An Adaptive Early Stopping Strategy for Query-based Passage Re-ranking
semanticscholar(2020)
摘要
Recent research on the semantic retrieval task shows that the pretrained language models like BERT have impressive re-ranking performance[4]. In the re-ranking process, the fine-tuned language model is feed with (query, document) pairs and the whole time complexity is directly proportional to both the query size and the recall set size. In this paper, we describe a simple yet effective strategy of early stopping strategy based on the confidence score. In our experiment, such strategy can avoid up to 30% unnecessary inference computation cost without sacrificingmuch ranking precision. The codes and documents are available at https://github.com/ chengsyuan/WSDM-Adhoc-Document-Retrieval. Our team dlutycx ranked first on the unleak track.
更多查看译文
AI 理解论文
溯源树
样例
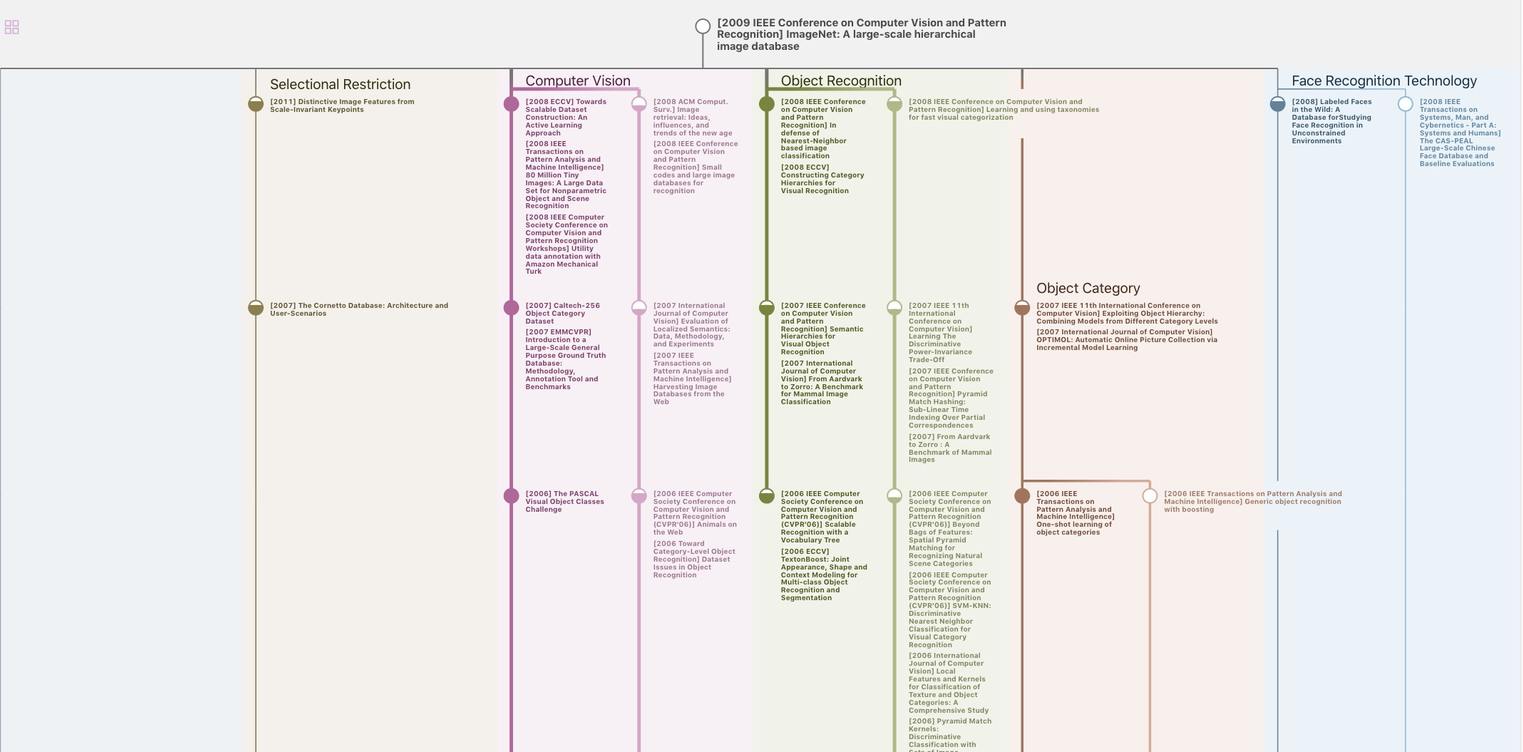
生成溯源树,研究论文发展脉络
Chat Paper
正在生成论文摘要