Applying and Adapting the Reformer as a Computationally Efficient Approach to the SQuAD 2.0 Question-Answering Task
semanticscholar(2020)
摘要
For the default project, we adapted the Reformer, an ostensibly more computationally efficient Transformer, for the SQuAD 2.0 task. This is an exciting task, as the Reformer has never been applied to question-answering in the literature. Along with the Reformer’s novelty (it was published in January 2020), we are interested in the use of locality-sensitive hashing the key idea behind the Reformer’s memorysaving benefits which greatly improves the speed of the attention computation. However, after testing two different models to see if the Reformer could outperform the baseline model BiDAF, we found significant flaws with the Reformer, most importantly how long it took to train. Although the Reformer is more memoryefficient, it takes significantly longer to train than a Transformer, which is itself quite slow to train. Ultimately, even per-epoch, the Reformer was not able to match the baseline model’s statistics. Our studies do not indicate that the Reformer is a strong model for the SQuAD 2.0 question-answering task. This project does not include any external collaborators and is not shared with any other class.
更多查看译文
AI 理解论文
溯源树
样例
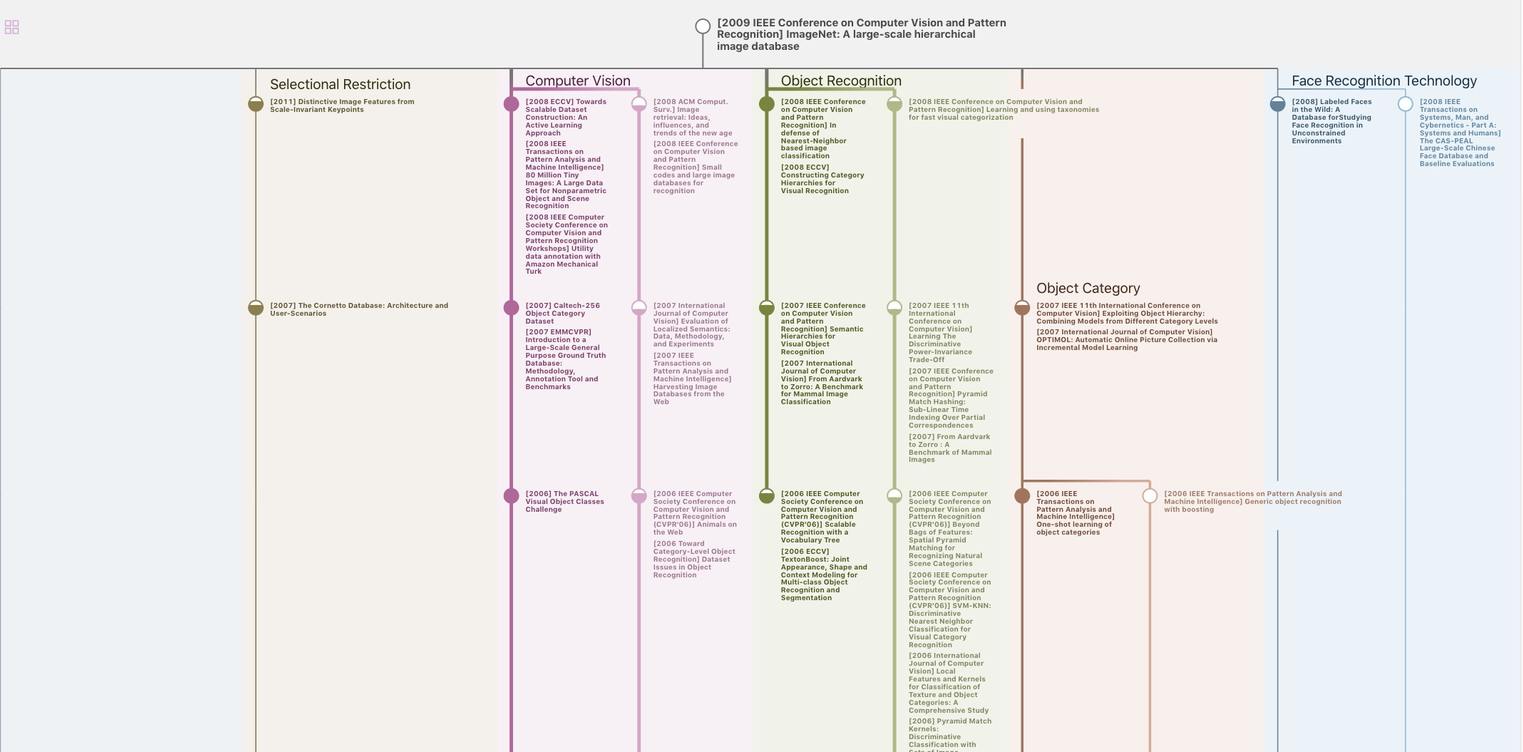
生成溯源树,研究论文发展脉络
Chat Paper
正在生成论文摘要