Part of Speech Production in Patients With Primary Progressive Aphasia: An Analysis Based on Natural Language Processing.
American journal of speech-language pathology(2020)
摘要
Background Primary progressive aphasia (PPA) is a neurodegenerative disorder characterized by a progressive decline of language functions. Its symptoms are grouped into three PPA variants: nonfluent PPA, logopenic PPA, and semantic PPA. Grammatical deficiencies differ depending on the PPA variant. Aims This study aims to determine the differences between PPA variants with respect to part of speech (POS) production and to identify morphological markers that classify PPA variants using machine learning. By fulfilling these aims, the overarching goal is to provide objective measures that can facilitate clinical diagnosis, evaluation, and prognosis. Method and Procedure Connected speech productions from PPA patients produced in a picture description task were transcribed, and the POS class of each word was estimated using natural language processing, namely, POS tagging. We then implemented a twofold analysis: (a) linear regression to determine how patients with nonfluent PPA, semantic PPA, and logopenic PPA variants differ in their POS productions and (b) a supervised classification analysis based on POS using machine learning models (i.e., random forests, decision trees, and support vector machines) to subtype PPA variants and generate feature importance (FI). Outcome and Results Using an automated analysis of a short picture description task, this study showed that content versus function words can distinguish patients with nonfluent PPA, semantic PPA, and logopenic PPA variants. Verbs were less important as distinguishing features of patients with different PPA variants than earlier thought. Finally, the study showed that among the most important distinguishing features of PPA variants were elaborative speech elements, such as adjectives and adverbs.
更多查看译文
AI 理解论文
溯源树
样例
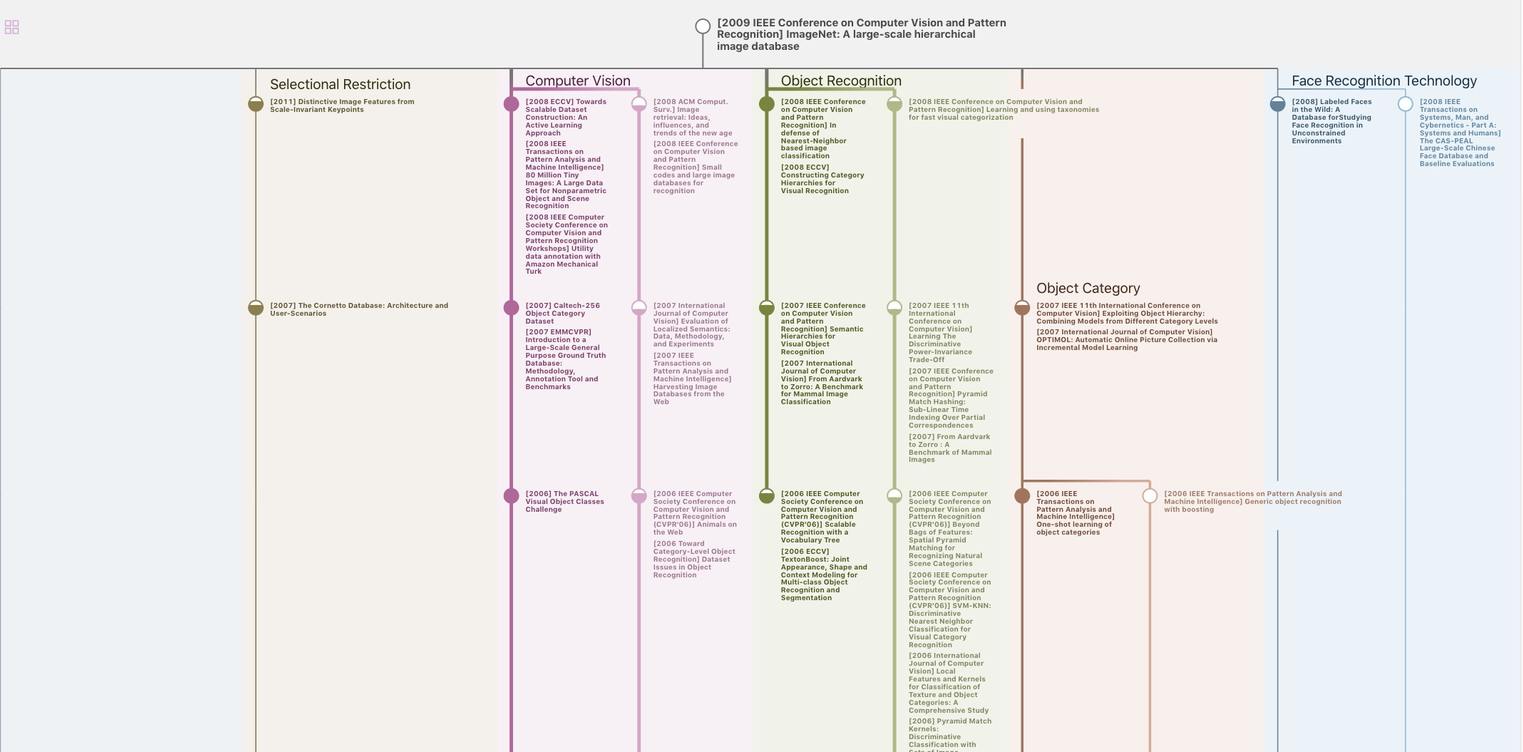
生成溯源树,研究论文发展脉络
Chat Paper
正在生成论文摘要