Enhancement of Fuzzy Possibilistic C-Means Algorithm using EM Algorithm (EMFPCM)
semanticscholar(2017)
摘要
The major difficulties that arise in several fields, comprising pattern recognition, machine learning and statistics, is clustering. The basic data clustering problem might be defined as finding out groups in data or grouping related objects together. A cluster is a group of objects which are similar to each other within a cluster and are dissimilar to the objects of other clusters. The similarity is typically calculated on the basis of distance between two objects or clusters. Two or more objects present inside a cluster and only if those objects are close to each other based on the distance between them. In order to provide better clustering approaches that fits for all applications and to improve the efficiency of data clustering, this paper proposes a effective clustering techniques called Enhancement of Fuzzy Possibilistic C-Means Algorithm using EM Algorithm (EMFPCM). Thus with the help of EMFPCM, noise is reduced, provides more accuracy and thus provides better result in predicting the user behavior. The algorithm was implemented and the experiment result proves that this method is very effective in predicting user behavior. This approach is suitable for applications in business, such as to design personalized web service. The performance of the proposed approaches is evaluated on the UCI machine repository datasets such as Iris, Wine, Lung Cancer and Lymphograma. The parameters used for the evaluation is Clustering accuracy, Mean Squared Error (MSE), Execution Time and Convergence behavior.
更多查看译文
AI 理解论文
溯源树
样例
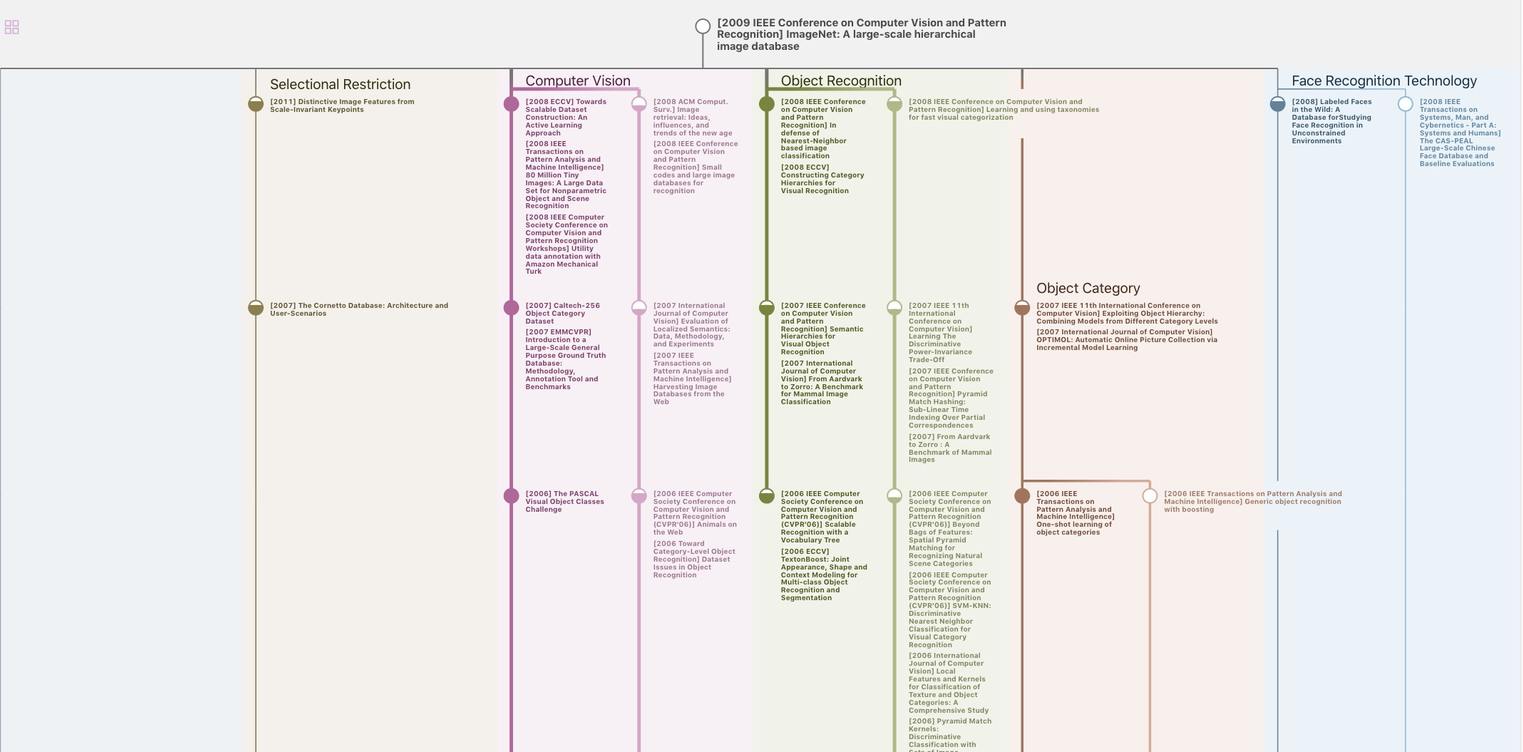
生成溯源树,研究论文发展脉络
Chat Paper
正在生成论文摘要