Alternating proximal-gradient steps for (stochastic) nonconvex-concave minimax problems
arxiv(2023)
摘要
Minimax problems of the form min(x) max(y) Psi(x, y) have attracted increased interest largely due to advances in machine learning, in particular generative adversarial networks and adversarial learning. These are typically trained using variants of stochastic gradient descent for the two players. Although convex-concave problems are well understood with many efficient solution methods to choose from, theoretical guarantees outside of this setting are sometimes lacking even for the simplest algorithms. In particular, this is the case for alternating gradient descent ascent, where the two agents take turns updating their strategies. To partially close this gap in the literature we prove a novel global convergence rate for the stochastic version of this method for finding a critical point of psi (center dot) := max(y) Psi (center dot, y) in a setting which is not convex-concave.
更多查看译文
关键词
minimax,saddle point,nonconvex-concave,complexity,prox-gradient method,stochastic gradient descent
AI 理解论文
溯源树
样例
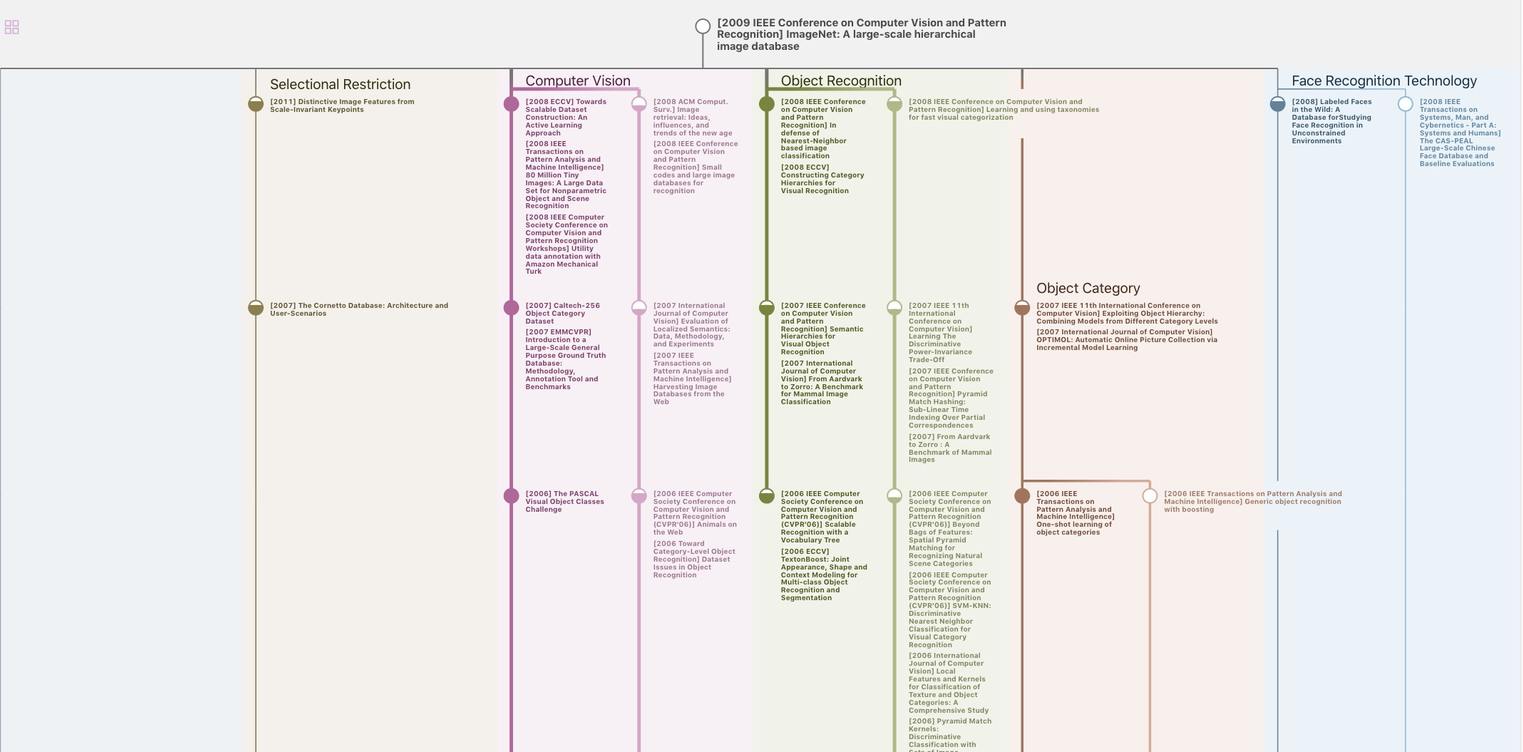
生成溯源树,研究论文发展脉络
Chat Paper
正在生成论文摘要