Weakly Non-Rigid Mr-Trus Prostate Registration Using Fully Convolutional And Recurrent Neural Networks
MEDICAL IMAGING 2020: IMAGE PROCESSING(2021)
摘要
In this study, we propose a new deep learning (DL) framework, a combination of fully convolutional and recurrent neural networks, and integrate them with a weakly supervised method for 3D MRI-transrectal ultrasound (TRUS) image registration. The MR and TRUS images are often highly anisotropic in its dimensions. For instance, in 3D US images the scale of each voxel in depth is often 5 similar to 10 times larger than that in each image slice. This high anisotropy makes the common 3D isotropic kernel suffer poor generality, resulting in unsatisfactory registration results in terms of accuracy. The key idea of the paper is to explicitly leverage 3D image anisotropy through the exploitation of the intra-slice context with a fully convolutional network (FCN) and the utilization of the inter-slice context with a recurrent neural network (RNN). After the 3D hierarchical features in MRI and TRUS have been extracted, we generate the dense deformation field by aligning corresponding prostate labels for individual image pairs. Experimental results showed that our proposed FCN-RNN neural network produces a mean target registration error (TRE) of 2.77 +/- 1.40 mm, and a mean dice similarity coefficient (DSC) of 0.9.
更多查看译文
关键词
weakly supervised method, MR-TRUS registration, RNN, FCN, prostate
AI 理解论文
溯源树
样例
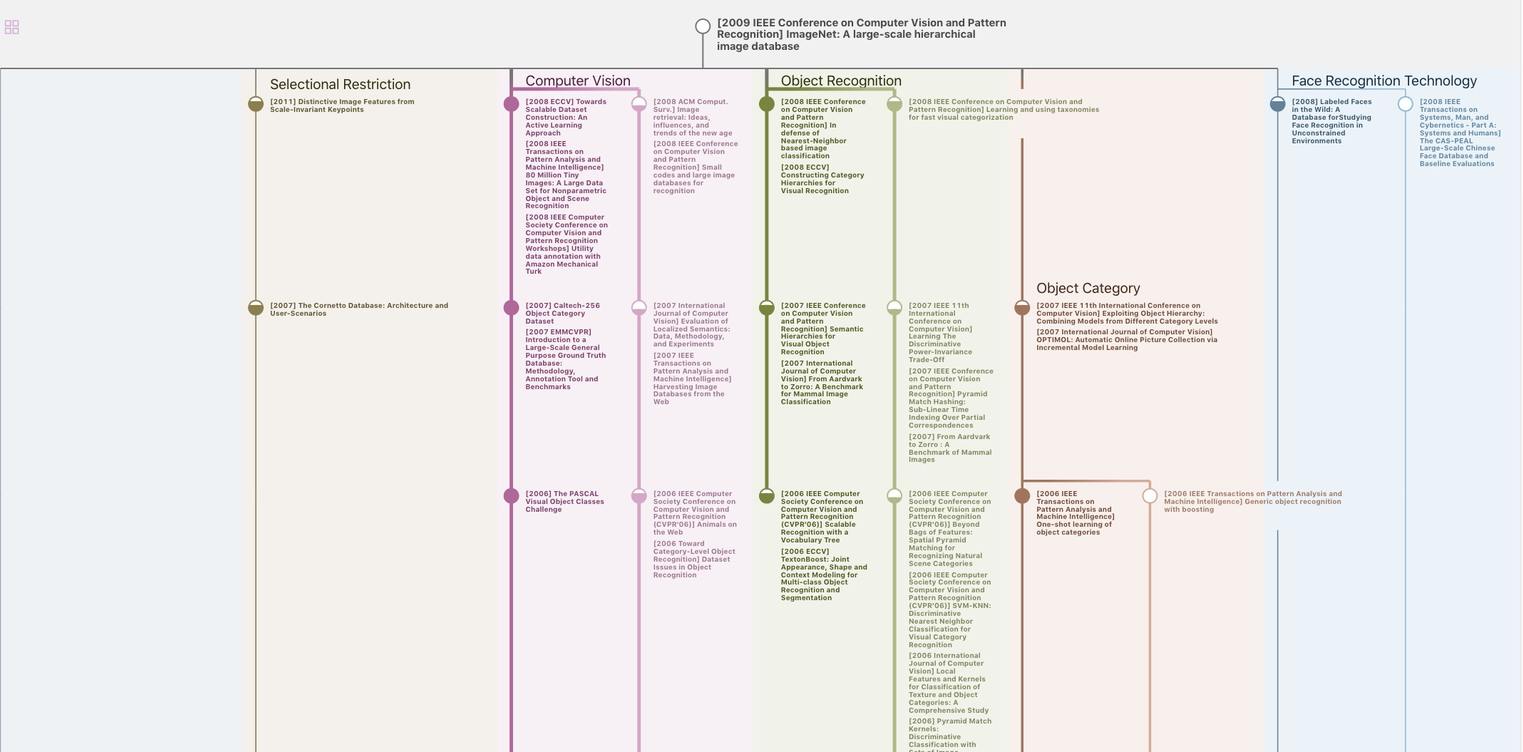
生成溯源树,研究论文发展脉络
Chat Paper
正在生成论文摘要