A Dynamic Classification Approach To Churn Prediction In Banking Industry
AMCIS 2020 PROCEEDINGS(2020)
Abstract
Churn prediction is the process of using transaction data to identify customers who are likely to cease their relationship with a company. To date, most work in churn prediction focuses on sampling strategies and supervised modeling over a short period of time. Few have explored the area of mining customer behavior pattern in longitudinal data. This research developed a dynamic approach to optimizing model specifications by using time-series predictors, multiple time periods, and rare event detection to enable accurate churn prediction. The study used a unique three-year dataset consisting of 32,000 transaction records of a retail bank in Florida, USA. It uses trend modeling to capture the change of customer behavior over time. Results show that data from multiple time periods helped to improve model precision and recall. This dynamic churn prediction approach can be generalized to other fields for which mining long term customer data is necessary.
MoreTranslated text
Key words
Banking, churn prediction, decision support, customer retention, feature engineering, business analytics
AI Read Science
Must-Reading Tree
Example
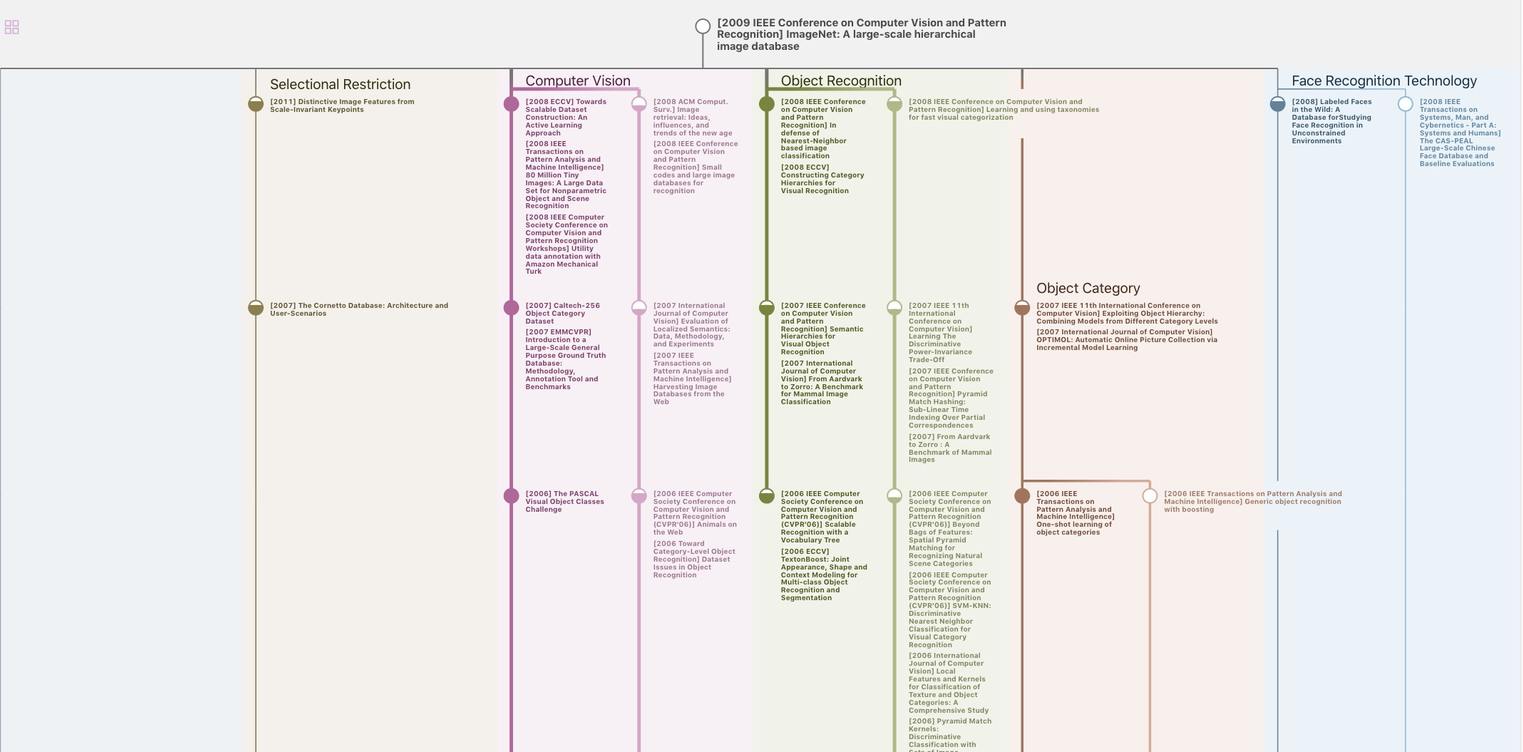
Generate MRT to find the research sequence of this paper
Chat Paper
Summary is being generated by the instructions you defined