Towards Self-Optimizing Network : Applying Deep Learning to Network Tra ffi c Categorization and Identification in the Context of Application-Aware Network
semanticscholar(2018)
摘要
The application-aware routing is a network routing technology optimized for a network with an inconsistent link performance, a problem which is common for a multi-institution research and academic network. Using the application-aware routing, an application-aware network routes each flow independently via the optimal path corresponding to the identified application characteristic. This technology enables the creation of a self-optimizing network. However, an automatic network flow categorization and identification system is required. In the scope of this work, network flow categorization is defined as the process of generating a meaningful classification whereas network flow identification is defined as identifying which class a network flow belongs to. These are challenging problems with various applicabilities. We present a deep learning approach to network flow categorization and identification problems. Deep learning provides several advantages over existing solutions in the context of the application-aware network. According to our experiments, a 3-layer stacked denoising autoencoder trained with CAIDA Internet traffic dataset produces the most meaningful classification and a useful class identifier (classifier). This deep neural network (DNN) model generates three-classes classification: a bandwidth-bound pattern, a latency-bound pattern, and an irregular pattern. A design of a highly scalable implementation of a self-optimizing network using a DNN model is also presented with justification for each design decision. Our findings suggest that a deep learning approach to network flow categorization and identification problems in the context of the application-aware network and the self-optimizing network are promising.
更多查看译文
AI 理解论文
溯源树
样例
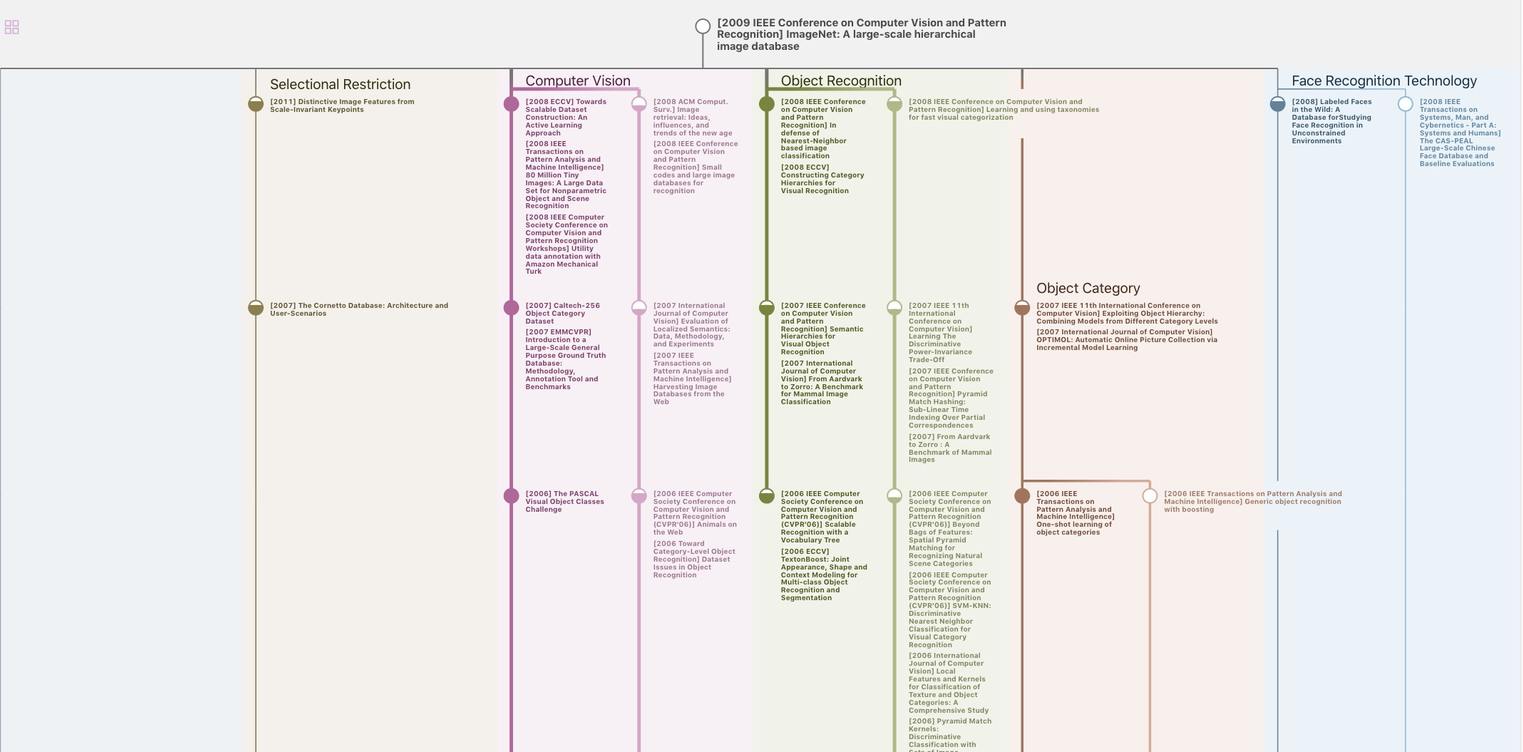
生成溯源树,研究论文发展脉络
Chat Paper
正在生成论文摘要