Economical ensembles with hypernetworks
arxiv(2020)
摘要
Averaging the predictions of many independently trained neural networks is a simple and effective way of improving generalization in deep learning. However, this strategy rapidly becomes costly, as the number of trainable parameters grows linearly with the size of the ensemble. Here, we propose a new method to learn economical ensembles, where the number of trainable parameters and iterations over the data is comparable to that of a single model. Our neural networks are parameterized by hypernetworks, which learn to embed weights in low-dimensional spaces. In a late training phase, we generate an ensemble by randomly initializing an additional number of weight embeddings in the vicinity of each other. We then exploit the inherent randomness in stochastic gradient descent to induce ensemble diversity. Experiments with wide residual networks on the CIFAR and Fashion-MNIST datasets show that our algorithm yields models that are more accurate and less overconfident on unseen data, while learning as efficiently as a single network.
更多查看译文
关键词
economical ensembles,hypernetworks
AI 理解论文
溯源树
样例
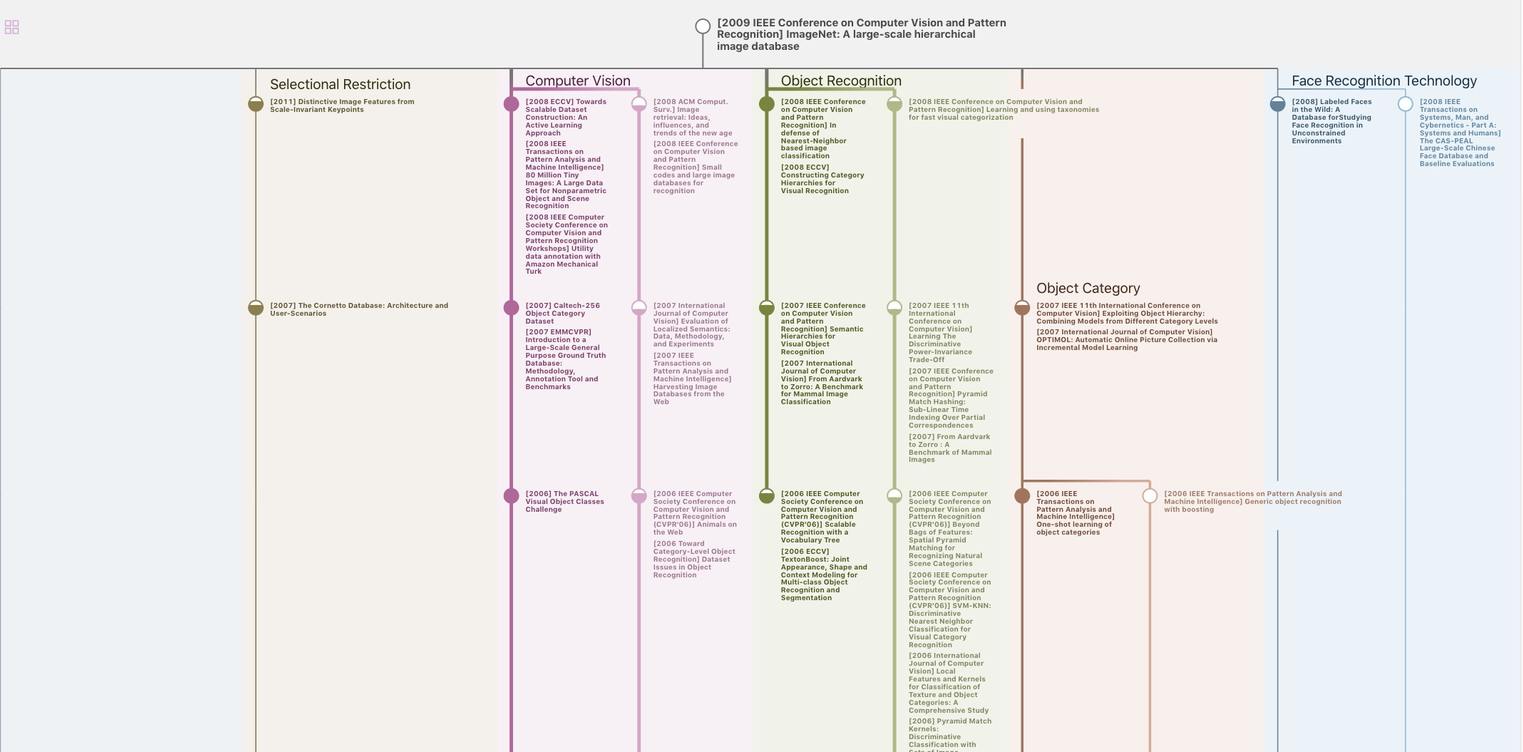
生成溯源树,研究论文发展脉络
Chat Paper
正在生成论文摘要