A Bayesian Approach to Evaluation of Soil Biogeochemical Models
semanticscholar(2020)
摘要
Abstract. To make predictions about the effect of rising global surface temperatures, we rely on mathematical soil biogeochemical models (SBMs). However, it is not clear which models have better predictive accuracy, and a rigorous quantitative approach for comparing and validating the predictions has yet to be established. In this study, we present a Bayesian approach to SBM comparison that can be incorporated into a statistical model selection framework. We compared the fits of a linear and non-linear SBM to soil respiration CO2 flux data compiled in a recent meta-analysis of soil warming field experiments. Fit quality was quantified using two Bayesian goodness-of-fit metrics, the Widely Applicable information criterion (WAIC) and Leave-one-out cross-validation (LOO). We found that the linear model generally out-performed the non-linear model at fitting the meta-analysis data set. Both WAIC and LOO computed a higher overfitting penalty for the non-linear model than the linear model, conditional on the data set. Fits for both models generally improved when they were initialized with lower and more realistic steady state soil organic carbon densities. Testing whether linear models offer definitively superior predictive performance over non-linear models on a global scale will require comparisons with additional site-specific data sets of suitable size and dimensionality. Such comparisons can build upon the approach defined in this study to make more rigorous statistical determinations about model accuracy while leveraging emerging data sets, such as those from long-term ecological research experiments.
更多查看译文
AI 理解论文
溯源树
样例
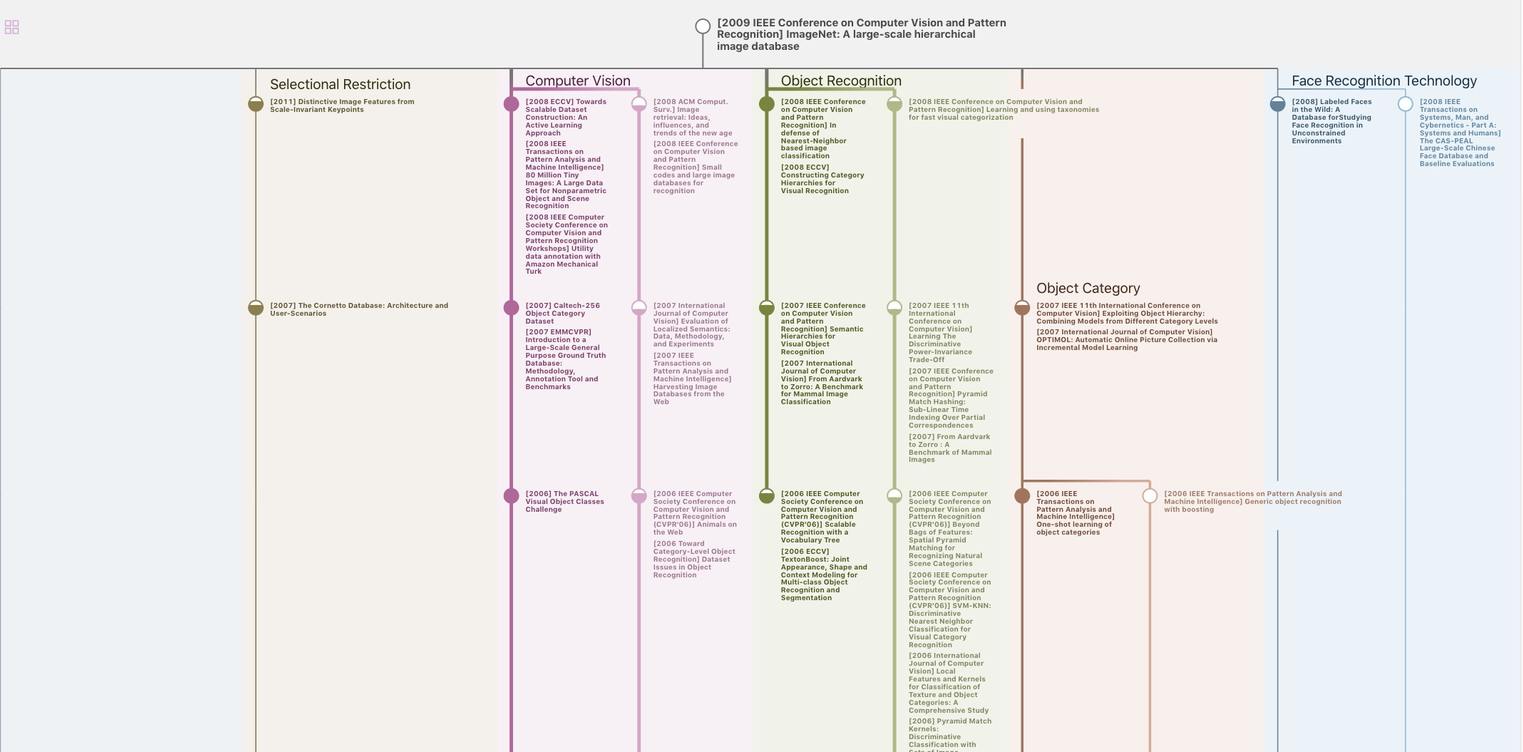
生成溯源树,研究论文发展脉络
Chat Paper
正在生成论文摘要