Off-Policy Multi-Agent Decomposed Policy Gradients
arxiv(2020)
摘要
Recently, multi-agent policy gradient (MAPG) methods witness vigorous progress. However, there is a discrepancy between the performance of MAPG methods and state-of-the-art multi-agent value-based approaches. In this paper, we investigate the causes that hinder the performance of MAPG algorithms and present a multi-agent decomposed policy gradient method (DOP). This method introduces the idea of value function decomposition into the multi-agent actor-critic framework. Based on this idea, DOP supports efficient off-policy learning and addresses the issue of centralized-decentralized mismatch and credit assignment in both discrete and continuous action spaces. We formally show that DOP critics have sufficient representational capability to guarantee convergence. In addition, empirical evaluations on the StarCraft II micromanagement benchmark and multi-agent particle environments demonstrate that our method significantly outperforms state-of-the-art value-based and policy-based multi-agent reinforcement learning algorithms. Demonstrative videos are available at https://sites.google.com/view/dop-mapg.
更多查看译文
关键词
off-policy,multi-agent
AI 理解论文
溯源树
样例
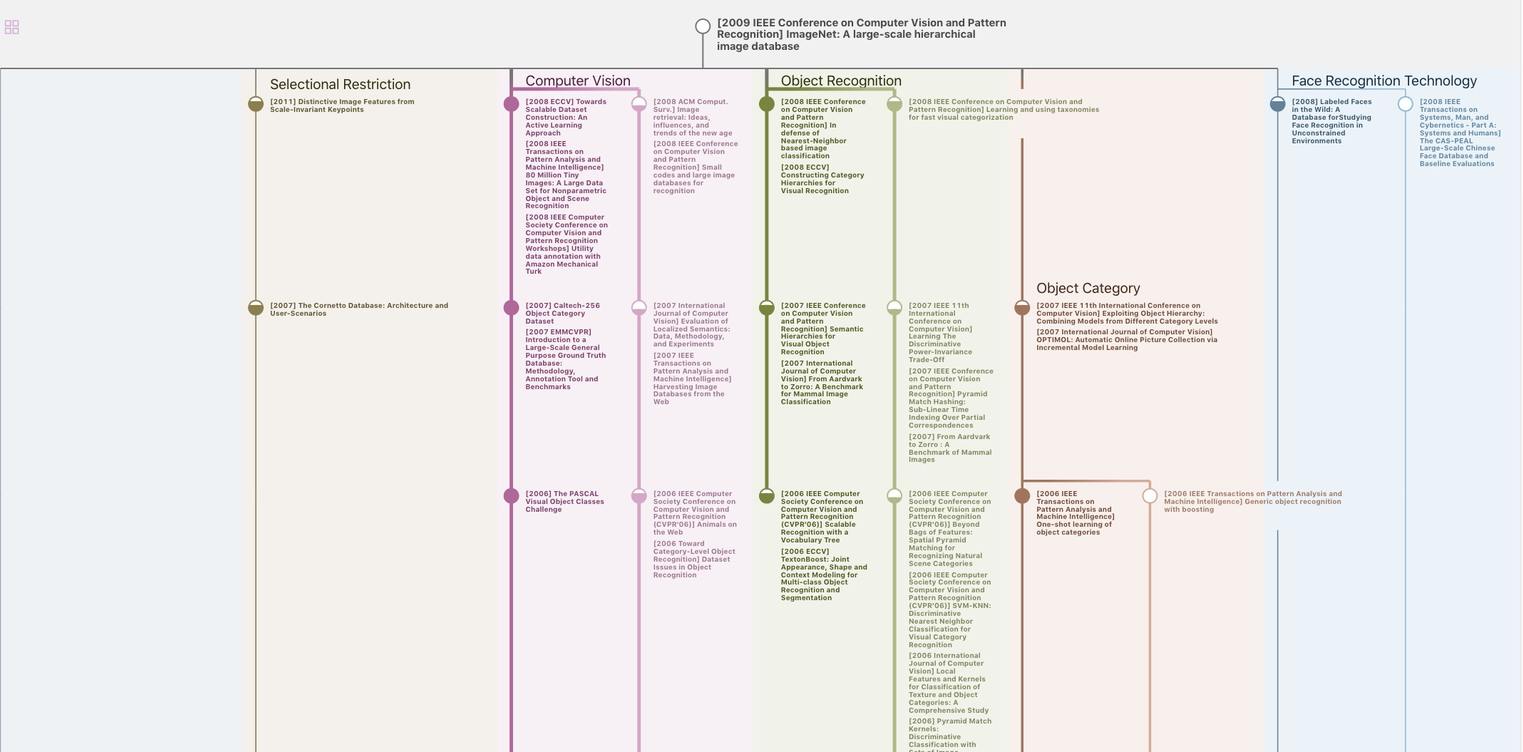
生成溯源树,研究论文发展脉络
Chat Paper
正在生成论文摘要