Variable selection in sparse GLARMA models
STATISTICS(2022)
摘要
In this paper, we propose a novel and efficient two-stage variable selection approach for sparse GLARMA models, which are pervasive for modelling discrete-valued time series. Our approach consists in iteratively combining the estimation of the autoregressive moving average (ARMA) coefficients of GLARMA models with regularized methods designed for performing variable selection in regression coefficients of Generalized Linear Models (GLM). We first establish the consistency of the ARMA part coefficient estimators in a specific case. Then, we explain how to efficiently implement our approach. Finally, we assess the performance of our methodology using synthetic data, compare it with alternative methods and illustrate it on an example of real-world application. Our approach, which is implemented in the GlarmaVarSel R package, is very attractive since it benefits from a low computational load and is able to outperform the other methods in terms of recovering the non-null regression coefficients.
更多查看译文
关键词
GLARMA models, sparse, discrete-valued time series
AI 理解论文
溯源树
样例
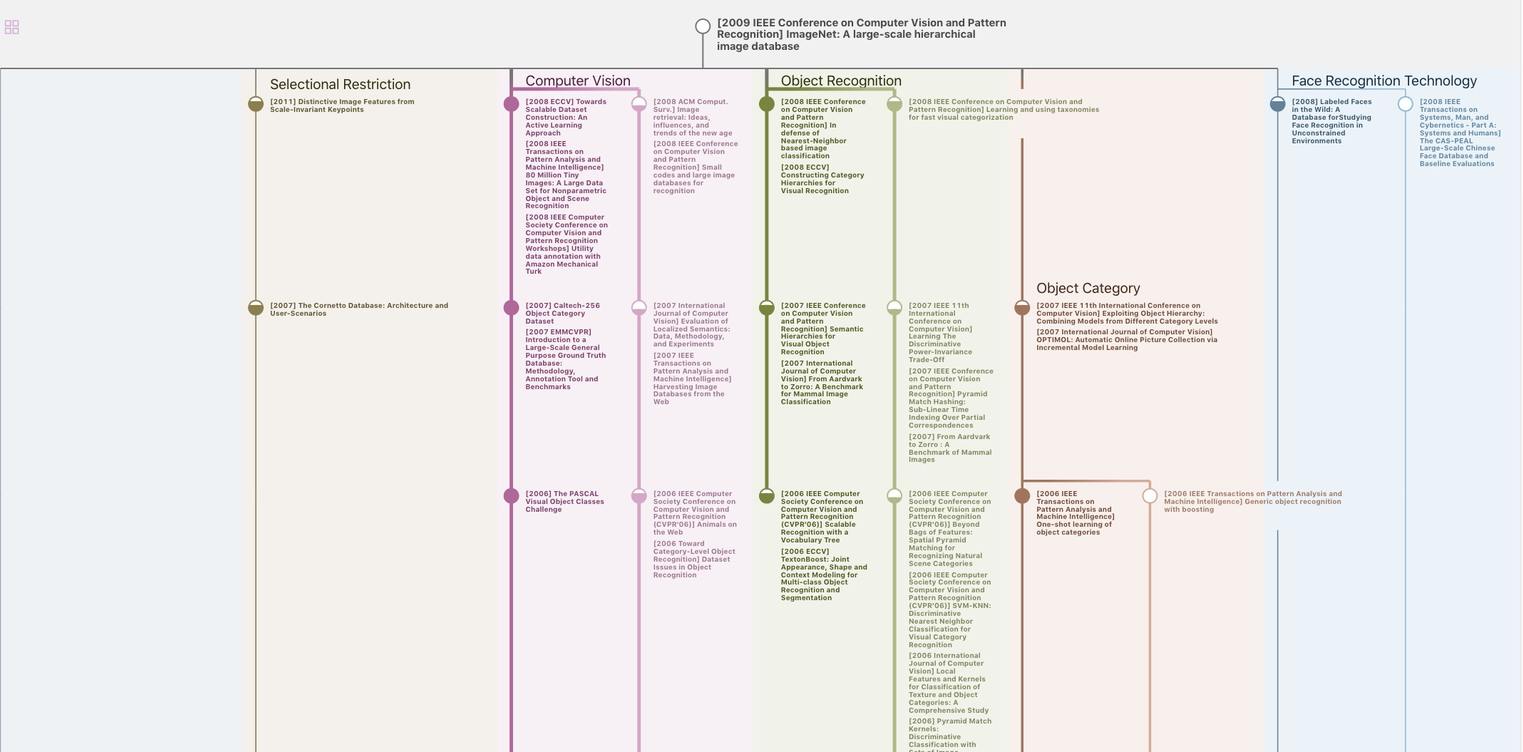
生成溯源树,研究论文发展脉络
Chat Paper
正在生成论文摘要