Entropy-Regularized Optimal Transport for Machine Learning. (Régularisation Entropique du Transport Optimal pour le Machine Learning)
semanticscholar(2019)
摘要
This thesis proposes theoretical and numerical contributions to use Entropy-regularized Optimal Transport (EOT) for machine learning. We introduce Sinkhorn Divergences (SD), a class of discrepancies between probability measures based on EOT which interpolates between two other well-known discrepancies: Optimal Transport (OT) and Maximum Mean Discrepancies (MMD). We develop an efficient numerical method to use SD for density fitting tasks, showing that a suitable choice of regularization can improve performance over existing methods. We derive a sample complexity theorem for SD which proves that choosing a large enough regularization parameter allows to break the curse of dimensionality from OT, and recover asymptotic rates similar to MMD. We propose and analyze stochastic optimization solvers for EOT, which yield online methods that can cope with arbitrary measures and are well suited to …
更多查看译文
AI 理解论文
溯源树
样例
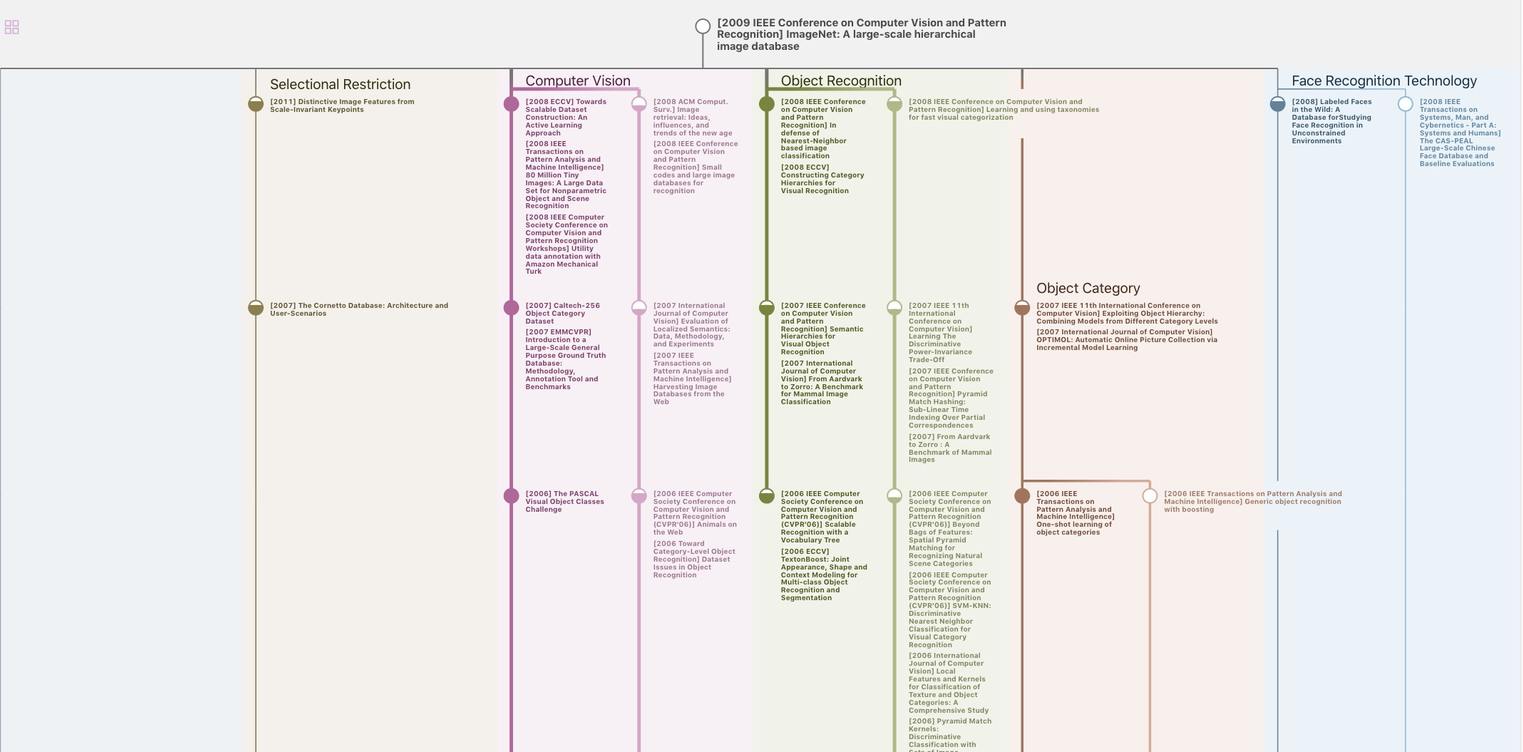
生成溯源树,研究论文发展脉络
Chat Paper
正在生成论文摘要