Adaptive Risk-Taking: Theory and Evidence from Developing Countries∗
semanticscholar(2020)
摘要
Developing countries exhibit high rates of macroeconomic volatility and low rates of individual risk-taking. In this paper we present evidence for a new channel linking these phenomena: lifetime experiences of macroeconomic volatility directly change individual risk attitudes. We combine two panel data sets from Indonesia and Mexico (n=15,000), containing elicited measures of risk aversion for the same subjects years apart, with state-level real GDP growth time series capturing their lifetime macroeconomic experiences. We find that living through periods of increasing volatility increases measured risk aversion. This effect is robust to controlling for changes in income, wealth, savings, and exposure to violence and natural disasters. Increases in measured risk aversion correlate with decreases in risky behaviors like smoking, migration, and the planting of cash crops. To better understand the mechanisms at play we develop a model of risk-taking adaptation over the life course. In our model a Bayesian expected utility maximizer who is exposed to exogenous background risk learns about the mean and variance of that risk from its realizations over their lifetime, in turn affecting their endogenous risky choice. Our model generates a novel testable prediction: large negative shocks will increase measured risk aversion more than commensurate positive shocks. We find significant evidence for this predicted asymmetry in our Indonesian sample. ∗Levin: rzlevin@ucsd.edu; Vidart: dvidartd@ucsd.edu. We are deeply grateful for the helpful comments and constant encouragement of many advisors and colleagues, including Prashant Bharadwaj, Pol Campos-Mercade, Mitch Downey, James Fowler, Simone Galperti, Alex Imas, Sam Krumholz, David Lagakos, Munseob Lee, Mark Machina, Tommaso Porzio, Valerie Ramey, Natalia Ramondo, Wayne Sandholtz, Joel Sobel, Isabel Trevino, Johannes Wieland, as well as participants at several conferences and numerous UCSD workshops. All mistakes are our own.
更多查看译文
AI 理解论文
溯源树
样例
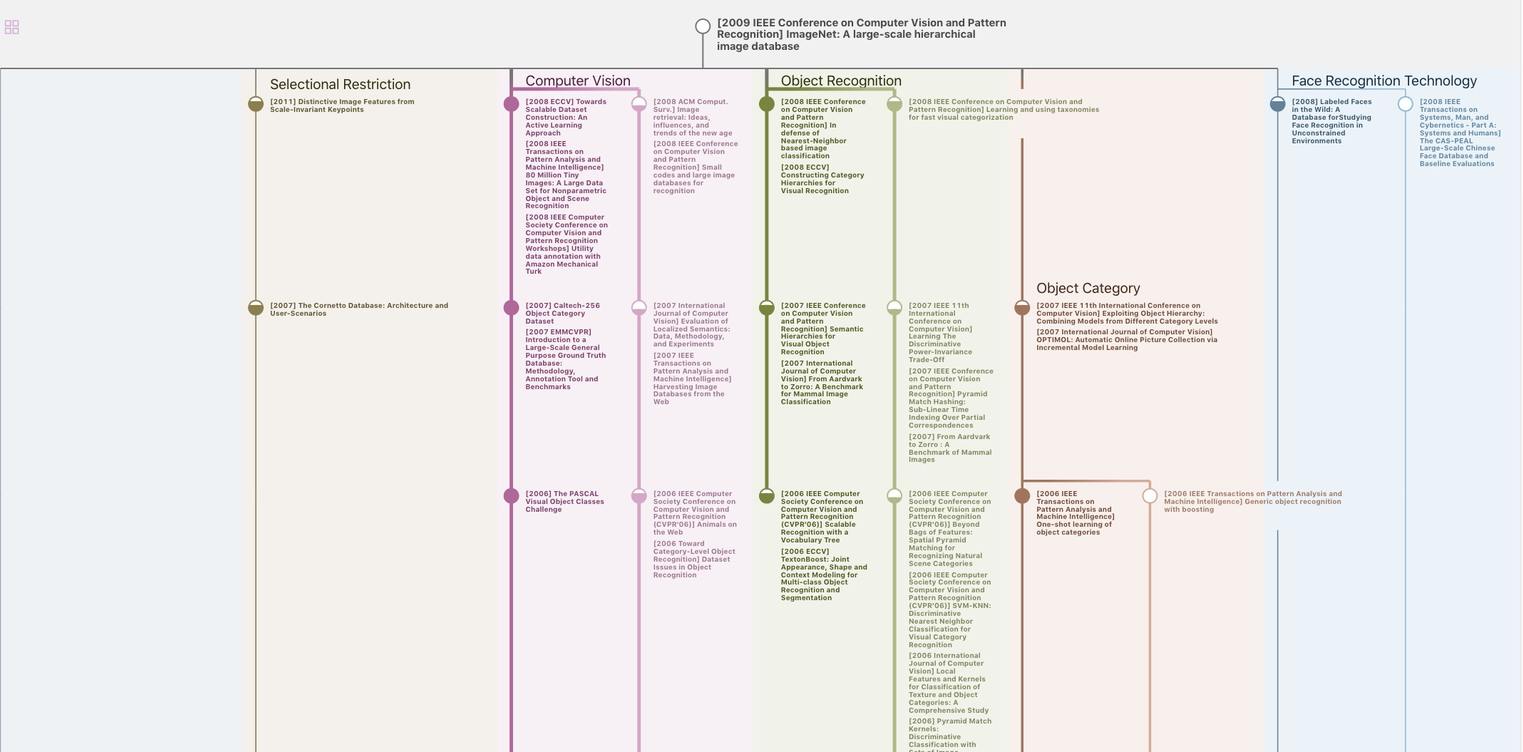
生成溯源树,研究论文发展脉络
Chat Paper
正在生成论文摘要