Predicting Stock Prices and Analyst Recommendations
semanticscholar(2016)
摘要
Since the mid-2000s, virtually all financial trades are executed via computers. Much of that trading occurs algorithmically, with computers executing purchases and sales in response to a market made heavily of other algorithmic traders. 1 About 66 percent of all equity transactions in the United States now are done via high-frequency (algorithmic) trading. 2 As a consequence, market behavior has fundamentally changed. In several occurrences in the past decade, markets experienced ”flash crashes” where one algorithm making a big sale triggered a waterfall of other algorithms to respond similarly and the market tumbled to a fraction of its value within seconds. 3 The rise in computerized trading has been accompanied with increasing efforts to use machine learning to predict future market behavior. Previous research has focused on forecasting movements in price (either as a continuous price or in discrete intervals) or forecasting decisions to buy or sell. These studies have met with mixed results. For both practical and theoretical reasons, the price of traded equities remains difficult to predict, with the task described as ”difficult if not impossible” by some. This is due both to the large number of possible features that could be used to train an algorithm as well as the lack of consensus on what, theoretically, ought to underpin the valuation of a security. 4 Researchers have tried a range of techniques, including supervised learning techniques, artificial neural nets, backpropagation networks, hybrid Kohonen self organizing maps, and other methods. 5 Several prior projects in this course have examined financial trading as well. Although computerized trading has left its mark on equities markets, much of equity transaction today still occurs based on value judgments by humans. These judgments, in turn, often reflect the sentiment of professional equity analysts employed by financial institutions like Goldman Sachs. Indeed, many computer scientists have shifted their focus away from forecasting prices or other stock technical properties and instead focusing on forecasting perceptions.6 A large number of traders conduct market research through Bloomberg terminals. Bloomberg is the market leader in distributing financial data.7 Bloomberg’s market power is so substantial that the UK government had to postpone a major sovereign debt buyback when the Bloomberg network went offline briefly in 2015.8 For public equities, Bloomberg offers a consensus analyst recommendation from 1-5 (strong sell to strong buy) that reflect the aggregate opinion of equity analysts. Prior research on how these recommendations impact the market suggests that they may actually move markets in the opposite direction (fueled, perhaps, by a perception that others with the same information will move in the direction of the recommendation). Regardless of direction, researchers agree that such recommendations have a major impact on market perceptions and prices. 9 We take a novel approach to applying supervised learning to financial modeling. In addition to forecasting the price of an equity in the future, which we treat as a regression problem, we also forecast Bloomberg consensus analyst recommendations, as a classification problem. (Analyst recommendation categories between 1-5). As discussed previously, these consensus numbers have tremendous power to shape market perceptions and are associated with detectable movements in the value of the stock. Prior studies have forecasted price, but little to nothing has been published on forecasting analyst recommendations.
更多查看译文
AI 理解论文
溯源树
样例
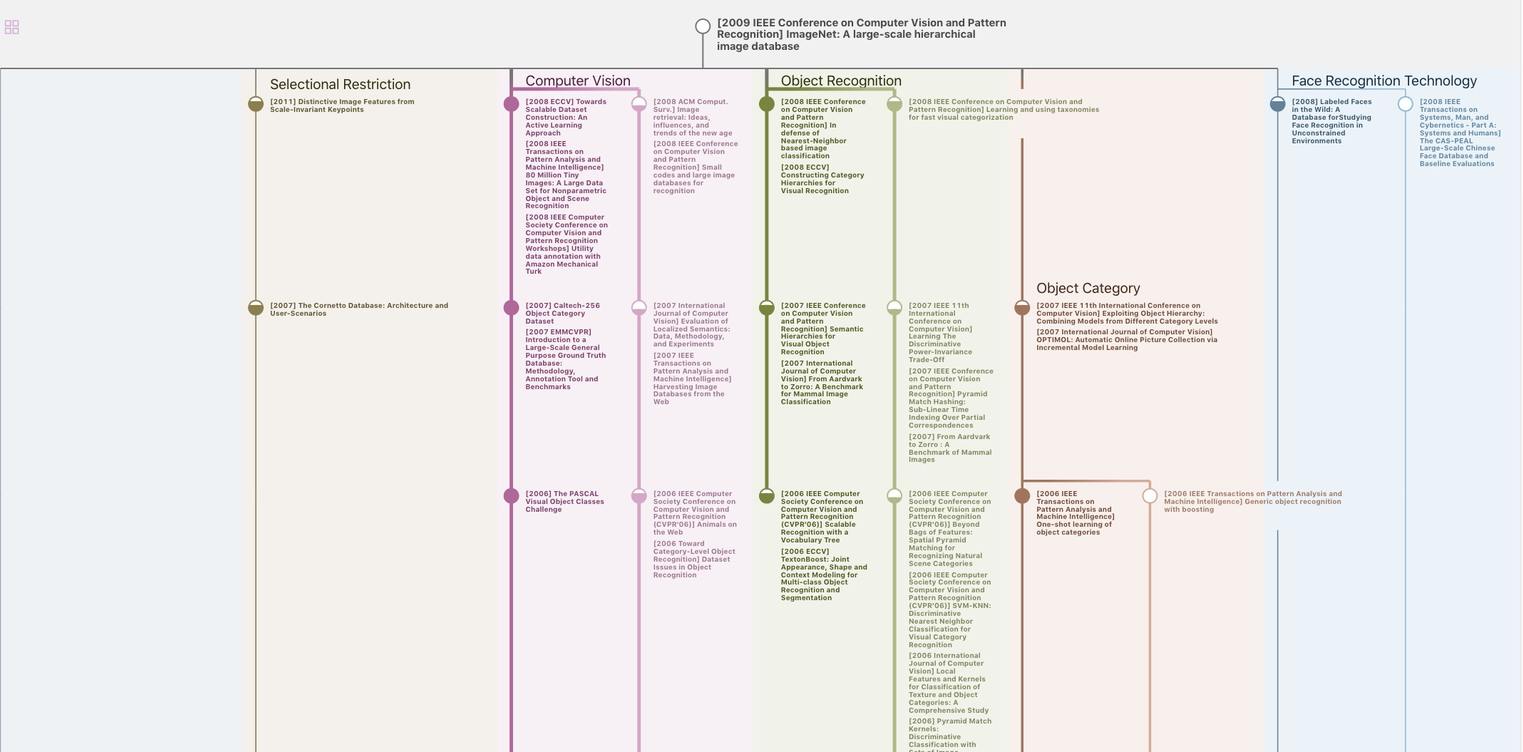
生成溯源树,研究论文发展脉络
Chat Paper
正在生成论文摘要