Distributed Restarting NewtonCG Method for Large-Scale Empirical Risk Minimization
user-5eddf84c4c775e09d87c9229(2017)
摘要
In this paper, we propose a distributed damped Newton method in which sample size is gradually increasing to quickly obtain a solution whose empirical loss is under satisfactory statistical accuracy. Our proposed method is multistage in which the solution of one stage serves as a warm start for the next stage which contains more samples (including the samples in the previous stage). This overall multistage algorithm reduce the number of passes over data. Moreover, our algorithm in nature is easy to be distributed and shares the strong scaling property indicating that acceleration is always expected by using more computing nodes. Various iteration complexity results regarding descent direction computation and stopping criteria are analyzed under convex setting. Our results of experiments illustrate that the proposed algorithm can outperform other comparable methods for training machine learning tasks including neural networks.
更多查看译文
AI 理解论文
溯源树
样例
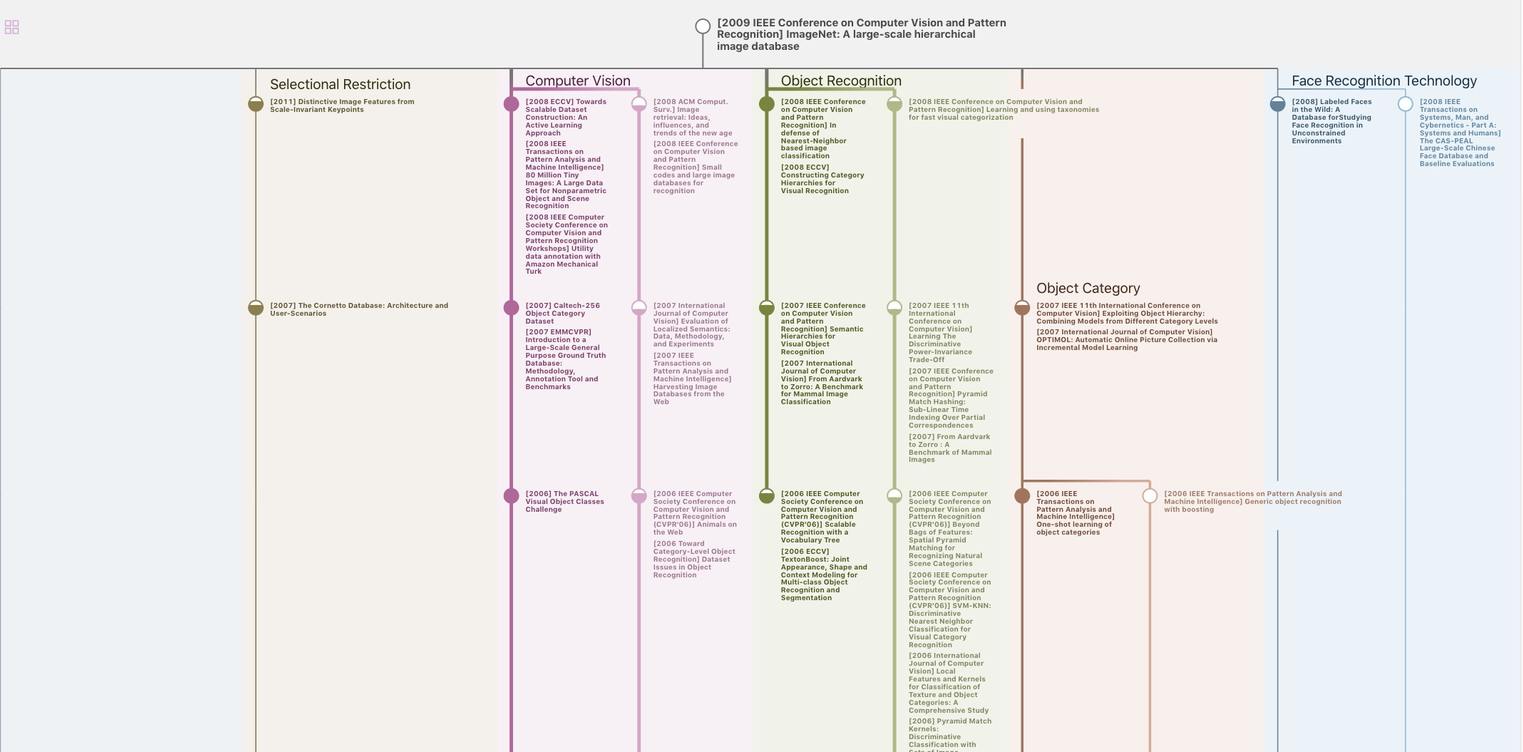
生成溯源树,研究论文发展脉络
Chat Paper
正在生成论文摘要