A Machine Learning Approach to Assess Student Group Collaboration Using Individual Level Behavioral Cues
ECCV Workshops(2020)
摘要
K-12 classrooms consistently integrate collaboration as part of their learning experiences. However, owing to large classroom sizes, teachers do not have the time to properly assess each student and give them feedback. In this paper we propose using simple deep-learning-based machine learning models to automatically determine the overall collaboration quality of a group based on annotations of individual roles and individual level behavior of all the students in the group. We come across the following challenges when building these models: 1) Limited training data, 2) Severe class label imbalance. We address these challenges by using a controlled variant of Mixup data augmentation, a method for generating additional data samples by convexly combining different pairs of data samples and their corresponding class labels. Additionally, the label space for our problem exhibits an ordered structure. We take advantage of this fact and also explore using an ordinal-cross-entropy loss function and study its effects with and without Mixup.
更多查看译文
关键词
Education,Collaboration assessment,Limited training data,Class imbalance,Mixup data augmentation,Ordinal-cross-entropy loss.
AI 理解论文
溯源树
样例
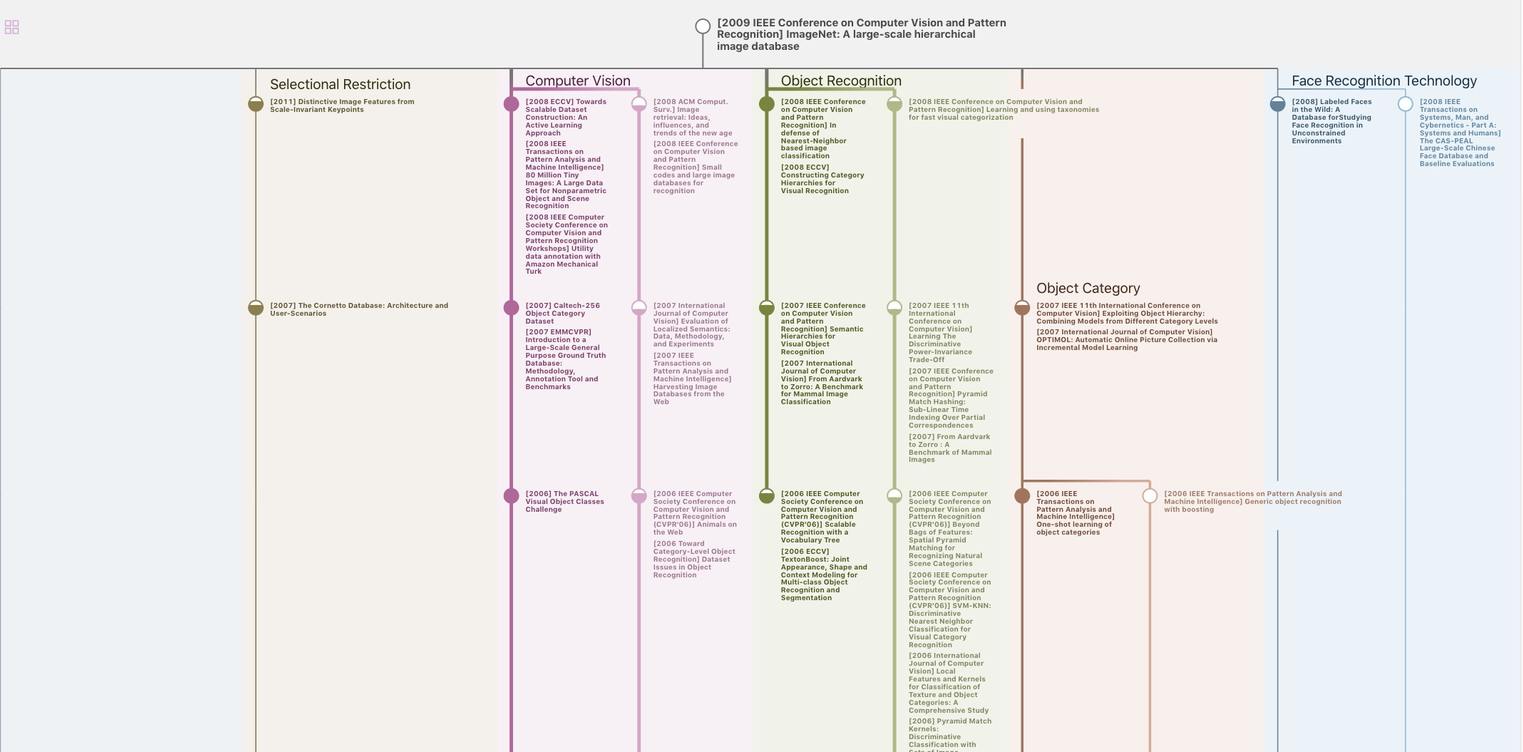
生成溯源树,研究论文发展脉络
Chat Paper
正在生成论文摘要