End-To-End Learning Of Compressible Features
2020 IEEE INTERNATIONAL CONFERENCE ON IMAGE PROCESSING (ICIP)(2020)
摘要
Pre-trained convolutional neural networks (CNNs) are powerful off-the-shelf feature generators and have been shown to perform very well on a variety of tasks. Unfortunately, the generated features are high dimensional and expensive to store: potentially hundreds of thousands of floats per example when processing videos. Traditional entropy based lossless compression methods are of little help as they do not yield desired level of compression, while general purpose lossy compression methods based on energy compaction (e.g. PCA followed by quantization and entropy coding) are sub-optimal, as they are not tuned to task specific objective. We propose a learned method that jointly optimizes for compressibility along with the task objective for learning the features. The plug-in nature of our method makes it straight-forward to integrate with any target objective and trade-off against compressibility. We present results on multiple benchmarks and demonstrate that our method produces features that are an order of magnitude more compressible, while having a regularization effect that leads to a consistent improvement in accuracy.
更多查看译文
关键词
Feature compression, Neural networks
AI 理解论文
溯源树
样例
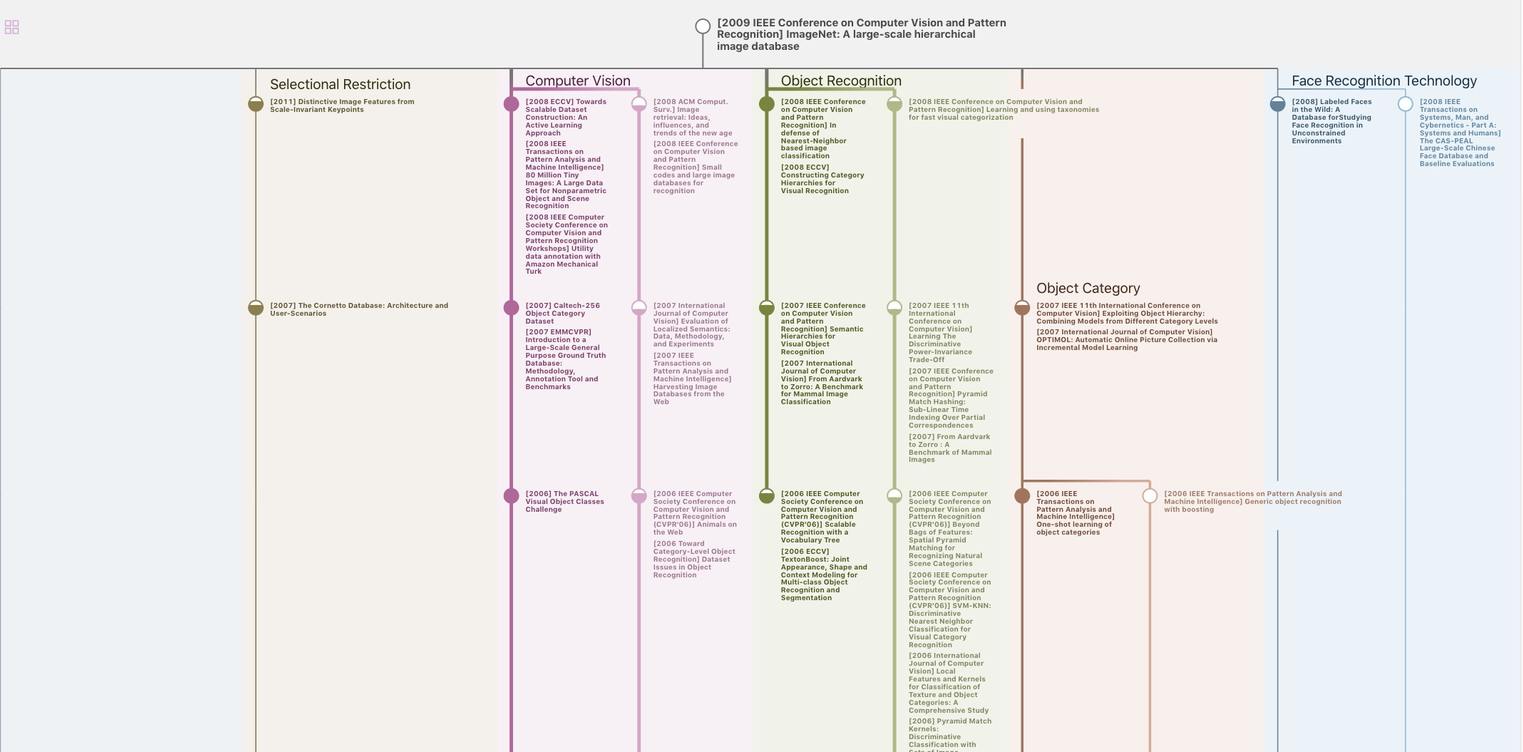
生成溯源树,研究论文发展脉络
Chat Paper
正在生成论文摘要