Efficiency Of Local Learning Rules In Threshold-Linear Associative Networks
PHYSICAL REVIEW LETTERS(2021)
摘要
We derive the Gardner storage capacity for associative networks of threshold linear units, and show that with Hebbian learning they can operate closer to such Gardner bound than binary networks, and even surpass it. This is largely achieved through a sparsification of the retrieved patterns, which we analyze for theoretical and empirical distributions of activity. As reaching the optimal capacity via nonlocal learning rules like back propagation requires slow and neurally implausible training procedures, our results indicate that one-shot self-organized Hebbian learning can be just as efficient.
更多查看译文
关键词
local learning rules,networks,threshold-linear
AI 理解论文
溯源树
样例
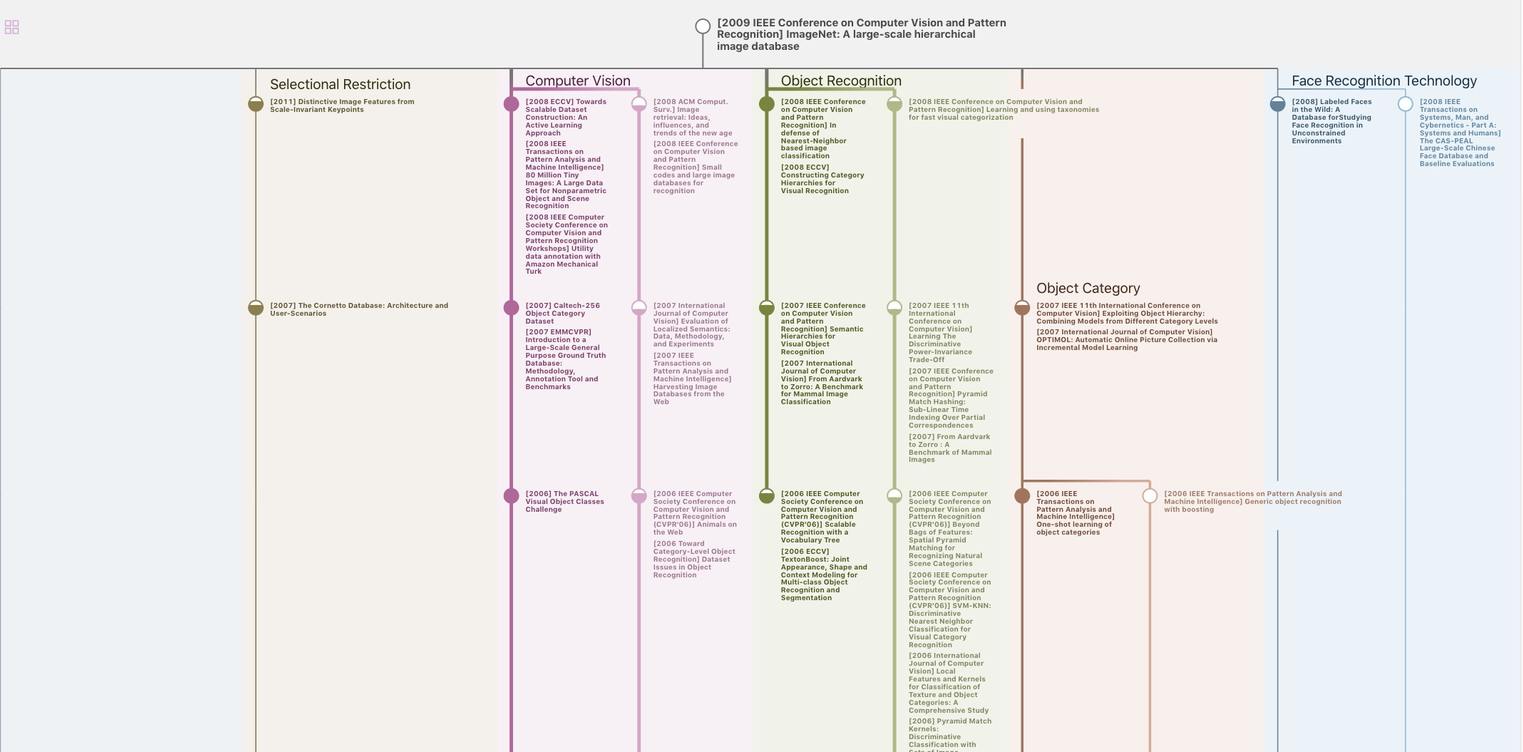
生成溯源树,研究论文发展脉络
Chat Paper
正在生成论文摘要