Biological Sequence Design using Batched Bayesian Optimization
semanticscholar(2019)
摘要
Being able to effectively design biological molecules like DNA and proteins to desired specifications would have a transformative effect on science. Currently, the most popular design method in biomolecular engineering is directed evolution [1, Nobel Prize 2018], which explores sequence space by making small mutations to existing sequences. Alternatively, Bayesian optimization (BO) is an attractive framework for model-based black-box optimization, and has recently been successful in molecular design [26, 19, 10, 9, 7, 14, 17]. However, most large-scale BO efforts within the ML community have focused on hyper-parameter tuning for ML; such methods often do not translate to biological sequence design, where the search space is over a discrete alphabet, wet-lab experiments are run with considerable parallelism (many sequences measured simultaneously), experiments are sufficiently time consuming and expensive that only few rounds of experiments are feasible, and we must account for the safety of patients that will be treated with the sequence. This paper discusses the particularities of batched BO within this unique context, and investigates the design choices required for robust and scalable design.
更多查看译文
关键词
Bayesian optimization,Machine learning,Computer science,Artificial intelligence
AI 理解论文
溯源树
样例
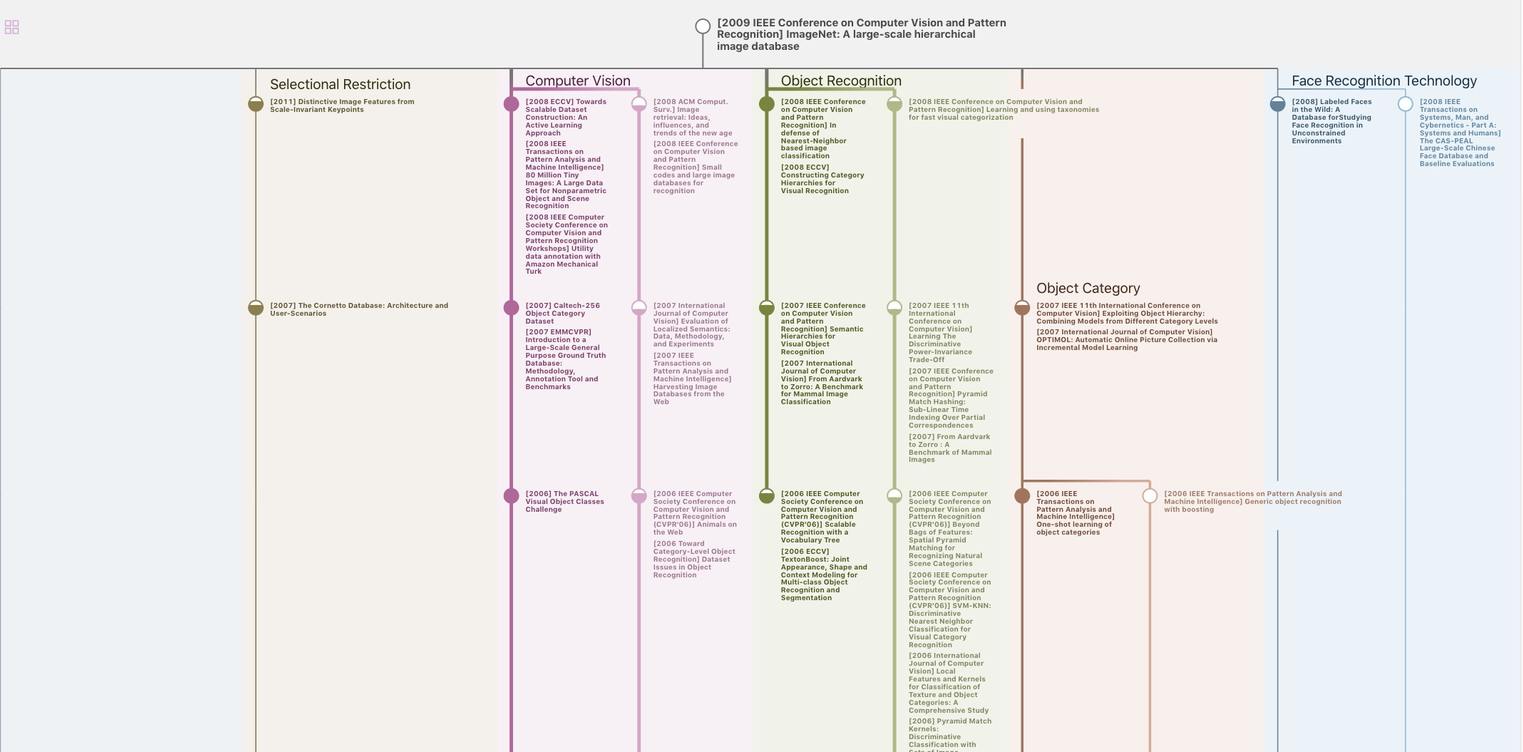
生成溯源树,研究论文发展脉络
Chat Paper
正在生成论文摘要