Minimax Policy for Heavy-Tailed Bandits
arxiv(2021)
摘要
We study the stochastic Multi-Armed Bandit (MAB) problem under worst-case regret and heavy-tailed reward distribution. We modify the minimax policy MOSS for the sub-Gaussian reward distribution by using saturated empirical mean to design a new algorithm called Robust MOSS. We show that if the moment of order $1+\epsilon $ for the reward distribution exists, then the refined strategy has a worst-case regret matching the lower bound while maintaining a distribution-dependent logarithm regret.
更多查看译文
关键词
policy,heavy-tailed,multi-armed
AI 理解论文
溯源树
样例
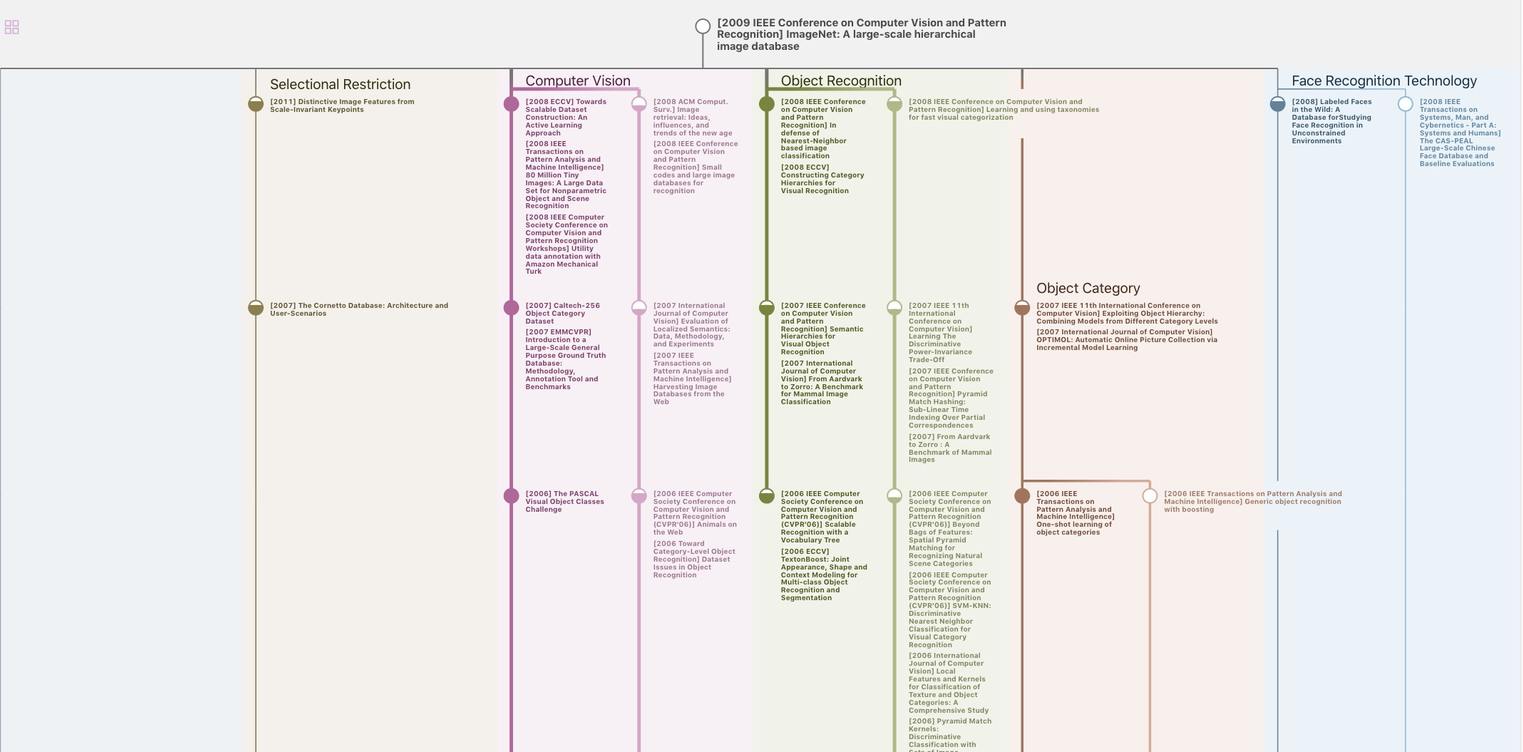
生成溯源树,研究论文发展脉络
Chat Paper
正在生成论文摘要