Knowledge-Based Topic Model for Multi-Modal Social Event Analysis
IEEE transactions on multimedia(2020)
摘要
With the accumulation of data on the Internet and progress in representation learning techniques, knowledge priors learned from a large-scale knowledge base has been increasingly used in probabilistic topic models. However, it is challenging to learn interpretable topics and a discriminative event representation based on multi-modal information. To address these issues, we propose a knowledge priors- and max-margin-based topic model for multi-modal social event analysis, called the KGE-MMSLDA, in which feature representation and knowledge priors are jointly learned. Our model has three main advantages over current methods: (1) It integrates additional knowledge from external knowledge base into a unified topic model in which the max-margin classifier, and multi-modal information are exploited to increase the number of event descriptions obtained. (2) We mined knowledge priors from over 74,000 web documents. Multi-modal data with these knowledge priors are then incorporated into the topic model to increase the number of coherent topics learned. (3) A large-scale multi-modal dataset (containing 10 events, where each event contained approximately 7,000 Flickr pages) was collected and has been released publicly for event topic mining and classification research. In comparative experiments, the proposed method outperformed state-of-the-art models on topic coherence, and obtained a classification accuracy of 85.1%.
更多查看译文
关键词
Analytical models,Knowledge based systems,Social networking (online),Data mining,Data models,Internet,Knowledge engineering,Knowledge embedding,multi-modal,topic coherence,event classification
AI 理解论文
溯源树
样例
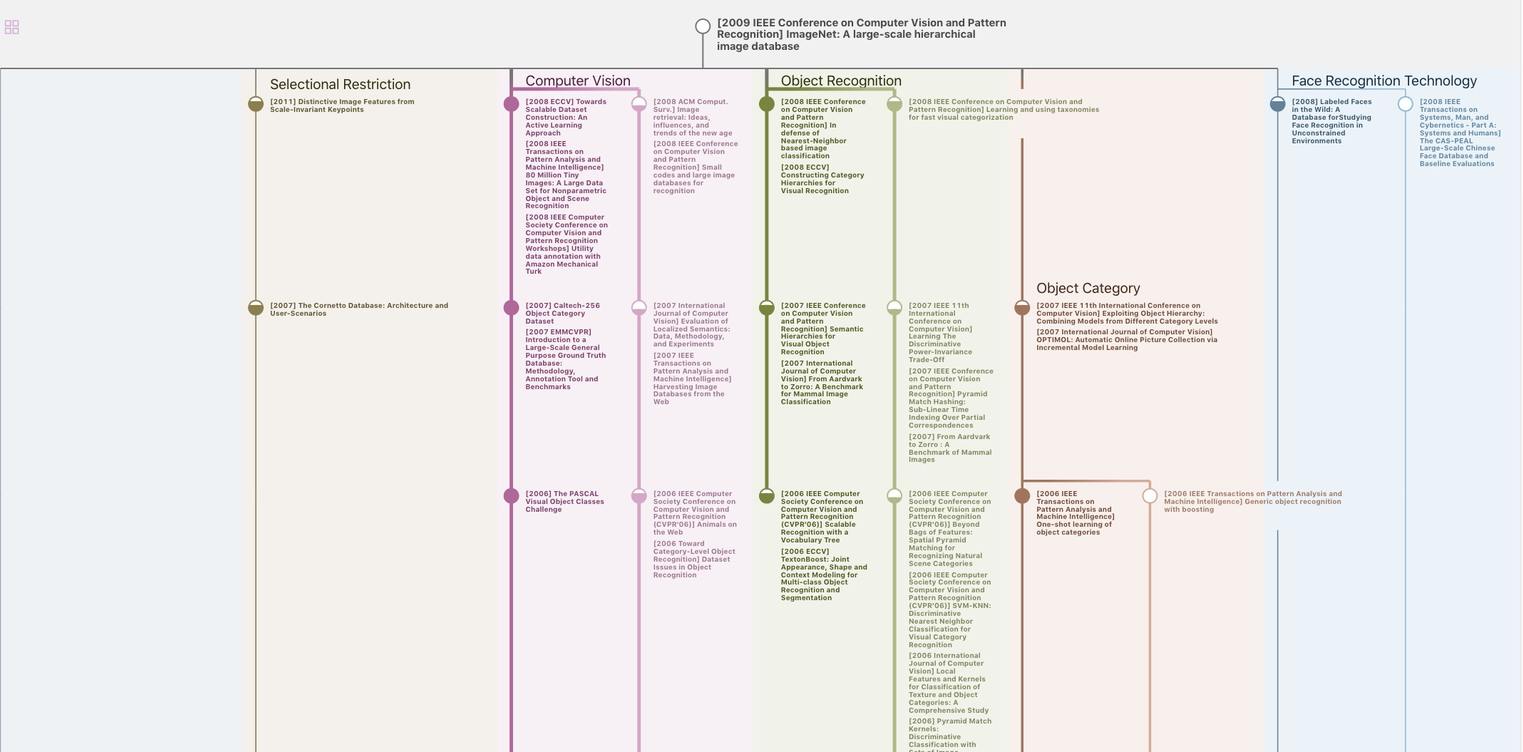
生成溯源树,研究论文发展脉络
Chat Paper
正在生成论文摘要