GarNet++: Improving Fast and Accurate Static3D Cloth Draping by Curvature Loss
IEEE Transactions on Pattern Analysis and Machine Intelligence(2020)
Abstract
In this paper, we tackle the problem of static 3D cloth draping on virtual human bodies. We introduce a two-stream deep network model that produces a visually plausible draping of a template cloth on virtual 3D bodies by extracting features from both the body and garment shapes. Our network learns to mimic a Physics-Based Simulation (PBS) method while requiring two orders of magnitude less computation time. To train the network, we introduce loss terms inspired by PBS to produce plausible results and make the model collision-aware. To increase the details of the draped garment, we introduce two loss functions that penalize the difference between the curvature of the predicted cloth and PBS. Particularly, we study the impact of mean curvature normal and a novel detail-preserving loss both qualitatively and quantitatively. Our new curvature loss computes the local covariance matrices of the 3D points, and compares the Rayleigh quotients of the prediction and PBS. This leads to more details while performing favorably or comparably against the loss that considers mean curvature normal vectors in the 3D triangulated meshes. We validate our framework on four garment types for various body shapes and poses. Finally, we achieve superior performance against a recently proposed data-driven method.
MoreTranslated text
Key words
Clothing,Three-dimensional displays,Shape,Computational modeling,Solid modeling,Biological system modeling,Deformable models
AI Read Science
Must-Reading Tree
Example
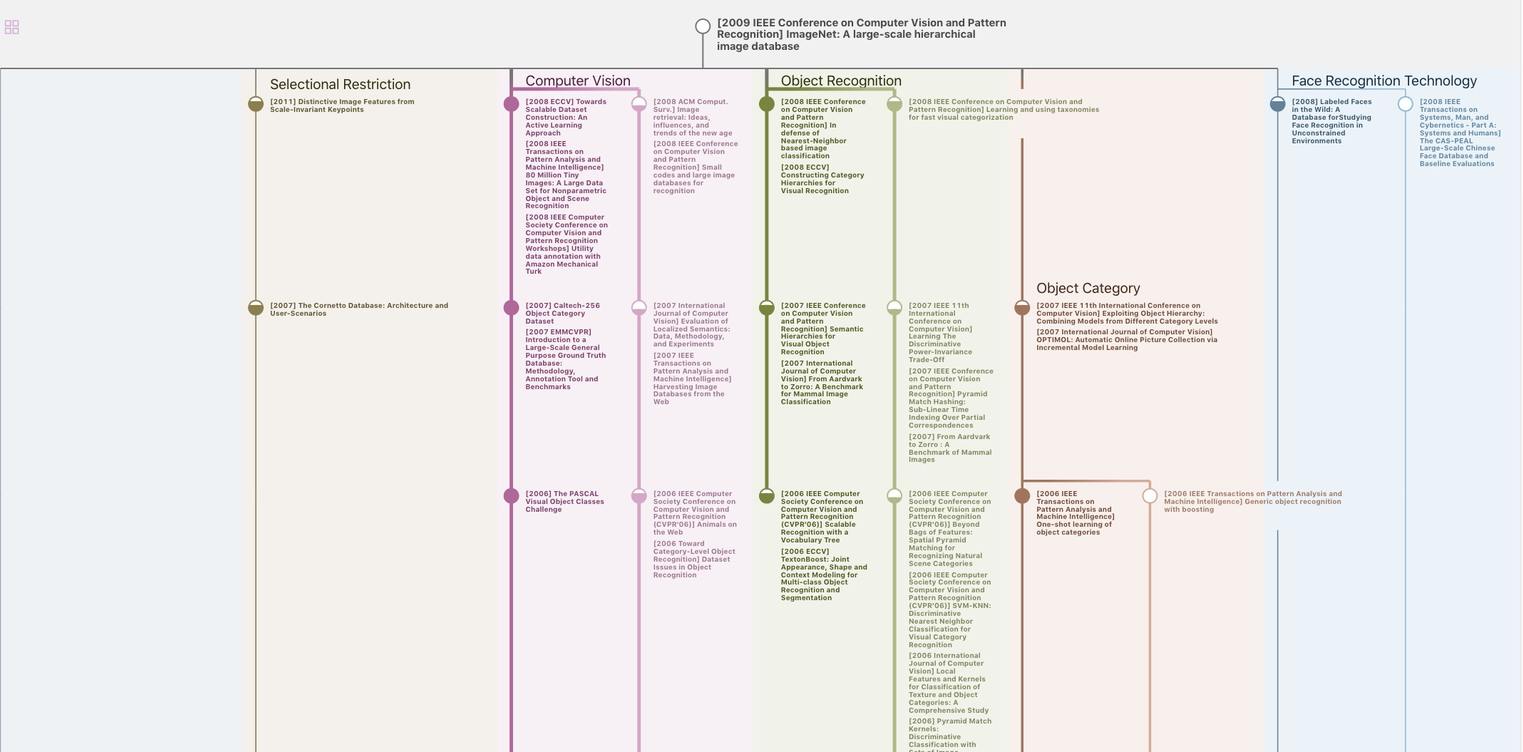
Generate MRT to find the research sequence of this paper
Chat Paper
Summary is being generated by the instructions you defined