Incorporating Compositional Heterogeneity Into Lie Markov Models For Phylogenetic Inference
ANNALS OF APPLIED STATISTICS(2020)
摘要
Phylogenetics uses alignments of molecular sequence data to learn about evolutionary trees. Substitutions in sequences are modelled through a continuous-time Markov process, characterised by an instantaneous rate matrix, which standard models assume is time-reversible and stationary. These assumptions are biologically questionable and induce a likelihood function which is invariant to a tree's root position. This hampers inference because a tree's biological interpretation depends critically on where it is rooted. Relaxing both assumptions, we introduce a model whose likelihood can distinguish between rooted trees. The model is nonstationary with step changes in the instantaneous rate matrix at each speciation event. Exploiting recent theoretical work, each rate matrix belongs to a nonreversible family of Lie Markov models. These models are closed under matrix multiplication, so our extension offers the conceptually appealing property that a tree and all its subtrees could have arisen from the same family of nonstationary models.We adopt a Bayesian approach, describe an MCMC algorithm for posterior inference and provide software. The biological insight that our model can provide is illustrated through an analysis in which nonreversible but stationary and nonstationary but reversible models cannot identify a plausible root.
更多查看译文
关键词
Compositional heterogeneity, Lie Markov models, phylogenetics, rooting
AI 理解论文
溯源树
样例
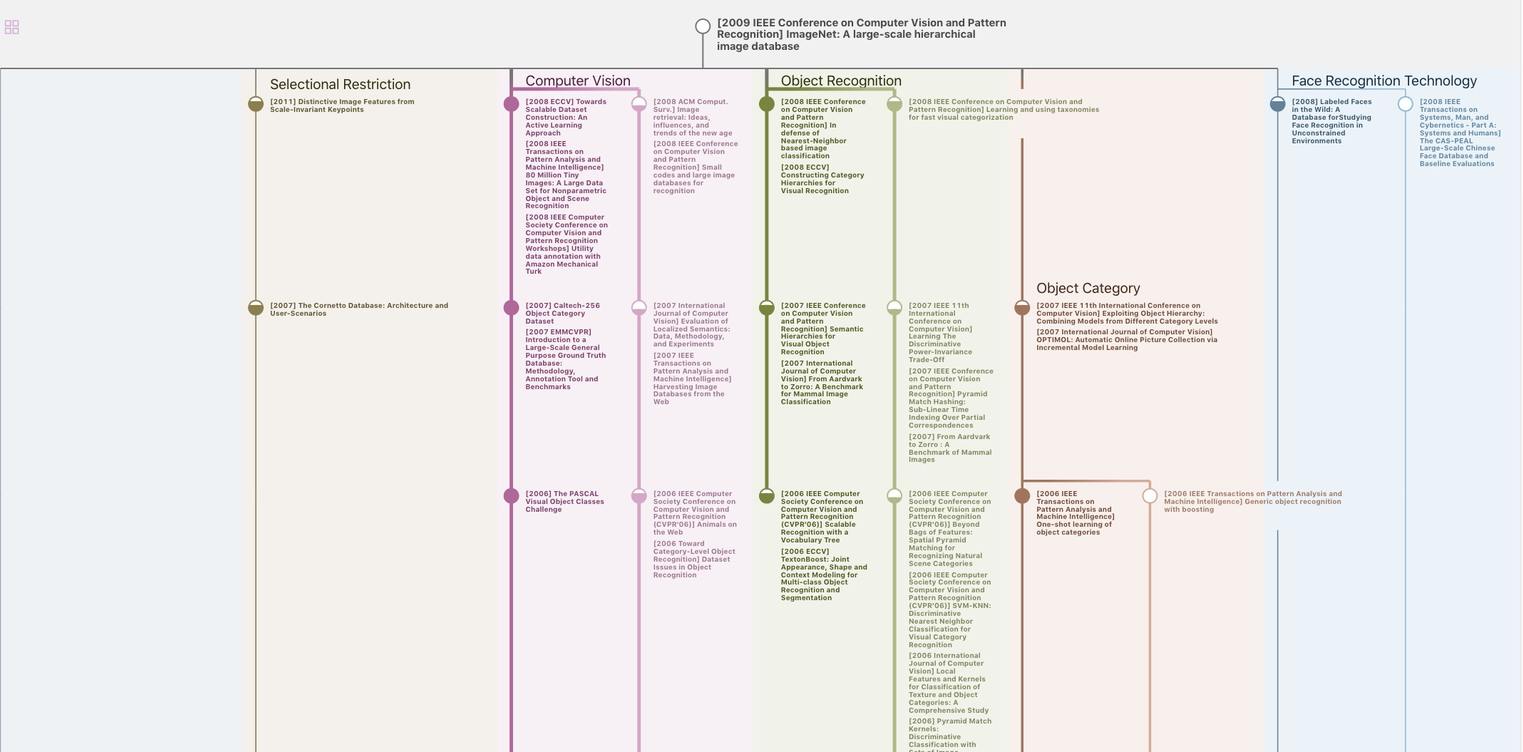
生成溯源树,研究论文发展脉络
Chat Paper
正在生成论文摘要