Supervised Learning in Temporally-Coded Spiking Neural Networks with Approximate Backpropagation
CoRR(2020)
摘要
In this work we propose a new supervised learning method for temporally-encoded multilayer spiking networks to perform classification. The method employs a reinforcement signal that mimics backpropagation but is far less computationally intensive. The weight update calculation at each layer requires only local data apart from this signal. We also employ a rule capable of producing specific output spike trains; by setting the target spike time equal to the actual spike time with a slight negative offset for key high-value neurons the actual spike time becomes as early as possible. In simulated MNIST handwritten digit classification, two-layer networks trained with this rule matched the performance of a comparable backpropagation based non-spiking network.
更多查看译文
AI 理解论文
溯源树
样例
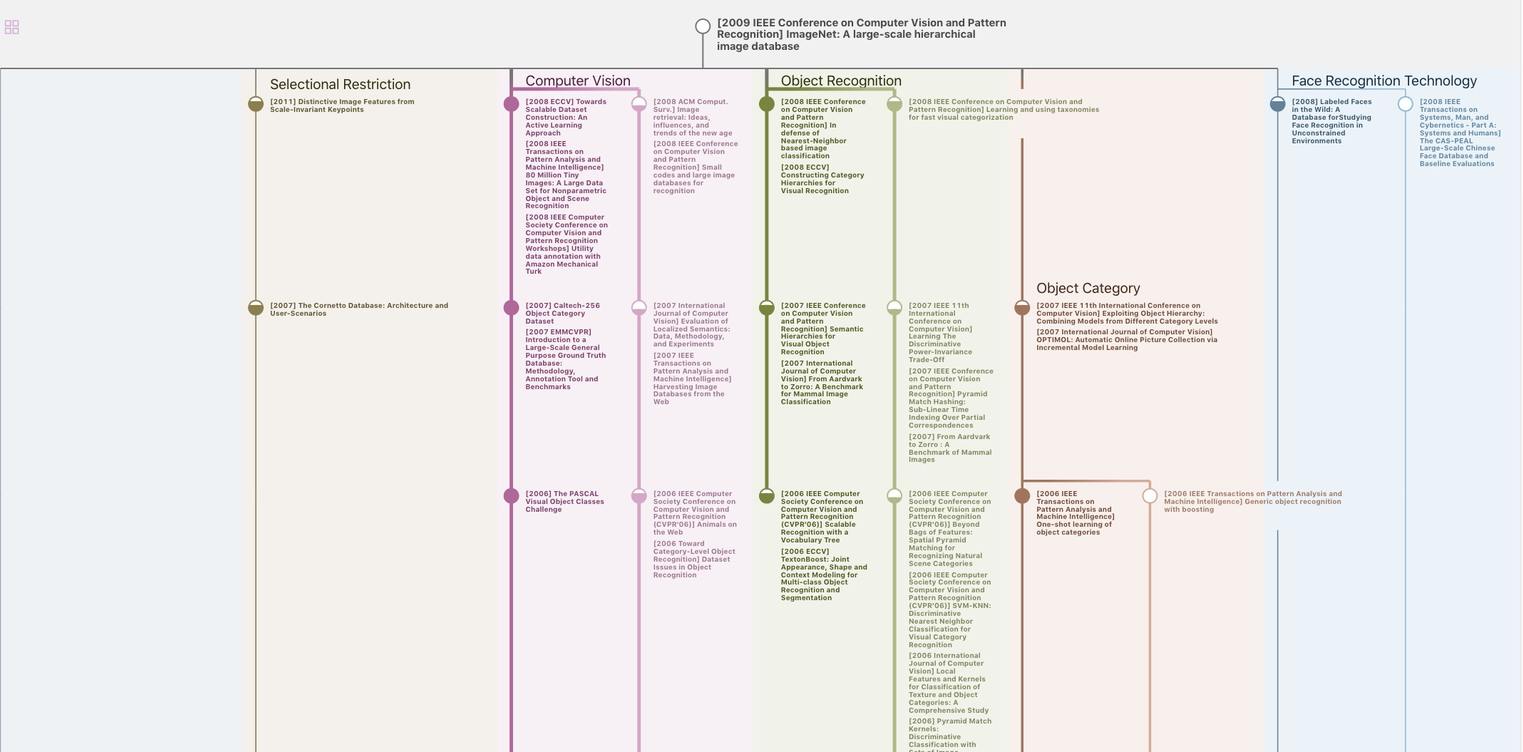
生成溯源树,研究论文发展脉络
Chat Paper
正在生成论文摘要