.
更多查看译文
关键词
Biped locomotion, Deep reinforcement learning, Gait analysis, Physically based simulation, Push-recovery stability
AI 理解论文
溯源树
样例
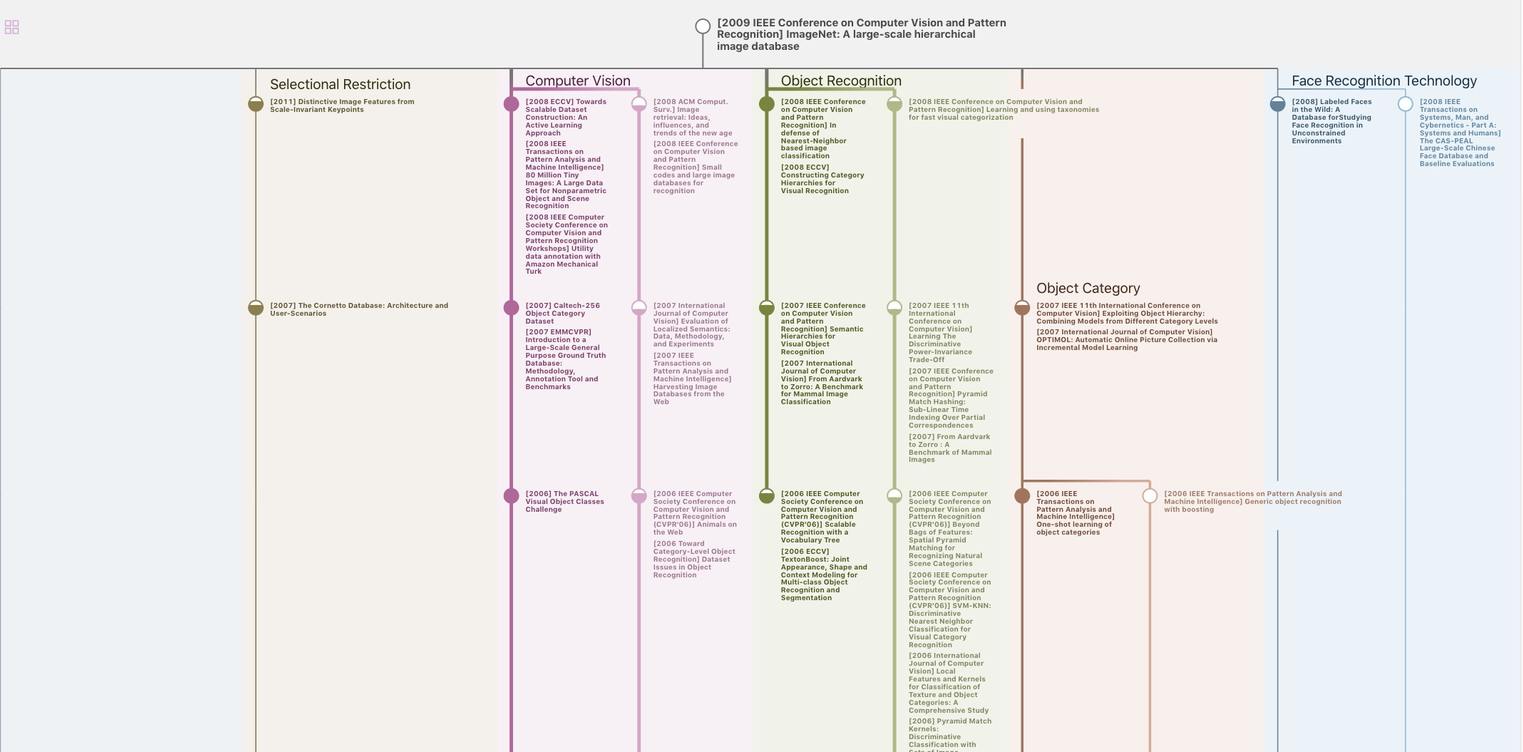
生成溯源树,研究论文发展脉络
Chat Paper
正在生成论文摘要