Preconditioned Gradient Descent Algorithm For Inverse Filtering On Spatially Distributed Networks
IEEE SIGNAL PROCESSING LETTERS(2020)
Abstract
Graph filters and their inverses have been widely used in denoising, smoothing, sampling, interpolating and learning. Implementation of an inverse filtering procedure on spatially distributed networks (SDNs) is a remarkable challenge, as each agent on an SDN is equipped with a data processing subsystem with limited capacity and a communication subsystem with confined range due to engineering limitations. In this letter, we introduce a preconditioned gradient descent algorithm to implement the inverse filtering procedure associated with a graph filter having small geodesic-width. The proposed algorithm converges exponentially, and it can be implemented at vertex level and applied to time-varying inverse filtering on SDNs.
MoreTranslated text
Key words
Signal processing algorithms, Approximation algorithms, Iterative methods, Data processing, Symmetric matrices, Linear systems, Filtering, Graph signal processing, inverse filtering, spatially distributed network, gradient descent method, preconditioning, quasi-Newton method
AI Read Science
Must-Reading Tree
Example
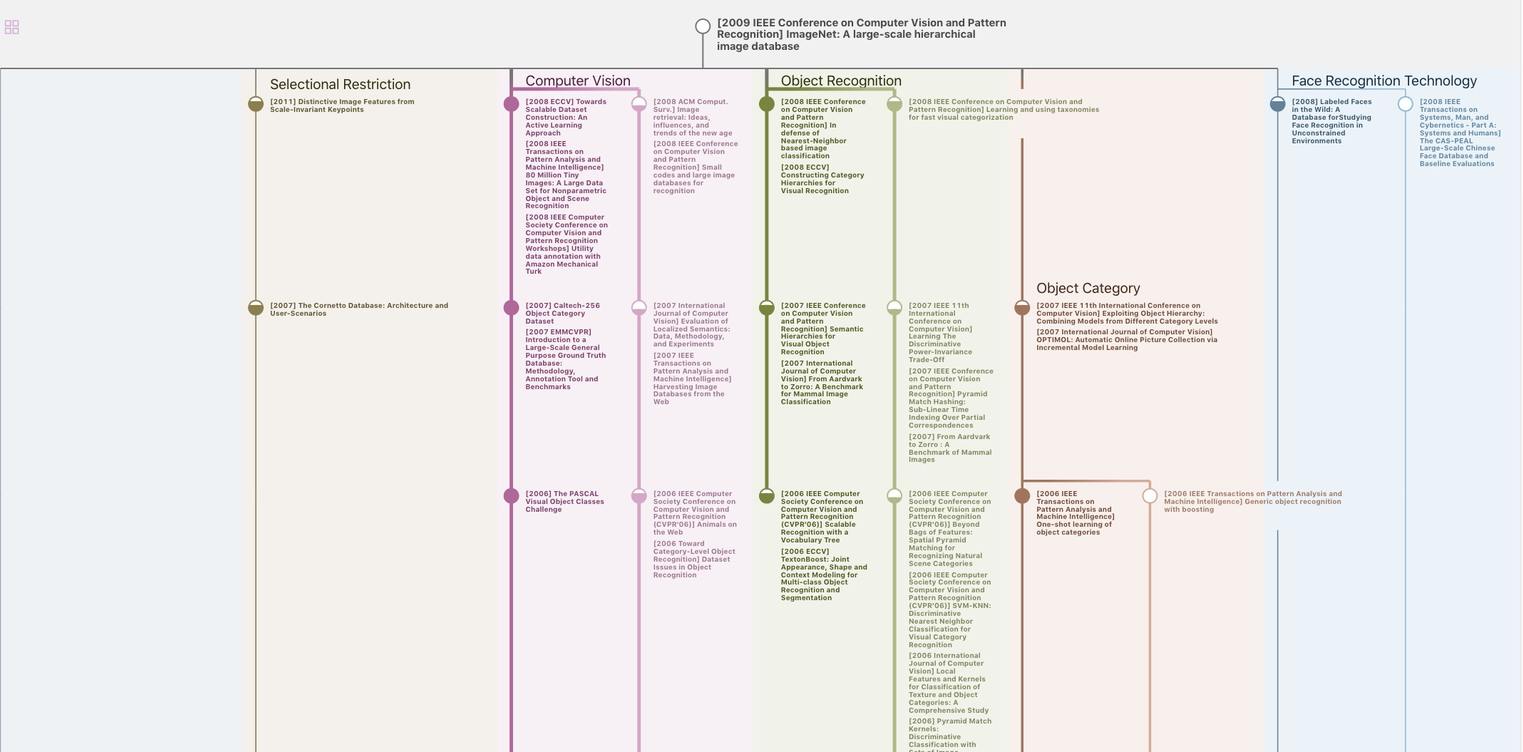
Generate MRT to find the research sequence of this paper
Chat Paper
Summary is being generated by the instructions you defined