Reduction Of Motion Artifacts In Head Ct Exams Using Multi-Scale Convolutional Neural Network
MEDICAL IMAGING 2020: IMAGE PROCESSING(2021)
摘要
Patient motion during computed tomography (CT) scan can result in serious degradation of imaging quality, and is of increasing concern due to the aging population and associated diseases. In this paper, we address this problem by focusing on the reduction of head motion artifacts. To achieve this, we introduce a head motion simulation system and a multi-scale deep learning architecture. The proposed motion simulation system can simulate rigid movement including translation and rotation. The images with simulated motion serve as the training set for the network, and the original motion free images serve as the gold standard. Motion artifacts exhibit in the image space as streaks and patchy shadows. We propose a multiscale neural network to learn the artifact. With different branches equipped with ResBlock and down-sampling, the network can learn long scale streaks and short scale shadow artifacts. Although we trained the network on simulated images, we find that the learned network generalizes well to images with real motion artifacts.
更多查看译文
关键词
computed tomography, head motion artifacts, convolutional neural network
AI 理解论文
溯源树
样例
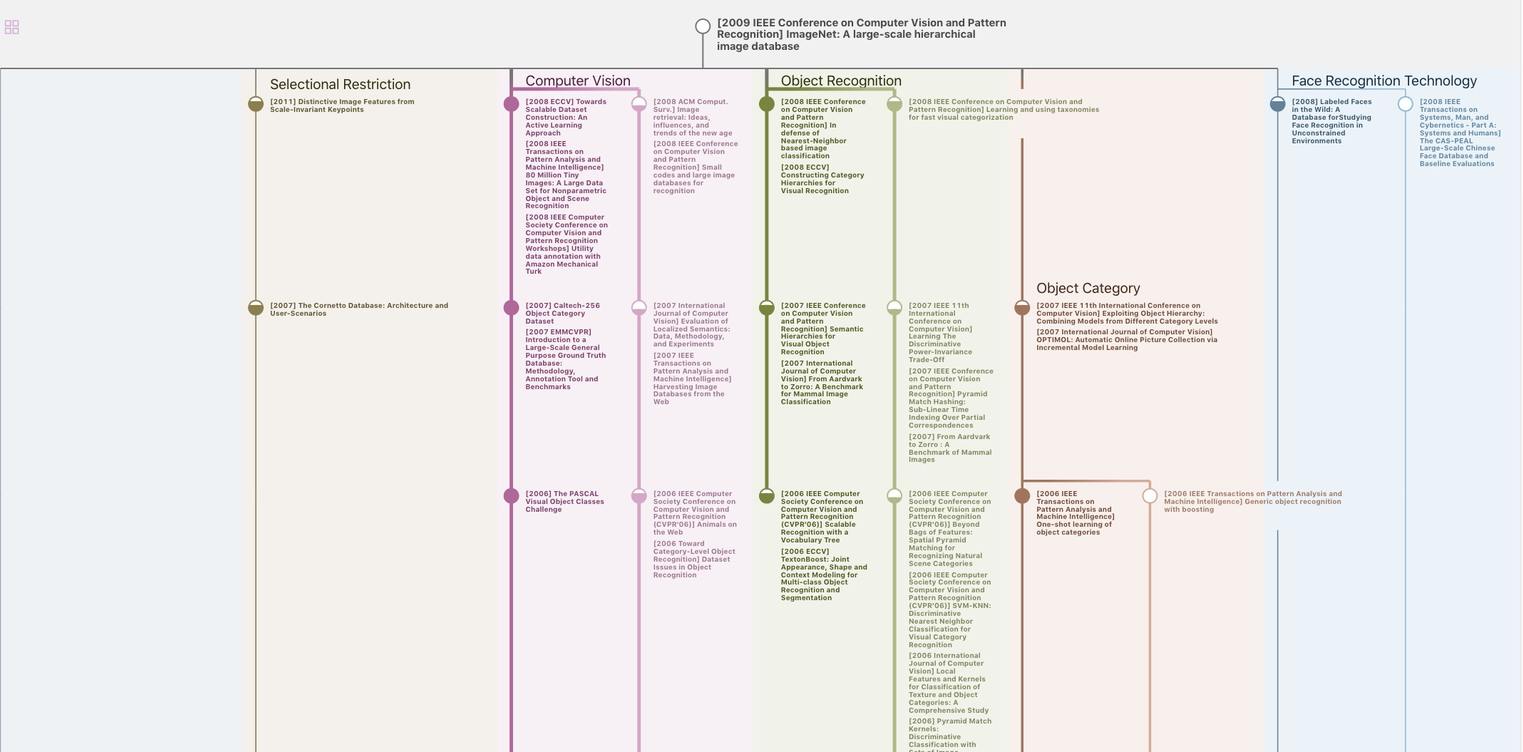
生成溯源树,研究论文发展脉络
Chat Paper
正在生成论文摘要