基于改进的Faster R-CNN的目标检测与识别
semanticscholar(2019)
摘要
In recent years, with the continuous development of in-depth learning, image research and application based on in-depth learning has achieved excellent results in many fields. RCNN network and full convolution network make the development of target detection technology more and more rapid. Faster R-CNN algorithm has been proposed and widely used in the field of target detection and recognition. In this paper, we mainly study the object detection based on Faster R-CNN algorithm for the image in the data set of self-made office supplies. Compared with RCNN series algorithms, Faster R-CNN proposes a regional recommendation network, and integrates feature extraction, candidate box extraction, boundary box regression and classification into a network, which greatly improves the overall performance. In this paper, an improved Faster R-CNN algorithm based on activation function is proposed. When extracting features, the data set usually has a large number of high-density continuity characteristics, while the activation function is sparse, which solves the problem of target detection of office supplies under small targets and complex background, and improves the detection speed and accuracy.
更多查看译文
AI 理解论文
溯源树
样例
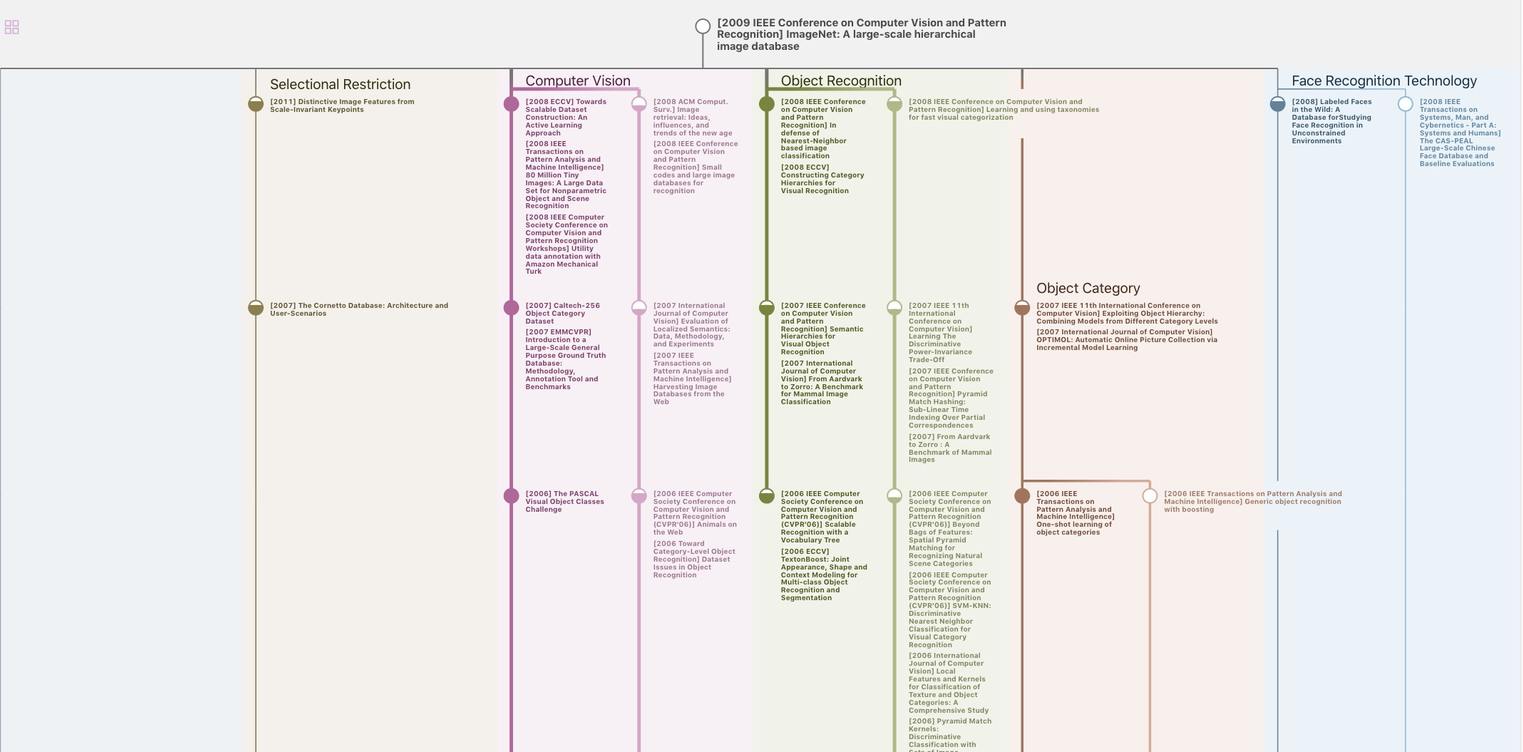
生成溯源树,研究论文发展脉络
Chat Paper
正在生成论文摘要