Task-Assisted Domain Adaptation With Anchor Tasks
2021 IEEE WINTER CONFERENCE ON APPLICATIONS OF COMPUTER VISION WACV 2021(2021)
摘要
Some tasks, such as surface normals or single-view depth estimation, require per-pixel ground truth that is difficult to obtain on real images but easy to obtain on synthetic. However, models learned on synthetic images often do not generalize well to real images due to the domain shift. Our key idea to improve domain adaptation is to introduce a separate anchor task (such as facial landmarks) whose annotations can be obtained at no cost or are already available on both synthetic and real datasets. To further leverage the implicit relationship between the anchor and main tasks, we apply our HEADFREEZE technique that learns the cross-task guidance on the source domain with the final network layers, and use it on the target domain. We evaluate our methods on surface normal estimation on two pairs of datasets (indoor scenes and faces) with two kinds of anchor tasks (semantic segmentation and facial landmarks). We show that blindly applying domain adaptation or training the auxiliary task on only one domain may hurt performance, while using anchor tasks on both domains is better behaved. Our HEADFREEZE technique outperforms competing approaches, reaching performance in facial images on par with a recently popular surface normal estimation method using shape from shading domain knowledge.
更多查看译文
关键词
task-assisted domain adaptation,anchor tasks,surface normals,single-view depth estimation,per-pixel ground truth,synthetic images,facial landmarks,HeadFreeze technique,cross-task guidance,auxiliary task,facial images,surface normal estimation method,semantic segmentation
AI 理解论文
溯源树
样例
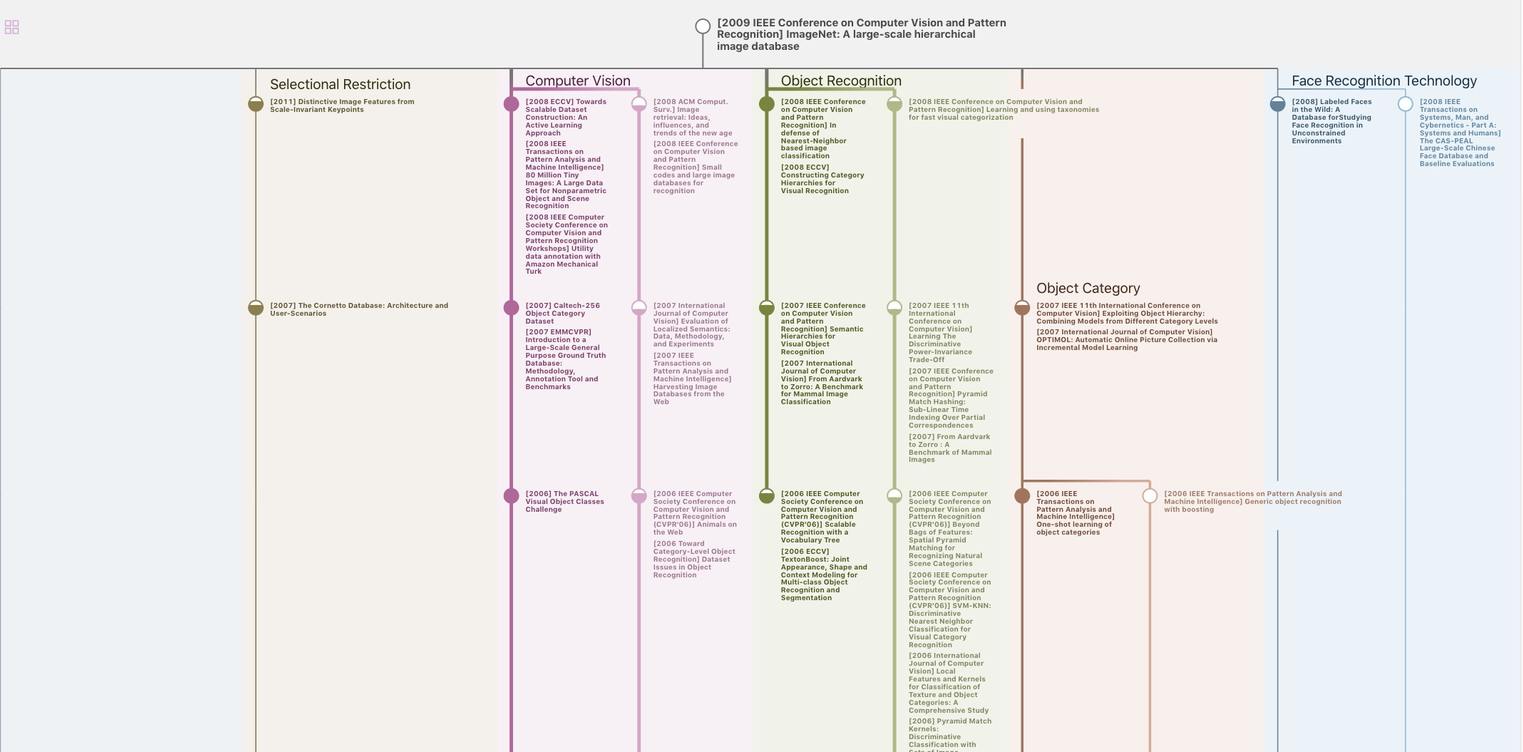
生成溯源树,研究论文发展脉络
Chat Paper
正在生成论文摘要