Enhancing African Low-Resource Languages: Swahili Data For Language Modelling
DATA IN BRIEF(2020)
摘要
Language modelling using neural networks requires adequate data to guarantee quality word representation which is important for natural language processing (NLP) tasks. How ever, African languages, Swahili in particular, have been disadvantaged and most of them are classified as low resource languages because of inadequate data for NLP. In this article, we derive and contribute unannotated Swahili dataset, Swahili syllabic alphabet and Swahili word analogy dataset to address the need for language processing resources especially for low resource languages. Therefore, we derive the unannotated Swahili dataset by pre-processing raw Swahili data using a Python script, formulate the syllabic alphabet and develop the Swahili word analogy dataset based on an existing English dataset. We envisage that the datasets will not only support language models but also other NLP downstream tasks such as part-of-speech tagging, machine translation and sentiment analysis. (C) 2020 The Authors. Published by Elsevier Inc.
更多查看译文
关键词
Natural language processing, Deep learning, Language modelling, Unannotated data, Word analogy, Syllables, Neural networks
AI 理解论文
溯源树
样例
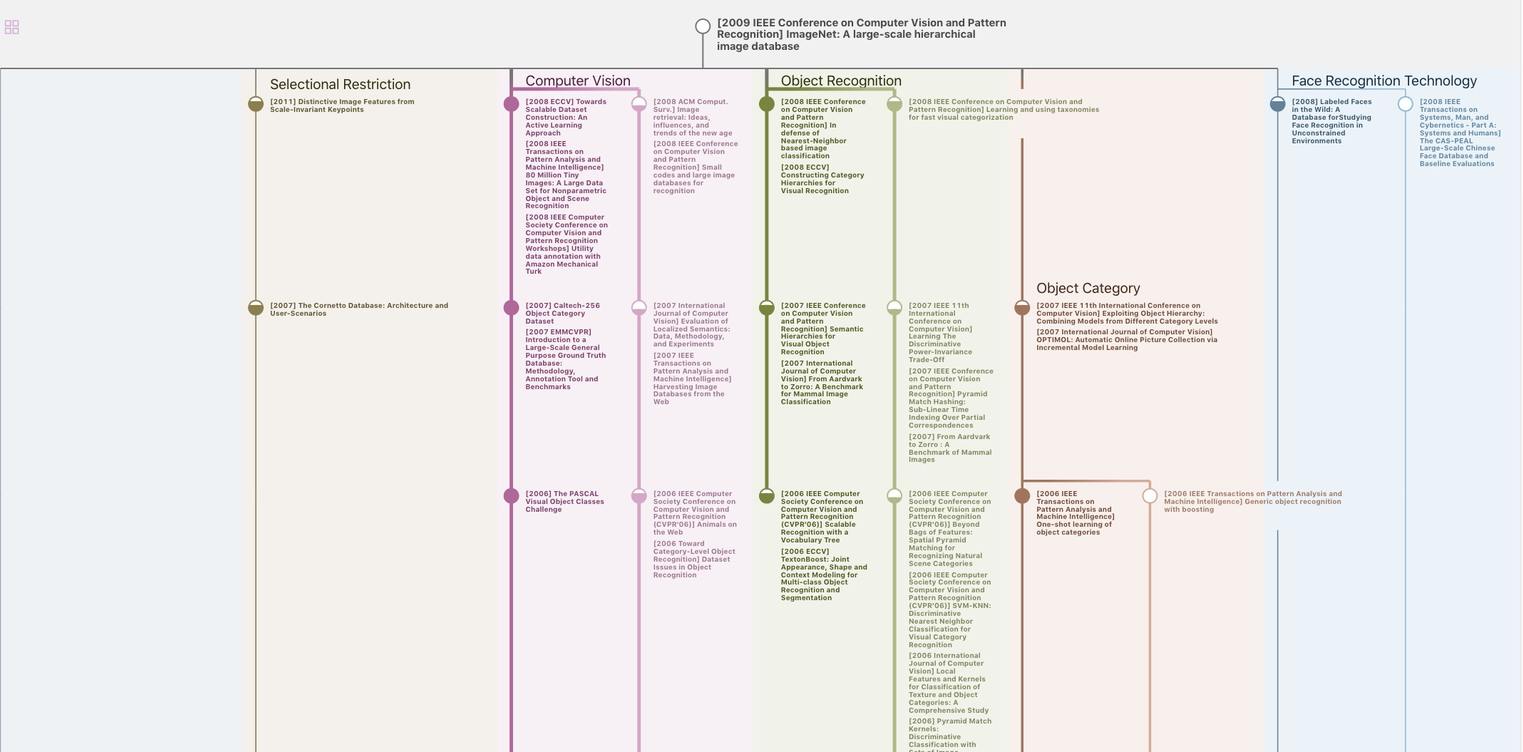
生成溯源树,研究论文发展脉络
Chat Paper
正在生成论文摘要