NeuralQA: A Usable Library for Question Answering (Contextual Query Expansion plus BERT) on Large Datasets
EMNLP(2020)
摘要
Existing tools for Question Answering (QA) have challenges that limit their use in practice. They can be complex to set up or integrate with existing infrastructure, do not offer configurable interactive interfaces, and do not cover the full set of subtasks that frequently comprise the QA pipeline (query expansion, retrieval, reading, and explanation/sensemaking). To help address these issues, we introduce NeuralQA - a usable library for QA on large datasets. NeuralQA integrates well with existing infrastructure (e.g., ElasticSearch instances and reader models trained with the HuggingFace Transformers API) and offers helpful defaults for QA subtasks. It introduces and implements contextual query expansion (CQE) using a masked language model (MLM) as well as relevant snippets (RelSnip) - a method for condensing large documents into smaller passages that can be speedily processed by a document reader model. Finally, it offers a flexible user interface to support workflows for research explorations (e.g., visualization of gradient-based explanations to support qualitative inspection of model behaviour) and large scale search deployment. Code and documentation for NeuralQA is available as open source on Github.
更多查看译文
关键词
question answering,contextual query
AI 理解论文
溯源树
样例
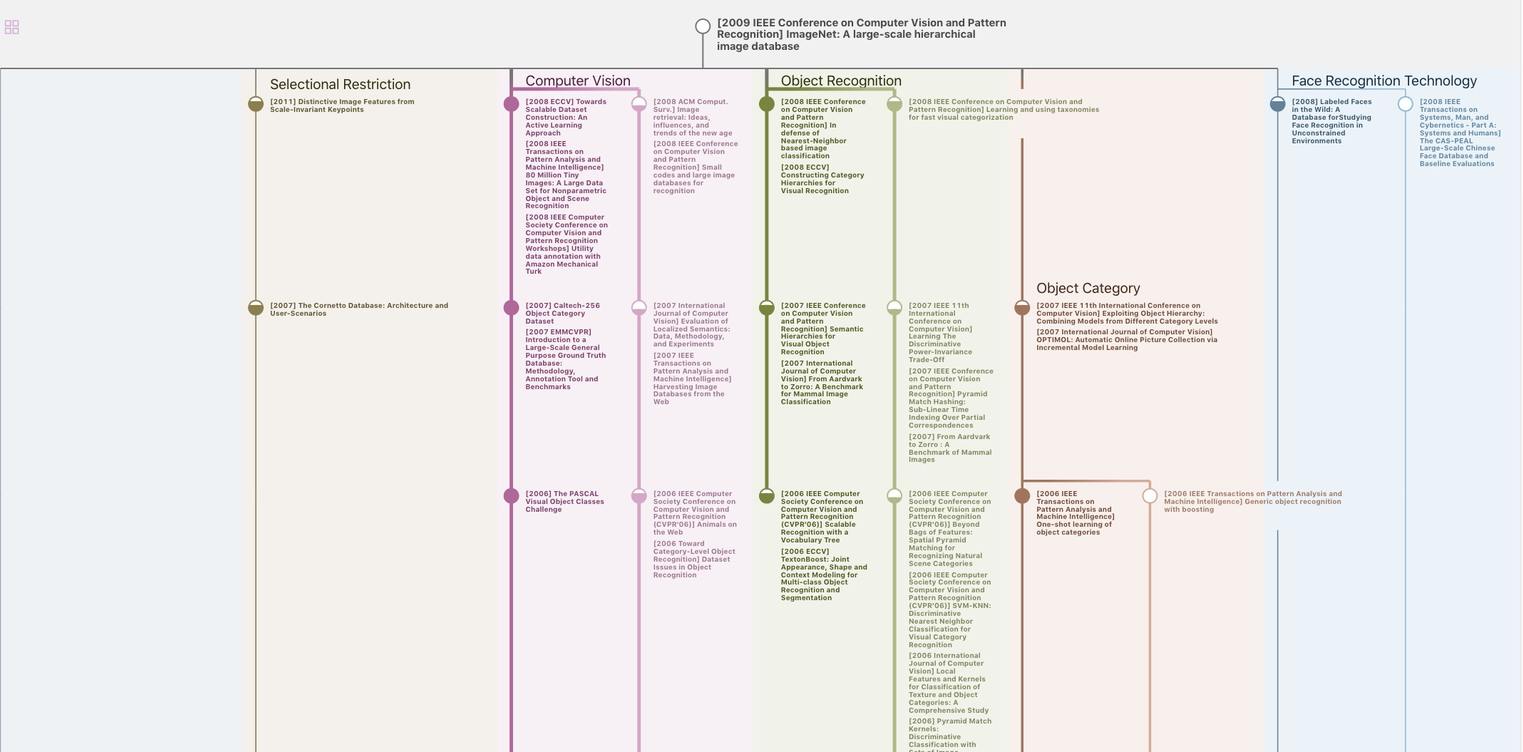
生成溯源树,研究论文发展脉络
Chat Paper
正在生成论文摘要