Adaptive Energy Management for Real Driving Conditions via Transfer Reinforcement Learning
CoRR(2020)
摘要
This article proposes a transfer reinforcement learning (RL) based adaptive energy managing approach for a hybrid electric vehicle (HEV) with parallel topology. This approach is bi-level. The up-level characterizes how to transform the Q-value tables in the RL framework via driving cycle transformation (DCT). Especially, transition probability matrices (TPMs) of power request are computed for different cycles, and induced matrix norm (IMN) is employed as a critical criterion to identify the transformation differences and to determine the alteration of the control strategy. The lower-level determines how to set the corresponding control strategies with the transformed Q-value tables and TPMs by using model-free reinforcement learning (RL) algorithm. Numerical tests illustrate that the transferred performance can be tuned by IMN value and the transfer RL controller could receive a higher fuel economy. The comparison demonstrates that the proposed strategy exceeds the conventional RL approach in both calculation speed and control performance.
更多查看译文
关键词
transfer reinforcement learning,real driving conditions
AI 理解论文
溯源树
样例
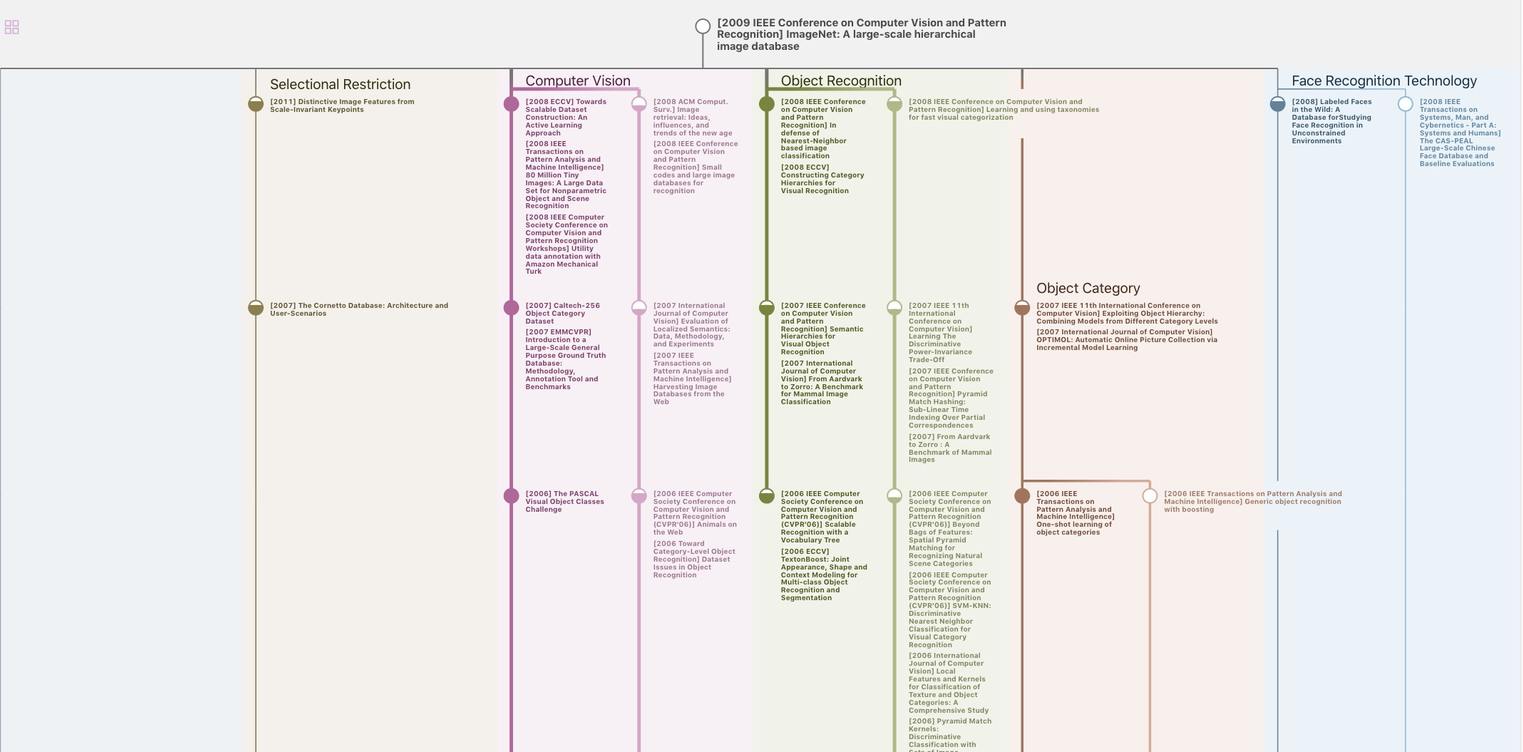
生成溯源树,研究论文发展脉络
Chat Paper
正在生成论文摘要