Image Super Resolution and Uncertainty Quantification for Earth Science Data on the NASA Earth Exchange AI Platform
AGUFM(2018)
摘要
Due to physical and technological constraints, earth science data products often suffer from insufficient spatial and temporal resolution required for remote sensing and climate applications. The class techniques for enhancing the resolution of images, called super-resolution, provides well suited methodology using convolutional neural networks for resolution enhancement of earth science data products. In particular, we present DeepSD, a super-resolution convolutional neural networks (SRCNN) augmented with topographical data to outperform traditional statistical techniques used for climate downscaling. The problem of image super-resolution is generally ill-posed which introduces uncertainty into the estimates. We show that Bayesian deep learning can be incorporated with an SRCNN to quantify and understand both aleatoric and epistemic uncertainties. With climate downscaling as an example, we scale …
更多查看译文
AI 理解论文
溯源树
样例
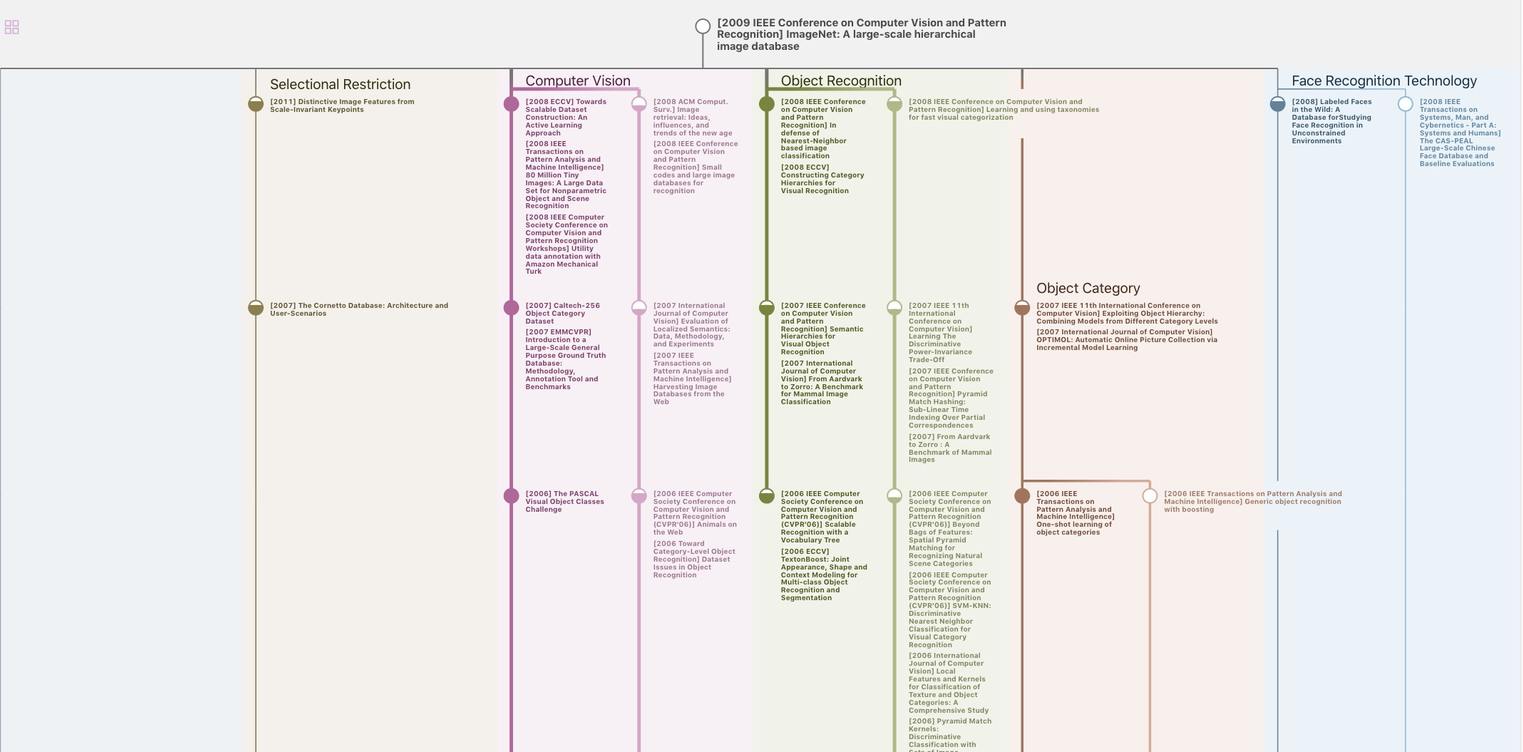
生成溯源树,研究论文发展脉络
Chat Paper
正在生成论文摘要