On the Complexity of Sequential Incentive Design
IEEE Transactions on Automatic Control(2022)
摘要
In many scenarios, a principal dynamically interacts with an agent and offers a sequence of incentives to align the agent’s behavior with a desired objective. This article focuses on the problem of synthesizing an incentive sequence that, once offered, induces the desired agent behavior even when the agent’s intrinsic motivation is unknown to the principal. We model the agent’s behavior as a Markov decision process, express its intrinsic motivation as a reward function, which belongs to a finite set of possible reward functions, and consider the incentives as additional rewards offered to the agent. We first show that the behavior modification problem (BMP), i.e., the problem of synthesizing an incentive sequence that induces a desired agent behavior at minimum total cost to the principal, is PSPACE-hard. We then show that two NP-complete variants of the BMP can be obtained by imposing certain restrictions on the incentive sequences available to the principal. We also provide a sufficient condition on the set of possible reward functions under which the BMP can be solved via linear programming. Finally, we propose two algorithms to compute globally and locally optimal solutions to the NP-complete variants of the BMP.
更多查看译文
关键词
Computational complexity,Markov processes,principal-agent interactions,optimization
AI 理解论文
溯源树
样例
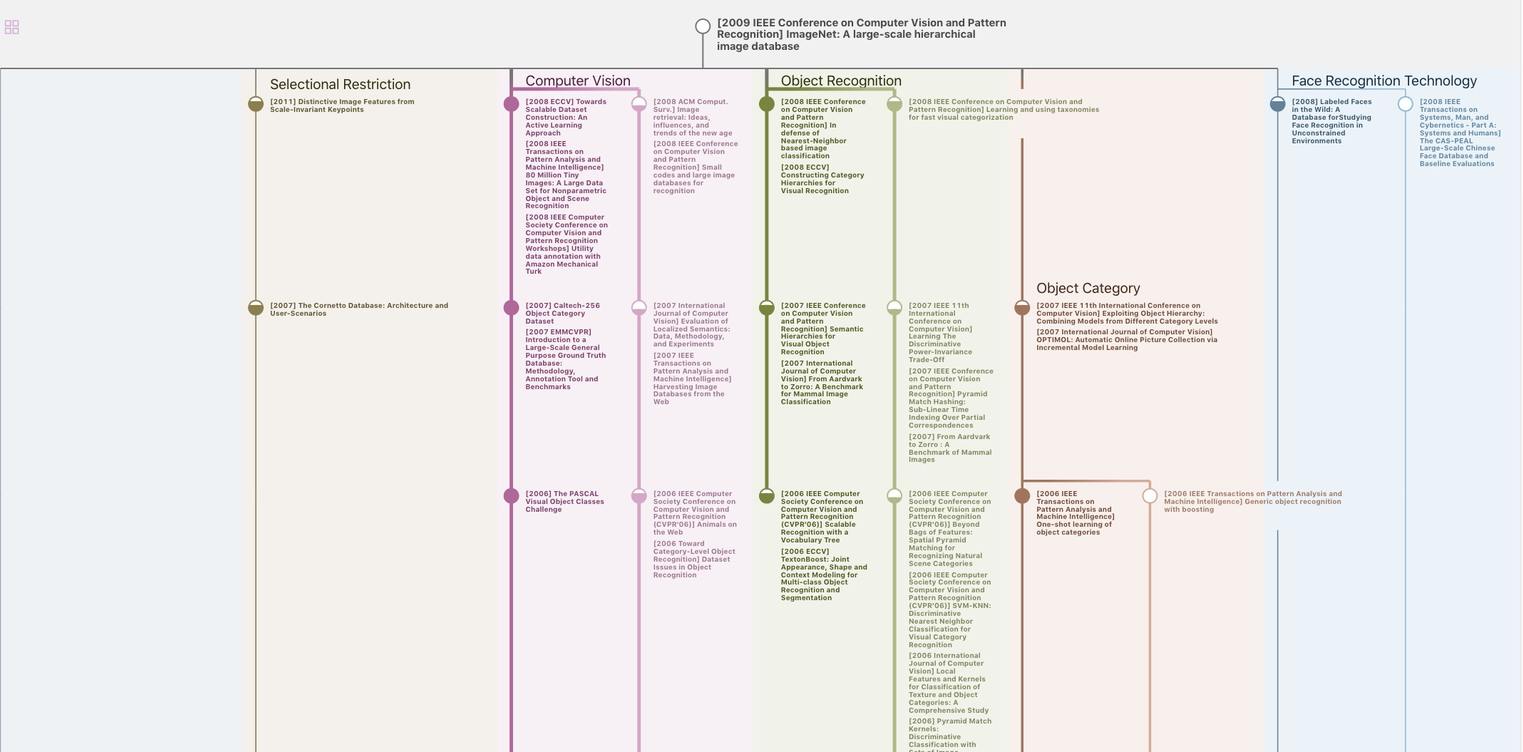
生成溯源树,研究论文发展脉络
Chat Paper
正在生成论文摘要