Highly Accurate Link Prediction in Networks Using Stacked Generalization
semanticscholar(2018)
摘要
Link prediction is an important task in complex networks which has a wide variety of applications in recommendation systems. Although many community detection methods can be used in this end, the recently proved No Free Lunch theorem for community detection implies that each makes some kind of trade-off, and no algorithm can be optimal on all inputs. Different algorithms will thus overor under-fit on different inputs, finding more, fewer, or just different communities than is optimal. Therefore, algorithms vary widely in how many communities they find in a given input, and these differences induce wide variation in accuracy on link prediction task. On the other hand, stacked generalization approach helps to design a high-level classification model via combining the lower-level models. The goal is to have better predictive performance by learning and fusing the best performance of each link prediction algorithm in different feature configurations. In this paper, we present a novel ensemble approach on link prediction using stacked generalization and show that via this approach we can achieve a higher precision and recall compared to each algorithm and to the best-overall-predictors. We present our results on 11 edge prediction algorithms on a novel and diverse corpus of 406 diverse real-world networks.
更多查看译文
AI 理解论文
溯源树
样例
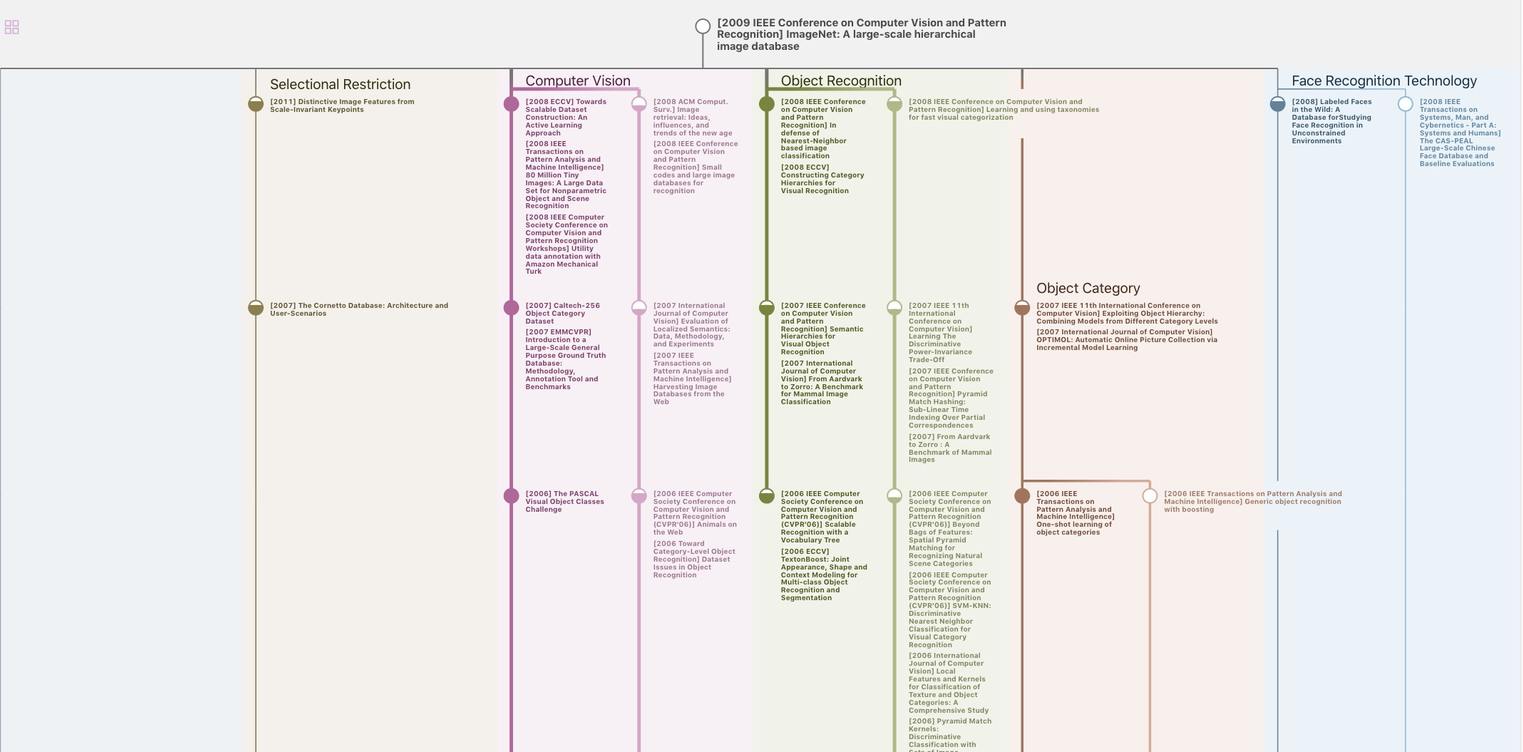
生成溯源树,研究论文发展脉络
Chat Paper
正在生成论文摘要