Batch Policy Learning in Average Reward Markov Decision Processes
arxiv(2022)
摘要
We consider the batch (off-line) policy learning problem in the infinite horizon Markov Decision Process. Motivated by mobile health applications, we focus on learning a policy that maximizes the long-term average reward. We propose a doubly robust estimator for the average reward and show that it achieves semiparametric efficiency. Further we develop an optimization algorithm to compute the optimal policy in a parameterized stochastic policy class. The performance of the estimated policy is measured by the difference between the optimal average reward in the policy class and the average reward of the estimated policy and we establish a finite-sample regret guarantee. The performance of the method is illustrated by simulation studies and an analysis of a mobile health study promoting physical activity.
更多查看译文
关键词
Markov decision process,average reward,policy optimization,doubly robust estima-tor
AI 理解论文
溯源树
样例
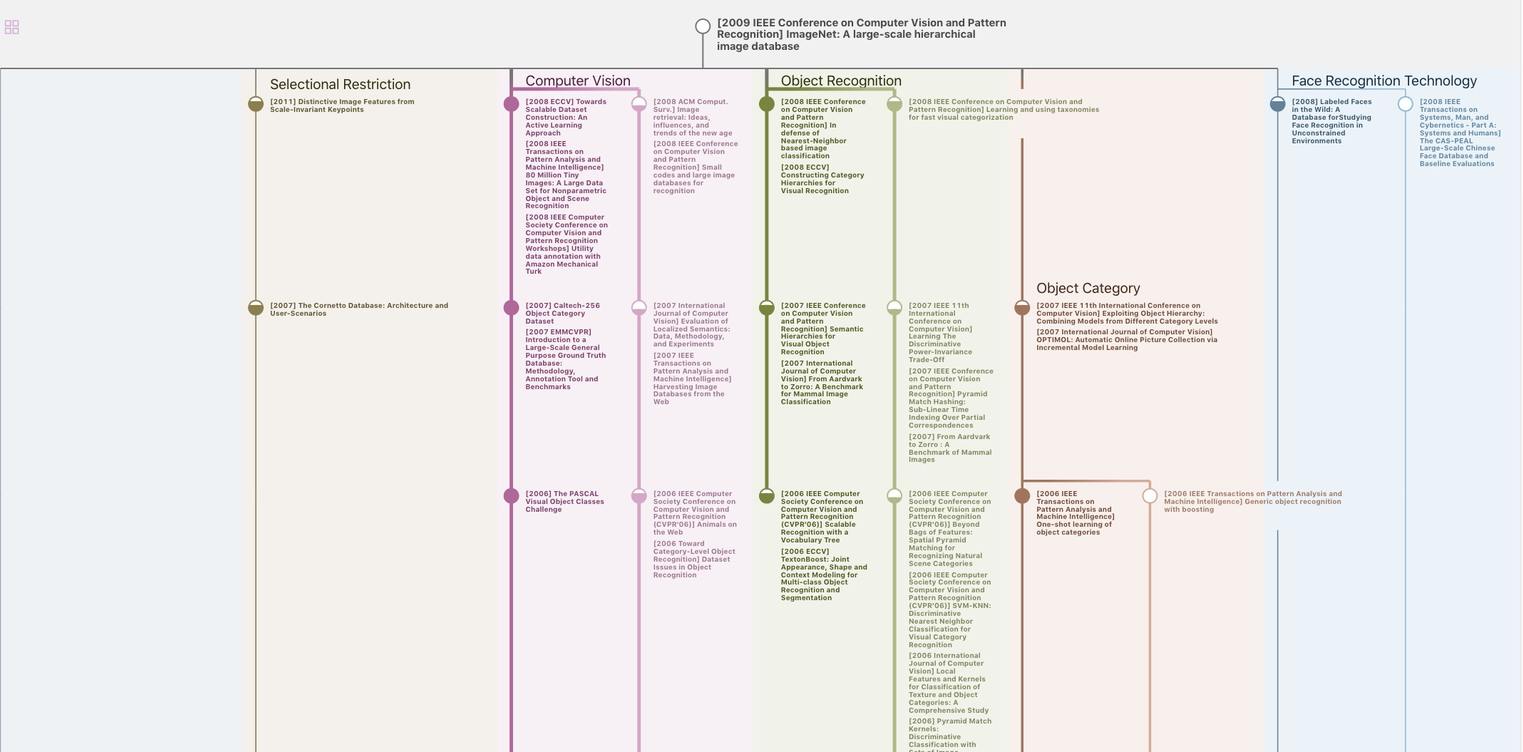
生成溯源树,研究论文发展脉络
Chat Paper
正在生成论文摘要