Power Allocation in Dual Connectivity Networks Based on Actor-Critic Deep Reinforcement Learning
2019 International Symposium on Modeling and Optimization in Mobile, Ad Hoc, and Wireless Networks (WiOPT)(2019)
摘要
Dual Connectivity (DC) has been proposed by Third Generation Partnership Project (3GPP), in order to address the small coverage areas and outage of users and improve the mobility robustness and rate of users in Heterogeneous Networks (HetNets). In the HetNet with DC, each user is assigned a Macro eNode Base Station (MeNB) and a Small eNode Base Station (SeNB) and transmits data to both eNode Base Stations (eNBs), simultaneously. In this paper, we present a power splitting scheme for the HetNet with DC; to maximize the total rate of the users while not exceeding the maximum transmit power of each user. In our proposed power splitting scheme, a Deep Reinforcement Learning (DRL) approach is taken based on the actor-critic model on continuous state-action spaces. Simulation results demonstrate that our power splitting scheme outperforms the baseline approaches in terms of total rate of users and fairness.
更多查看译文
关键词
Dual connectivity,heterogeneous networks,power allocation,deep reinforcement learning
AI 理解论文
溯源树
样例
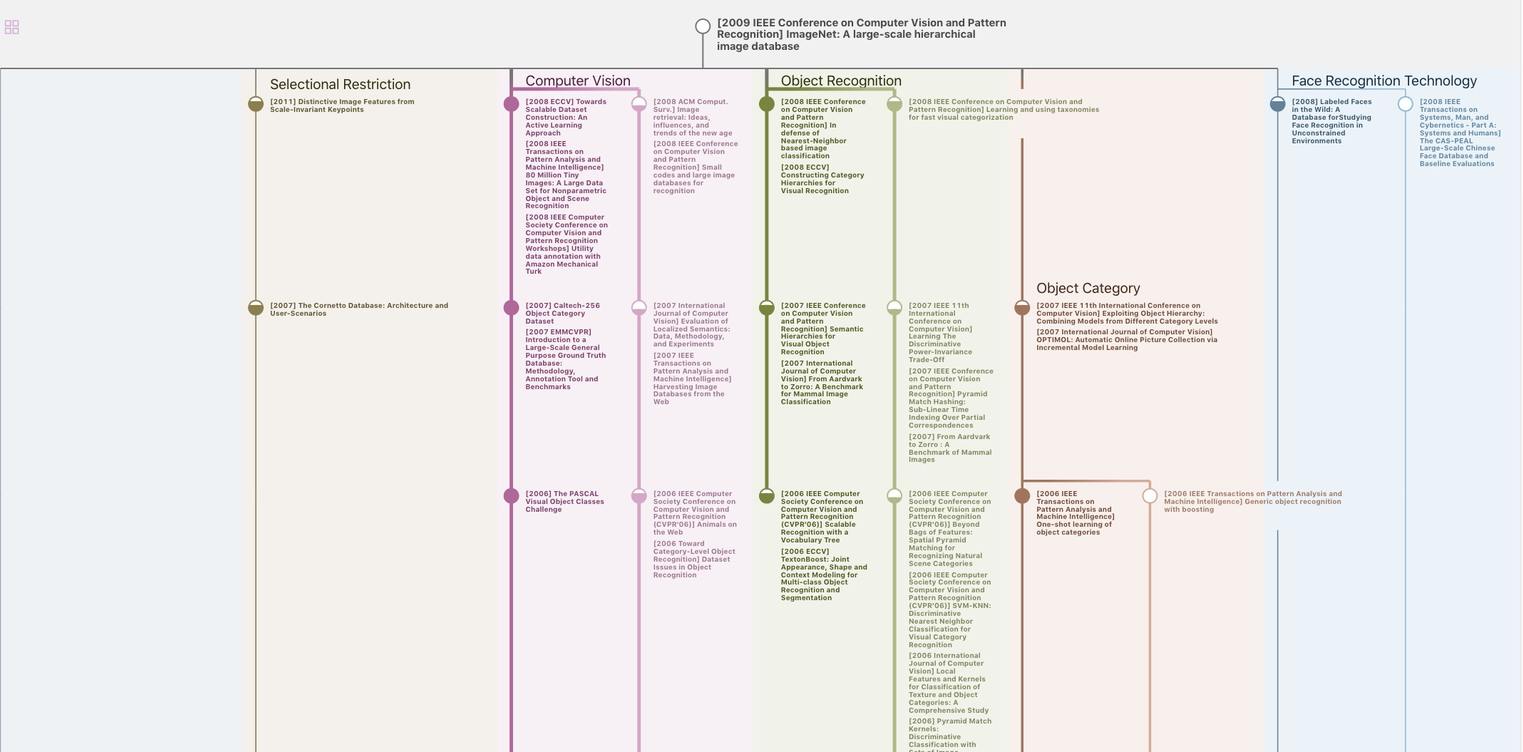
生成溯源树,研究论文发展脉络
Chat Paper
正在生成论文摘要