SlimDeblurGAN-Based Motion Deblurring and Marker Detection for Autonomous Drone Landing.
SENSORS(2020)
摘要
Deep learning-based marker detection for autonomous drone landing is widely studied, due to its superior detection performance. However, no study was reported to address non-uniform motion-blurred input images, and most of the previous handcrafted and deep learning-based methods failed to operate with these challenging inputs. To solve this problem, we propose a deep learning-based marker detection method for autonomous drone landing, by (1) introducing a two-phase framework of deblurring and object detection, by adopting a slimmed version of deblur generative adversarial network (DeblurGAN) model and a You only look once version 2 (YOLOv2) detector, respectively, and (2) considering the balance between the processing time and accuracy of the system. To this end, we propose a channel-pruning framework for slimming the DeblurGAN model called SlimDeblurGAN, without significant accuracy degradation. The experimental results on the two datasets showed that our proposed method exhibited higher performance and greater robustness than the previous methods, in both deburring and marker detection.
更多查看译文
关键词
unmanned aerial vehicle,autonomous landing,deep-learning-based motion deblurring and marker detection,network slimming,pruning model
AI 理解论文
溯源树
样例
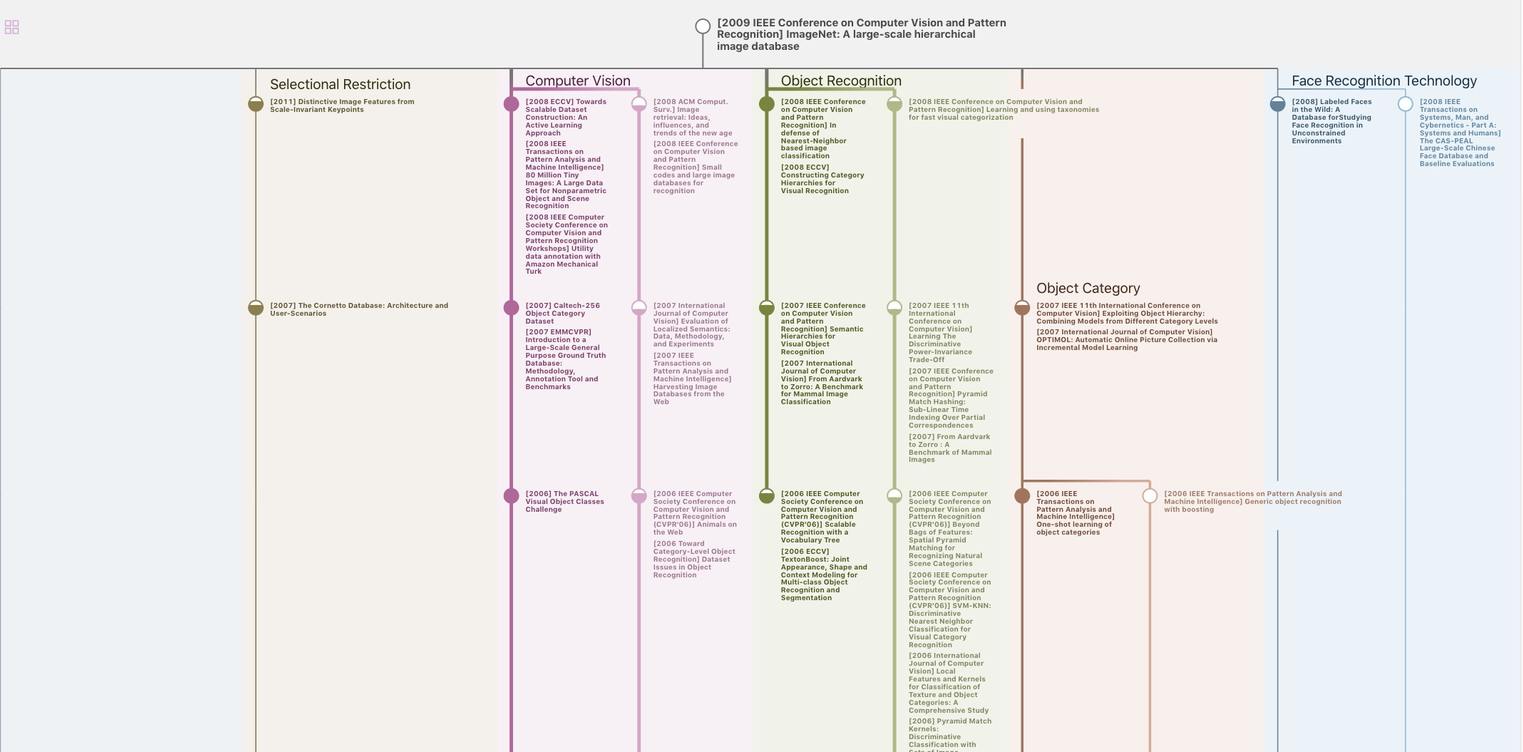
生成溯源树,研究论文发展脉络
Chat Paper
正在生成论文摘要